
- SUGGESTED TOPICS
- The Magazine
- Newsletters
- Managing Yourself
- Managing Teams
- Work-life Balance
- The Big Idea
- Data & Visuals
- Reading Lists
- Case Selections
- HBR Learning
- Topic Feeds
- Account Settings
- Email Preferences

When Blind Hiring Advances DEI — and When It Doesn’t

Three questions to ask before implementing the strategy into your hiring practices.
As a decision-making strategy, “blind hiring” involves blocking evaluators from receiving potentially biasing information about a job candidate until after an evaluation of their application materials are complete. Most famously, the tactic was used to boost the hiring of women in orchestras by having people audition from behind a screen that concealed their gender. But there’s a body of research that’s been conducted since that 2000 study showing that, while the strategy is generally effective, there are situations in which it might not help you diversify your candidate pool. The author outlines this research, and suggests three questions you should ask in order to get the most out of a blind hiring approach.
Inspired by the results of the famous orchestra study — where symphony orchestras began hiring more women by having people audition from behind a screen that concealed their gender — some major organizations are now using a “blind hiring” strategy to help achieve goals related to diversity. The typical blind hiring process involves stripping information from job application materials before review that could signal applicants’ memberships in specific groups and cue discrimination. Though not yet widespread, this de-biasing strategy is gaining traction: A recent survey of over 800 U.S.-based HR practitioners indicated that about 20% worked for organizations that used blind hiring and about 60% were familiar with it.

- SF Sean Fath is an Assistant Professor of Organizational Behavior at Cornell’s ILR School. His research interests include managerial decision making, bias reduction in social evaluations, and perceptions of social and organizational hierarchy.
Partner Center
- Search Menu
- Sign in through your institution
- Advance articles
- Author Guidelines
- Submission Site
- Open Access
- About European Sociological Review
- Editorial Board
- Advertising and Corporate Services
- Journals Career Network
- Self-Archiving Policy
- Dispatch Dates
- Journals on Oxford Academic
- Books on Oxford Academic

Article Contents
Introduction, conclusion and discussion, acknowledgements, appendix 1: occupations according to the required levels of education, sex ratios, and degree of decision-making: number of vacancies and applications (in parentheses) in each of the sampled occupations, appendix 2: samples of rresumes for a tax advisor*.
- < Previous
The Role of Gender Stereotypes in Hiring: A Field Experiment
These authors M. José González, Clara Cortina and Jorge Rodríguez have contributed equally to this article.
- Article contents
- Figures & tables
- Supplementary Data
M José González, Clara Cortina, Jorge Rodríguez, The Role of Gender Stereotypes in Hiring: A Field Experiment, European Sociological Review , Volume 35, Issue 2, April 2019, Pages 187–204, https://doi.org/10.1093/esr/jcy055
- Permissions Icon Permissions
Using correspondence testing, we investigate if employers discriminate against women based on stereotypes or prejudices. We sent four (two pairs of fictitious man–woman) résumés to 1,372 job offers from a broad selection of occupations. In one pair, candidates had equivalent curriculum vitae (CVs) except for their sex and their qualifications (meeting standards or higher). In the second pair, candidates differed by sex and parenthood status (with or without children). We interpret the observed differences in favour of men as signalling gender bias in recruitment. This bias is reduced when women have higher qualifications and increases when they have children. We interpret employers’ openness to modify their decisions when candidates’ personal characteristics differ from the group norm, and the absence of discrimination among highly qualified non-mothers, as evidence that gender bias in recruitment is largely grounded in employers’ stereotypes rather than in prejudices.
In this study, we use a correspondence testing approach to investigate whether employers discriminate against women based on stereotypes or prejudices. The correspondence test is an experimental technique that consists of sending pairs of résumés for job offers that are very similar in everything except the trait to be analysed—gender, in our case. Discrimination is established if candidates with the trait—females—have a lower probability of being selected for further screening. We sent two pairs of résumés to 1,372 real job advertisements appearing in two large local labour markets (Madrid and Barcelona) in Spain in 2016.
This research design allows us to address some issues insufficiently explored in the literature, namely the extent to which women experience subtle forms of discrimination based on the order in which they are selected for further screening. More importantly, unlike in many previous studies, we additionally estimate if gender discrimination varies by an applicant’s parenthood status and level of qualifications. This allows us to assess to what extent employers’ discriminatory practices are grounded in stereotypes about males and females’ typical qualifications and prescribed roles as mothers and workers, rather than on unswerving prejudice against, or aversion towards, female workers. Finally, and as our research strategy combines within- and between-job comparisons of candidates’ appeal to employers, we add important controls to the analyses pertaining to job characteristics, like the degree of masculinization of the occupation, the level of authority required in the job, and the tightness of the labour market within which the job offer is posted.
Do Employers Favour Men?
The gender discrimination hypothesis states that gender bias in recruitment, that is, employers’ conscious or unconscious preference for male candidates, is pervasive and it may arise for different reasons. According to economic theory, discrimination may be explained by employers’ imperfect information on applicants’ human capital characteristics, which are relevant for the job and also difficult to standardize on a résumé ( Becker, 1985 ; Heckman, 1998 ). Employers use group-level statistical summaries (i.e., group averages) as proxies for these variables, and this explains why individuals from these group are treated differently. Thus, in selecting candidates for a job opening, employers not only consider the observed and standardizable qualifications shown in candidates’ curriculum vitae (CVs), but also rely on stereotypes about the typical level and dispersion of other difficult-to-standardise qualifications. This practice leads to forms of statistical discrimination based on rational assessments of productivity and risk regarding potential employees ( Baumle and Fossett, 2005 ). Ascribed characteristics such as gender and age are used as potential proxies for traits that are difficult and expensive to measure in real contexts.
Some stereotypes are descriptive and based on knowledge of men and women’s typical abilities. This knowledge can be direct, based on experience, or indirect and transmitted by trusted third parties. Thus, in our societies, in which the division of labour is gendered, men typically appear as possessing greater agentic and leadership qualities than women, and higher aspirations and commitment at work. In contrast, women are assumed to possess greater communal qualities associated with caring behaviours ( Cuddy et al. , 2004 ). Other stereotypes are prescriptive, that is, based on cultural beliefs about what men and women ought or ought not do. They are often justified with reference to higher communal values that reinforce a system of patriarchal authority favouring men ( Connell, 1995 ; Rudman and Glick, 2001 ). Prescriptions are accompanied by sanctions when someone violates them. Thus, working mothers who behave agentically may be perceived as lacking femininity and be subjected to a variety of sanctions ( Connell, 1995 ; Benard and Correll, 2010 ).
Sociologists have repeatedly shown how the patriarchal character of organizational life reinforces the idea of separate spheres for women and men, and contributes to the perception that being an ‘ideal worker’ is incompatible with being a ‘good mother’ ( Fuegen et al. , 2004 ; Ridgeway and Correll, 2004 ; Benard and Correll, 2010 ; Glass and Fodor, 2011 ; Byron and Roscigno, 2014 ). The ideal worker is a ‘committed’ employee who sacrifices his most personal concerns, such as those derived from family responsibilities, for the sake of his career; a worker who is expected to drop all current engagements when a new and important work demand arises, devotes many hours to ‘face time’ at work when needed, or works late nights or weekends if necessary ( Correll et al. , 2007 ). The ideal of the ‘good mother’, instead, places the child at the centre of the family and assumes that child care is the chief responsibility of the mother—an emotionally absorbing, labour-intensive, and time-consuming responsibility ( Hays, 1996 ).
Descriptive stereotypes contribute to the generation of prescriptive views or beliefs about men and women’s proper roles in society; and prescriptions typically lead to the desired outcomes. Hence, under the ‘motherhood mandate’, women are expected to be more family oriented and less committed to paid work, and thus, less productive than similarly qualified male workers ( Russo, 1976 ; Hays, 1996 ). They are also expected to have higher rates of absenteeism, which, according to employers, eventually affects their productivity at work ( Correll et al. , 2007 ). Employers’ expectations may even be harmful to some employees, such as when pregnant women are determined to appear ‘well’ and remain at work even when feeling sick ( Gatrell, 2011 ).
According to the theories discussed above, both descriptive and prescriptive gender stereotypes may influence hiring processes. We develop two hypotheses to test these possibilities. We expect that discrimination against female candidates will be smaller for candidates with higher levels of qualifications for the job. The reduction in the level of employers’ discrimination against female candidates will indicate that they allow any perceived handicaps in women’s unobservables to be compensated with candidates’ higher observables. We will interpret a reduction in discrimination among applicants with higher standardized qualifications, as evidence that employers engage in statistical discrimination based on descriptive stereotypes or on shared beliefs about the typically different traits and abilities of men and women, which can be counteracted when candidates show higher qualifications.
Furthermore, we expect that discrimination against female candidates will be higher for candidates with children. As before, the change in employers’ level of discrimination against women will indicate that they are willing to adapt their stereotypes on women and men’s qualifications when receiving further information on candidates’ characteristics. However, the increase in discrimination will in this case show that their behaviour is based on prescriptions about the proper roles of men and women in society, punishing non-compliant women with higher discrimination when the stereotype of the good mother and worker is challenged. Hence, if discrimination increases when we restrict our sample to applications by job candidates with children, we will interpret this finding as evidence for statistical discrimination based on prescriptive stereotypes .
Instead of (or in addition to) statistically discriminating women, employers, particularly males, may rely on gender prejudices, based on negative feelings about women, that similarly result in higher barriers to women’s employment, especially in high-status occupations ( England, 1994 ; Jaret, 1995 ). Prejudices are negative judgements about groups that carry a stigma. These judgements are irrational because—unlike statistical discrimination—they are not based on expectations about groups’ productivities ( Becker, 1985 ). It can arise at different stages of an individual’s work history (pre-selection, job interview, or promotion), which makes it difficult to tackle the subject empirically ( Baumle and Fossett, 2005 ). Psychologists have studied in detail the basis of prejudicial attitudes—see Hodson and Dhont (2015) for a recent review of this work. They have shown that prejudicial individuals are more likely to display automatic emotional responses of animosity or antipathy towards members of other groups, based on faulty and inflexible generalizations of their inferior qualities ( Allport, 1954 ). Prejudices are often acquired at an early age and as a consequence, in the case of gender, of segregated socialization ( Glick and Hilt, 2000 ). We propose to test this hypothesis of prejudicial discrimination by assessing if discrimination is significant also in the group of female candidates with the lowest probability of being statistically discriminated—the group of highly qualified non-mothers. In other words, if discrimination remains for the group of most clearly competent female candidates, we will interpret this finding as evidence for discrimination based on prejudice .
Gender Discrimination in Recruitment Decisions
Much of the evidence on gender discrimination derives from field experiments such as correspondence studies. These studies are considered to be the most reliable methods to reveal unequal treatment in hiring ( Riach and Rich, 2002 ), because, unlike observational studies, they can control for selection effects and problems associated with endogeneity. These effects occur, for example, when women themselves make employment and occupational choices that lead them to disadvantaged positions ( Skyt Nielsen et al. , 2004 ; Sahni and Paul, 2010 ). While these choices may also respond to the same stereotypes and prejudices affecting employers, or anticipate these attitudes, they can generally only indirectly be attributed to discrimination ( Lundberg and Startz, 1983 ).
In correspondence studies, fictitious individuals who have nearly identical résumés except for certain traits such as sex, apply for the same jobs, and differences in outcomes—usually callback rates—are interpreted as reflecting discrimination. The difficulty in correspondence studies is generally not with detecting discrimination but with identifying its sources—stereotypes or prejudices (Neumark et al. , 1996). To accomplish the latter, in many correspondence studies the experimenter varies the personal characteristics of the fictitious applicants to determine if differences in employers’ rates of response vary accordingly between men and women ( Larribeau et al. , 2013 ). For example, the experimenter may vary the parenthood status of the applicant within or across jobs, and ascertain if women are more discriminated against when they have children ( Correll et al. , 2007 ; Albert et al. , 2011 ; Bygren et al. , 2017 ). If they are, this is attributed to employers’ reliance on prescriptive stereotypes when making hiring decisions, based on beliefs that mothers should not be given a job because their place is at home. In other studies, the marital or age status of the applicant is modified, and used as an indicator of how likely they are to become a parent in the near future ( Petit, 2007 ).
Still in other studies, qualities that are typically unobserved in a CV, like personality traits, are subtly added to candidates’ résumés, and employers’ reactions are identified and explored to reveal if women who display ‘masculine’ traits are penalized more than others ( Weichselbaumer, 2004 ). Finally, in some studies what is varied is the level of standardized qualifications of the applicant, in the expectation that employers relying on stereotypes may consider that women’s typical handicaps in unobservable traits are smaller (i.e., can be compensated) when they have higher standardizable qualifications—perhaps because only women who do not have these handicaps can achieve such higher qualifications (Larribeau et al. , 2013). In all cases, what allows distinguishing stereotypical from prejudicial discrimination is employers’ disposition to change their hiring decisions against women when applicants’ personal characteristics diverge from gender stereotypical norms. Prejudicial discrimination, in contrast, is residually established as any discrimination left and exercised against the most favoured sub-group of women.
The evidence provided by correspondence studies on gender discrimination in hiring, and on its sources, is rather mixed. A few studies conclude that there is no discrimination (e.g., Albert et al. , 2011 ; Bygren et al. , 2017 ). Some others maintain that discrimination occurs only for some subgroups of female applicants, as expected under the hypothesis of statistical discrimination based on stereotypes ( Petit, 2007 ). The remainder suggest that it occurs only in some contexts for some age groups ( Albert et al. , 2011 ). Correspondence studies differ also in terms of the contexts that they choose to study. Some are located in countries with strong family policies promoting and facilitating mothers’ employment, like Sweden or France ( Petit, 2007 ; Bygren et al. , 2017 ); others in more traditional institutional contexts like Spain ( Albert et al. , 2011 ; León and Pavolini, 2014 ); and still others, in societies with mixed gender equality records, like the United States or the United Kingdom ( Neumark et al. , 1996 ; Correll et al. , 2007 ; Larribeau et al. , 2013).
We sent sets of fake résumés to a wide-ranging sample of 1,372 job openings/vacancies between June and November 2016 in the two largest Spanish cities. Madrid and Barcelona are two of the most economically dynamic cities in the country, with a larger supply of job offers and employment rates far above the national mean, which makes them particularly suitable for our experimental research. Spain has recently experienced a rapid and massive incorporation of women into the labour market and the educational system, but it still exhibits large gender inequalities. According to the Spanish Statistical Office, in 2016 the employment rate for the population aged 25–54 (typical motherhood ages) was 65.6 per cent for women and 77.4 per cent for men, a difference of almost 12 points; the prevalence of unemployment in the same age group was higher for women than for men (20.3 per cent vs. 16.3 per cent); part-time work was overwhelmingly done by women (24.1 per cent vs. 7.8 per cent); women earned 14.2 per cent less than men when comparing their average gross hourly earnings, and they represented only a third (31 per cent) of workers in managerial positions (Eurostat). Gender inequalities in the labour market can be partly attributed to the limited support of the welfare state for working parents and mothers’ difficulties for reconciling paid work and caring responsibilities ( León and Pavolini, 2014 ). This explains why the presence of women in the labour market decreases with the number of children (according to Eurostat, in 2016 the employment rates for women aged 20–49 without children, with one or two children, and with three or more children were, respectively, 72, 67, and 52 per cent).
The sampling of jobs was designed to reflect the diversity of the labour markets in terms of the following: (i) the tightness or level of unemployment, which was proxied with the variable ‘city’ (the Spanish Statistical Office estimated the unemployment rates in Barcelona and Madrid in 2016 to be, respectively, 12, 5, and 14.8); (ii) the typical sex ratio of the occupations to which each job pertained, that is, male-dominated occupations (15–40 per cent women), mixed occupations not dominated by either sex (41–61 per cent women), or female-dominated occupations (62–83 per cent women); (iii), whether or not the tasks to be performed at work were managerial; and (iv) the average number of years of education of workers in the occupation, which we interpret as signalling the required level of qualifications for the job, that is, low, medium, or high. 1
However, it should be noted that the sample is far from being representative of all job openings in the two cities during the period of the experiment. First, the online Internet service we used to access the job openings, while widely used in both cities by job seekers, cannot capture other vacancies filled through more informal channels of recruitment ( Fernández-Muñoz and Blasco-Camacho, 2012 ). Second, by design, we decided to send applications to an approximately equal number of job openings in each of the 18 types of jobs (occupations) that resulted from multiplying the three levels of education by the three sex ratios by the two decision-making categories in which we divided all job openings—see Appendix 1 for a detailed list of the 18 occupations and the number of jobs applied for in each occupation (an extended version of the methodology, the data used in this study and the Stata do-file to completely replicate this study are available as Supplementary Data ).
In contrast to most previous correspondence studies, for each job opening we sent four fake applications. 2 The four applications consisted of two sets of matched CVs, with each set containing a CV from one male and one female with equivalent characteristics. The two sets differed in either candidates’ level of skills/qualifications for the job or their parenthood status (see the four sets of résumés in Appendix 2). As noted in the previous section, we were interested in assessing the effect of these two factors on employers’ gender discrimination practices. Thus, in approximately half of the job openings, we sent four applications consisting of two sets of matched-paired male–female applications differentiated by candidates’ skills. Skill differentiation was introduced in the CVs by making adjustments to the résumés. In half of the applications the candidate met the strict requirements for the job offer (i.e., a shop assistant had the typical educational level for this position, up to secondary education according to the labour force survey, and a short work experience). In the other half of the applications, the candidate also met the strict requirements for the job offer, up to secondary education for a shop assistant, but additionally he/she reported speaking a foreign language, having longer work experience and holding supervisory roles in previous jobs. In each of these four applications, the parenthood status of the candidates was fixed to either a ‘with two children’ or ‘without children’ status, with this status alternating across the job openings. In the other half of openings, we sent four applications consisting of two sets of matched-paired male–female applications, this time differentiated by candidates’ parenthood status, that is, by whether or not they had children. In these four applications, candidates’ skills were fixed to either low or high, with each skill level alternating across job openings.
By sending two pairs of applications differentiated by candidates’ skills to half of the job openings and two further pairs differentiated by parenthood status to the other half, we considerably reduced the sample of job openings needed to detect significant differences in discriminatory practices against women based on candidates’ qualifications and parenthood status. Additionally, this research design allowed us to test if variations in gender discriminatory practices according to candidates’ characteristics occur within the same job opening (e.g., when the same hiring agent discriminates against women when two candidates display low qualifications or when they have children, compared to when they do not) or across job openings (e.g., when two agents from two different companies display differential treatment towards women), or in both cases. 3
There is an ongoing debate about the pros and cons of sending match-paired applications to the same employer or single applications to multiple employers. We took this debate into consideration when choosing our research design and opted for the matched-pairs design. This design allows us to use the order in which the candidates were called back for an interview, thus helping to understand the multiple and subtle ways in which discrimination may be practised. The matched-pair approach is well established in the sociological literature—see, for instance, Quadlin (2018) or Bygren et al. (2017) . Unmatched designs based on random allocation of fictitious candidates across jobs guarantee that the treated and controlled arms are equivalent only in the expectation (on average across multiple replications of an experiment). A matched-pair design replicates the experiment on the same unit/employer, making the experiment more efficient and minimizing the risk of making Type II errors ( Bruhn and McKenzie, 2009 ).
Our decision to send four rather than two résumés to the same employer helped solve a problem often overlooked in matched-paired designs that send only two CVs. In the latter, the decision to decline a first invitation to attend a job interview issued to one of the fake candidates may increase the probability that the second fake candidate is selected, thus potentially obscuring the extent of discrimination. If employers’ hiring strategy consists of calling a fixed number of potential candidates for an interview, the probability of being on this list could be affected by other candidates’ (also fake candidates’) decisions to decline the invitation, giving an opportunity to a female candidate to be interviewed who otherwise might have never had this opportunity (especially in jobs with few applicants). By sending four résumés and by considering the order in which the candidates are selected, we avoid this problem and are better able to assess the extent of discrimination.
The age of all candidates varied randomly across job openings, but it was always within the range of 37 to 39 years. This age range was selected to reduce employers’ uncertainty about potential maternity or paternity leaves linked to future births. Each application used one of over 350 individual profiles created specifically for the study. The creation of such profiles and their online storage was required by the Internet service as a condition to apply for any of the job openings. To save resources, we decided to use the same profile for four different job openings. Each profile included a photograph randomly assigned to the corresponding fake male or female candidate and a résumé, standardized in the format required by the Internet service, without any cover letter. The standardized form of the résumé helped reduce any potential biases stemming from its layout.
Responses to the applications from interested employers, usually for the purpose of setting up an interview with the candidate, arrived in the form of either a call to a cell phone number or a message to an email account setup for groups of candidates never applying for the same opening. The fieldwork team used eight different cell phones to receive the phone calls and checked the email accounts of each of the fake candidates daily. Application rejections arrived in the form of messages sent explicitly by the web service or were inferred from the absence of any contact from the prospective employer 4 weeks after the application was submitted.
Our research design raises several ethical concerns as it imposes an additional burden on employers who review fictitious job applications and participate in an experiment without giving informed consent. Deception and absence of informed consent are serious issues which in our case required the approval of an Ethical Consortium before the field work could start. The Consortium decided that detecting and understanding how gender discrimination operates within the Spanish labour market outweighed these ethical concerns.
Analytical Strategy
To assess the presence of discrimination, we analyse differences in response rates by sex in multilevel models, in which applications (level 1) are nested within job vacancies (level 2). This model allows us to take into account the auto-correlation of decisions made by the same employer regarding the set of applications we sent to them for evaluation. Multilevel models are common in matched-pair designs because they are more efficient, and the standard errors they estimate are unbiased (see Uggen et al. , 2014 ). We estimate two types of models, according to whether the dependent variable is one of the following: (i) a binary variable measuring whether the candidate was or was not called back by the employer for further screening; or (ii) a limited interval variable reflecting the call order in which the candidates were called back, scaled from 0 (he or she was never called back), to 1 (he or she was called back in fifth place 4 ), to 2, 3, 4, and 5 (he or she was called back before any other candidate). For the first set of analyses (a), we use multilevel logit models; for the second set (b), we use multilevel ordered logit models, which are more appropriate than OLS regression to estimate models in which the interval dependent variable’s distribution is strongly skewed and bounded within a limited set of discrete, positive values ( Greene, 2012 ).
We use random intercepts because two characteristics used to distinguish the male–female matched pairs were forced to vary randomly. In half of the job openings, the two pairs differed in skill level and had the same parenthood status, which changed randomly for all four candidates across jobs. In the other half, they differed in parenthood status and had the same level of skills, which changed randomly across jobs. Hence, there were two possible sources of variation in skills and parenthood statuses: variations among fake candidates applying for the same job and variations among fake candidates applying for different jobs.
In all models, the independent variables of substantive interest are candidates’ sex, qualification level, and parenthood status. In all models, we test whether differences in callback probabilities between men and women differ for candidates with alternative skills and parenthood status via two-way interaction effects between gender and each of the other variables of interest. In all models, we also use two types of controls, linked to the characteristics of either the applications or the job openings. 5 For the former, the characteristics are: the order in which the application was sent within the set, and whether it was within a set of two, four, or six applications (and for the four-application sets, whether the trait that was fixed for all candidates was their level of skills or parenthood status). Among the latter controls, we included the number of other applicants that had applied for the job at the time we sent our first application, whether or not the job bestowed supervisory and decision-making power; the level of education required by the job; whether the three-digit occupational category to which the job belonged was male or female dominated, or mixed; and the city in which the job was offered.
Number of applications and callback probabilities by candidates’ sex, skill level, and parenthood status
. | . | Candidates’ skill levels and sex | . | |||||||
---|---|---|---|---|---|---|---|---|---|---|
Low | High | Total | ||||||||
Men . | Women . | Total . | Men . | Women . | Total . | Men . | Women . | Total . | ||
Candidates’ parentdood status | ||||||||||
Childless | 664 | 662 | 1,326 | 730 | 728 | 1,458 | 1,394 | 1,390 | 2,784 | |
0.093 | 0.048 | 0.071 | 0.136 | 0.124 | 0.130 | 0.115 | 0.088 | 0.102 | ||
With children | 649 | 649 | 1,298 | 769 | 769 | 1,538 | 1,418 | 1,418 | 2,836 | |
0.076 | 0.049 | 0.062 | 0.126 | 0.079 | 0.103 | 0.103 | 0.066 | 0.084 | ||
Total | 1,313 | 1,311 | 2,624 | 1,499 | 1,497 | 2,996 | 2,812 | 2,808 | 5,620 | |
0.085 | 0.049 | 0.067 | 0.131 | 0.101 | 0.116 | 0.109 | 0.077 | 0.093 |
. | . | Candidates’ skill levels and sex | . | |||||||
---|---|---|---|---|---|---|---|---|---|---|
Low | High | Total | ||||||||
Men . | Women . | Total . | Men . | Women . | Total . | Men . | Women . | Total . | ||
Candidates’ parentdood status | ||||||||||
Childless | 664 | 662 | 1,326 | 730 | 728 | 1,458 | 1,394 | 1,390 | 2,784 | |
0.093 | 0.048 | 0.071 | 0.136 | 0.124 | 0.130 | 0.115 | 0.088 | 0.102 | ||
With children | 649 | 649 | 1,298 | 769 | 769 | 1,538 | 1,418 | 1,418 | 2,836 | |
0.076 | 0.049 | 0.062 | 0.126 | 0.079 | 0.103 | 0.103 | 0.066 | 0.084 | ||
Total | 1,313 | 1,311 | 2,624 | 1,499 | 1,497 | 2,996 | 2,812 | 2,808 | 5,620 | |
0.085 | 0.049 | 0.067 | 0.131 | 0.101 | 0.116 | 0.109 | 0.077 | 0.093 |
The overall callback rate was 9.3 per cent 6 (see lowest right-hand corner of Table 1 ), which is within the standard of previous audit studies ( Bertrand and Mullainathan, 2004 ; Quadlin, 2018 ) despite the high unemployment rate of the country. As expected, candidates with higher qualifications on their résumés were called in larger numbers than candidates with lower qualifications (11.6 per cent vs. 6.7 per cent). Additionally, childless candidates received more callbacks than candidates with children (10.2 per cent vs. 8.4 per cent). More importantly for this study, men were called back in higher proportions than women (10.9 per cent vs. 7.7 per cent). Finally, Table 1 shows that even in the sub-group of women subject to lower discrimination (highly qualified non-mothers) men were called back in higher proportions than women (13.6 per cent vs. 12.4 per cent).
Multilevel logit of being called back for an interview, by candidates’ sex, level of qualification, and parenthood status, and controlling for key characteristics of the application and the job opening
. | Coefficient . | SE . |
---|---|---|
Female | −0.86 | 0.242 |
High skills | 0.57 | 0.200 |
Female # high skill | 0.43 | 0.267 |
With children | −0.28 | 0.186 |
Female # with children | −0.34 | 0.256 |
Masculinised occupation | −1.45 | 0.296 |
Feminized occupation | −1.34 | 0.328 |
Non-managerial | −1.15 | 0.270 |
Low education job | −0.71 | 0.300 |
High education job | −1.50 | 0.306 |
Barcelona | 1.26 | 0.255 |
No. of applications | −0.00 | 0.001 |
Order of application | 0.01 | 0.057 |
4 application sets (parenthood status fixed) | 0.76 | 1.225 |
4 application sets (skill level fixed) | 1.51 | 1.219 |
6 application sets | −0.56 | 1.293 |
Constant | −3.80 | 1.260 |
/lnsig2u | 2.03 | 0.133 |
Sigma_u | 2.76 | 0.183 |
Rho | 0.70 | 0.028 |
. | Coefficient . | SE . |
---|---|---|
Female | −0.86 | 0.242 |
High skills | 0.57 | 0.200 |
Female # high skill | 0.43 | 0.267 |
With children | −0.28 | 0.186 |
Female # with children | −0.34 | 0.256 |
Masculinised occupation | −1.45 | 0.296 |
Feminized occupation | −1.34 | 0.328 |
Non-managerial | −1.15 | 0.270 |
Low education job | −0.71 | 0.300 |
High education job | −1.50 | 0.306 |
Barcelona | 1.26 | 0.255 |
No. of applications | −0.00 | 0.001 |
Order of application | 0.01 | 0.057 |
4 application sets (parenthood status fixed) | 0.76 | 1.225 |
4 application sets (skill level fixed) | 1.51 | 1.219 |
6 application sets | −0.56 | 1.293 |
Constant | −3.80 | 1.260 |
/lnsig2u | 2.03 | 0.133 |
Sigma_u | 2.76 | 0.183 |
Rho | 0.70 | 0.028 |
Notes: Baseline: male, low skilled, childless, mixed occupation, managerial, mid-education job, Madrid, 0 previous applicants, sent first, only two applications sent.
Significant at the 0.01 level.
Directional test.
Focussing on the variables of substantive interest, the results in Table 2 suggest that women are significantly more discriminated against by employers than men. These gender discriminatory practices are not significantly different among candidates with lower and higher qualifications or among candidates with and without children. 7 However, the direction of the differences is as expected in our two hypotheses concerning statistical discrimination—higher gender discrimination among less qualified applicants and among candidates with children.
Multilevel ordered logit of being called back for an Interview, by candidates’ sex, level of qualification, and parenthood status, and controlling for key characteristics of the application and the job opening
. | Coefficient . | SE . |
---|---|---|
Female | −0.57 | 0.229 |
High skills | 0.28 | 0.185 |
Female # high skill | 0.47 | 0.251 |
With children | 0.21 | 0.178 |
Female # with children | −0.56 | 0.245 |
Masculinised occupation | −1.19 | 0.241 |
Feminized occupation | −2.33 | 0.329 |
Non-managerial | −0.71 | 0.225 |
Low education job | −0.08 | 0.257 |
High education job | −0.94 | 0.261 |
Barcelona | 1.19 | 0.219 |
No. of applications | −0.00 | 0.001 |
Order of application | 0.53 | 0.998 |
4 application sets (parenthood status fixed) | 0.75 | 0.989 |
4 application sets (skill level fixed) | −0.75 | 1.053 |
6 application sets | 0.04 | 0.054 |
Cutpoint 1 | 3.68 | 1.036 |
Cutpoint 2 | 3.69 | 1.036 |
Cutpoint 3 | 3.80 | 1.037 |
Cutpoint 4 | 4.03 | 1.038 |
Cutpoint 5 | 4.60 | 1.040 |
Variance constant | 4.44 | 0.663 |
. | Coefficient . | SE . |
---|---|---|
Female | −0.57 | 0.229 |
High skills | 0.28 | 0.185 |
Female # high skill | 0.47 | 0.251 |
With children | 0.21 | 0.178 |
Female # with children | −0.56 | 0.245 |
Masculinised occupation | −1.19 | 0.241 |
Feminized occupation | −2.33 | 0.329 |
Non-managerial | −0.71 | 0.225 |
Low education job | −0.08 | 0.257 |
High education job | −0.94 | 0.261 |
Barcelona | 1.19 | 0.219 |
No. of applications | −0.00 | 0.001 |
Order of application | 0.53 | 0.998 |
4 application sets (parenthood status fixed) | 0.75 | 0.989 |
4 application sets (skill level fixed) | −0.75 | 1.053 |
6 application sets | 0.04 | 0.054 |
Cutpoint 1 | 3.68 | 1.036 |
Cutpoint 2 | 3.69 | 1.036 |
Cutpoint 3 | 3.80 | 1.037 |
Cutpoint 4 | 4.03 | 1.038 |
Cutpoint 5 | 4.60 | 1.040 |
Variance constant | 4.44 | 0.663 |
Significant at the 0.05 level.
The cut points at the bottom of the table indicate the thresholds on the latent variable governing the probability across all jobs that a candidate would be chosen with a different priority or not at all. Cut point 1 is the estimated threshold on this latent variable used to distinguish candidates chosen in the last (fifth) place compared to candidates who were not selected for an interview (whose cut point is set to 0). Cut point 2 is the estimated threshold on the latent variable used to distinguish candidates chosen in fourth place from those chosen in fifth place; and so on until cut point 5, which is the estimated threshold that separates candidates chosen in first place from candidates chosen in second place.
The values of the cut points give a sense of the distance (in terms of probability) of falling into each category of the dependent variable. The ‘distance’ between the score 0 assigned to the baseline and cut point 1 is much larger (3.683) than the distance between cut points 1 and 2 (3.691–3.683 = 0.008). The scaling of the cut points in the dependent variable allows the coefficients in Table 3 to be interpreted as per a regular multilevel model with an interval dependent variable, as the change in the mean of the dependent variable per unit change in each independent variable (for continuous independent variables), or as the difference in the predicted mean of the dependent variable between any category of interest and the baseline category represented by candidates who were never called back (for categorical independent variables).
The effects of the controls on the mean of the rescaled variable trend in the same direction as those estimated previously for the logit model. Application characteristics have no impact on whether or not candidates are selected for an interview and the order in which it occurs. In terms of job characteristics, those that typically have a mixed male–female composition, that do not require much decision-making, that require medium levels of education, that were offered in Barcelona, and that received lower numbers of applications from non-fake candidates, all display higher scores in the optimally rescaled dependent variable, meaning that these candidates were given priority over other candidates by employers in the selection process.
The coefficients for the variables of substantive interest also trend in the same direction as in the multilevel logit model. The main difference is that the interaction effects between candidates’ gender and skills and between candidates’ gender and parenthood status are now significant (directional tests). The results show that applications by women are given significantly less priority by employers than applications by men, thus confirming the hypothesis on gender discrimination . This penalty is reduced if they have higher observable qualifications (see the positive coefficient for the interaction between sex and skills), thus confirming the hypothesis on discrimination based on descriptive stereotypes —that women’s disadvantage can be at least partially offset with higher standardizable qualifications, possibly because these qualifications compensate for any deficits in unstandardizable skills unobservable to us, the experimenters, which employers associate with female candidates. The results also show that women’s disadvantage increases significantly when they are mothers (see the negative coefficient for the interaction effect between sex and having children), thus confirming the hypothesis on discrimination based on prescriptive stereotypes —that women face a double disadvantage as females and mothers, possibly because employers expect their productivity at work to be negatively affected by their family commitments. Finally, the results show that contrary to what was posited in the hypothesis on prejudicial discrimination , discrimination against women is not significant for the group of women with the highest probability of being called back for further screening—highly qualified non-mothers. This can be more easily appreciated when an equivalent model to the one displayed in Table 3 is estimated in which the reference category for the candidates’ skills variable is switched to ‘high skills’. In this model, the coefficient for female’s main effect is negative (−0.10) but non-significant ( P = 0.305 in a directional test).
Figure 1 displays the probabilities for male and female candidates with low and high levels of qualifications and with and without children, predicted by the multilevel ordered logit in Table 2 .

Predicted cumulative probabilities of being selected for an interview in different orders, by candidates’ productivity marks and gender
The discrimination faced by females relative to males crystallises into an overall significant reduction of approximately 35 per cent in the probability of being called back for an interview 8 This penalty varies significantly according to candidates’ qualification levels and parenthood status. It is 23.4 per cent lower if women have higher skills, but increases to 46.6 per cent if they have lower levels of qualifications than similar men. It declines to a 20.3 per cent lower callback probability if they do not have children, but it rises to a 46.7 per cent lower probability if they do. Even in the most favourable case (highly qualified non-mothers), women experience a penalty of 6.7 per cent compared to their male counterparts, but it is statistically insignificant.
By fitting a multilevel multinomial logit model and comparing its results with those yielded by the multilevel ordered logit, we tested the parallel regression assumption that, on average, the estimated coefficients in this latter model are constant across all categories of the dependent variable. We could not reject this assumption. 9 However, we found some differences between specific coefficients that helped refine our previous findings. 10 Thus, we found that the weakening of gender discrimination among highly qualified candidates occurs significantly only if candidates are not called in first or second position. 11 The higher probability that more qualified candidates have of being selected applies to men when they are high on the list of employers’ prospective candidates, while for women it applies only when they are in lower positions. This finding suggests that the mechanism by which women may overcome any perceived deficits in productivity with higher observable qualifications, operates only when employers run out of candidates. In contrast, the penalty for mothers applies mainly to women’s chances of being given priority consideration (of being called in first or second position). 12
We have tested whether employers discriminate against women in the labour market in two major Spanish cities (Madrid and Barcelona). To assess if such discriminatory practices do indeed occur, we carried out a correspondence study in which we sent fake and equivalent résumés from non-existent male and female candidates to a large and heterogeneous sample of job openings advertised via the main Internet service operating in both cities. We then waited to see if employers reacted more favourably to men’s applications than to women’s applications.
In contrast to most existing studies into gender discrimination, we sent more than one set of matched-pair male–female applications to each job opening. The sets were differentiated by candidates’ skill levels—sufficient to meet the requirements of the job or clearly in excess of these requirements—and by their parenthood status—with or without children. This design allowed us to test some important hypotheses about the extent to which employers base their discriminatory practices on an aversion to women, possibly rooted in segregated forms of gender socialization during childhood, or on the use of informational and evaluative shortcuts based on gender stereotypes about female and male applicants’ different qualifications and proper roles in society. If employers adapted their decisions to applicants’ personal characteristics, taking into account how much these deviate from cognitive expectations and social prescriptions, they would be committing statistical discrimination grounded on stereotypes. If they adopted the same discriminatory behaviour regardless of candidates’ idiosyncrasies, their behaviour could be more easily attributed to an invariant emotional response, and to prejudicial discrimination.
The different variations we constructed on candidates’ CVs during the experiment—variations in skills which met or exceeded the posting or variations in parenthood status (with or without children)—further allowed us to test two hypotheses about the extent to which stereotypical discrimination was based on descriptive or prescriptive stereotypes. The results of our analyses supported the hypothesis about the overall presence of gender discrimination and the two hypotheses about statistical discrimination based on descriptive and prescriptive stereotypes, but we could detect the latter only when we used a refined version of our dependent variable that considered the order in which employers called the candidates back for an interview. In contrast, we could not detect a significant presence of prejudicial discrimination against the group of women with the highest probability of being selected for further screening.
The paper makes both empirical and methodological contributions to the field of gender discrimination in the labour market. Empirically, we were able to demonstrate that employers do discriminate against women in their hiring processes. More importantly, in line with previous research (Neumark et al. , 1996; Baumle and Fossett, 2005 ; Correll et al. , 2007 ), we have provided strong evidence that this discrimination has a ‘statistical’ basis, as it is grounded in employers’ stereotypes about the potentially lower productivity of female applicants. We have shown that these deficits are associated both with potential gaps in abilities that are difficult to include in a standard résumé and with mothers’ and fathers’ prescriptive roles as, respectively, committed housekeepers and workers.
We have also contributed to improving the experimental approach applied to correspondence studies by sending two, rather than just one, set of matched-pair, male–female applications to the same job opening. The sets distinguished pairs of candidates by the factors we were interested in examining in terms of their effects on gender discriminatory practices—candidates’ qualifications and parenthood status. By alternating the factors by which pairs of candidates were distinguished, we could save resources and significantly reduce the requisite sample size for the experiment. Additionally, this design allowed us to consider potentially undesirable experimental effects in matched-pair correspondence studies linked to the decision to refuse employers’ invitations to attend a job interview when these invitations occur. In a typical correspondence study, in which two applications are sent to the same job opening and there are few other credible applicants, a refusal by, for example, a fake male applicant to continue in the selection process might increase the probability of approaching the fake female applicant, thus obscuring the extent of discrimination against women. Such experimental effects are less likely to occur when there are several fake male and female candidates and can be captured by considering the order in which successful candidates are called for further screening.
This study is not without limitations. First, as previous research on the sources of discrimination has noted ( Heckman, 1998 ; Neumark, 2012 ), stereotypical discrimination may be based, not only on expectations or prescriptions about men and women’s average qualifications, but also on the dispersion of such qualifications in the two sexes, resulting in higher or lower uncertainties about candidates’ future productivities. A second limitation is that we cannot confirm if mothers’ double disadvantage as women and parents is the result of employers’ prescriptive views about their ‘natural’ nurturing roles, as we interpreted it here, or of their expectations about mothers’ lower commitment to work. That parenthood status is not associated with lower effort is demonstrated by our finding that fathers have a higher probability of being selected for further screening than non-fathers. Hence, we think it reasonable to conclude, in line with previous studies ( Benard and Correll, 2010 ), that any expectations about mothers’ lower commitments to work are based on prescriptive views about their proper place at home. In any case, both interpretations are complementary rather than alternative.
Finally, more work is needed to assess the characteristics of jobs that foster or mitigate discrimination against women vis-à-vis employers’ hiring practices. We controlled for some of the key job characteristics that the literature has previously argued could affect gender discriminatory practices. Our sampling strategy was intended to explore discrimination across a wide range of contexts and occupations, giving the same weight to each. This helped us obtain analytical estimates of discrimination and its forms that are insensitive to the specific distribution of jobs in the labour markets analysed. This was only partially accomplished, since we could not find as many jobs as planned that required significant decision-making. Very probably, this had the effect of underestimating gender discrimination, as this has been shown to be lower in jobs that require fewer agentic qualities from workers. More research is necessary to assess if the characteristics and requirements of the jobs and labour markets where they are placed exert a moderating or exacerbating influence on gender discrimination. This line of inquiry, too, shall be the focus of our future work.
M. José González is an Associate Professor in the Department of Political and Social Science at Pompeu Fabra University in Barcelona (Spain). Her research interests include family formation, child care, fatherhood, domestic work, and gender inequalities. Her recent work has appeared in European Journal of Women’s Studies , European Societies , and Population Research and Policy Review .
Clara Cortina is a Lecturer in the Department of Political and Social Science at Pompeu Fabra University in Barcelona (Spain). Her research interests include changes in partnership and family dynamics in contemporary Europe. Her recent work has appeared in Demographic Research , Review of Economics of the Household , European Journal of Population , and Population and Development Review .
Jorge Rodríguez is an Associate Professor Serra Hunter in Sociology and Criminology at the Department of Political and Social Sciences in Pompeu Fabra University in Barcelona (Spain). His research interests include occupational change, social mobility, status attainment, and social capital from a cross-national perspective, criminology and its studies of violence against women. His recent work has appeared Social Science Research , European Political Science Review , European Sociological Review , and Sociological Methods and Research .
We used micro-data from the Spanish Labour Force Survey (second quarter) to estimate the main characteristics of occupations (sex ratios and required levels of education). We classified occupations according to three broad categories: low level, with a mean of primary education (delivery men/women, waiting persons, sales clerks, foremen/women, head chefs, and store managers); medium level with a mean of secondary education (computer technicians, estate agents, office clerks, heads of logistics, warehouse managers, and supervising clerks); and high level with a mean of university degree (industrial engineers, tax advisors, physiotherapists, marketing directors, senior lawyers, and senior nurses). The degree of decision-making was derived from the wording of the job offer. Managerial jobs required some of the following characteristics: decision-making (made explicit in the advertisement), assuming responsibilities, supervising tasks, making decisions on objectives but not on procedures, experience more important than education, normally entail high earnings. Non-managerial jobs did not explicitly mention decision-making, entailed low responsibility, no supervising tasks, decisions on procedures but not objectives, and the job offers valued more education than experience and entailed low earnings. We validated the design of the correspondence study with a pilot that took place between mid-June and mid-July in 2016 for six occupations. The purpose of the pilot was to test whether main treatments (i.e., skills and parenthood status) were clearly identified by employers. We also conducted interviews with HR managers to understand how they viewed and identified main characteristics in online résumés.
In 13 of the job openings applied for, the employer closed the selection process before we could send all four applications. In all cases, we were able to send at least one pair of matched male–female applications and observe employers’ reactions. Sending a set of four applications for each posting at one time would give rise to suspicion of fake CVs. We decided to send job applications for each post randomly with a time interval of 45 minutes between each. We sent six applications for a subsample of job openings (about 8.4 per cent of the total number of applications) in the pilot study to assess if the between-jobs comparisons yielded the same results as the within-jobs comparisons. In the analyses reported, we control for the number of applications sent to each job.
In our design, we also explored the possibility that the differential treatment shown by the same employer towards women with one trait of interest (e.g., low skills) could vary according to the other trait of interest (e.g., if they had children) by sending six applications to a small subset of job openings (79 job openings, or about 8.5 per cent of all vacancies applied to). These subsets contained three pairs of matched male–female applications. Two pairs differed in one of the characteristics of interest (e.g., low vs. high level of qualifications), while they had the same trait corresponding to the second differentiation (e.g., they all had children). The third pair had the opposite trait in this second differentiation (e.g., they did not have children) and either one of the two traits of the first differentiation (e.g., they had low qualifications). The characteristics of the pairs were alternated across job openings to have all possible combinations and comparisons. In the analyses, we controlled for the number of applications sent to each job opening: two (when the employers closed the application process before we could send all four applications), four, or six. In the most typical case in which we sent four applications, we controlled for whether the trait that was fixed in all four applications was candidates’ qualification levels or parenthood status.
See note 3 above. While in principle a candidate could be called back in sixth place, we did not observe any instance in which this possibility materialized.
The main results hardly change with or without controls.
It is possible that this rate may be underestimated, as some of the applications appeared in the online service as not having been read by the employers, and we could not ascertain whether this was indeed the case or if it simply reflected that the service had not updated the status of these applications.
This conclusion also holds for the differences in the partial or marginal effects of sex across skill levels and parenthood status that can be calculated from Table 2, which are available upon request.
We report the cumulative probabilities of being selected in all possible orders for an interview against the probability of not being selected at all.
χ 2 = 24.02, P = 0.089. The Brant test is not implementable in the software we used to estimate the multilevel ordered logit. Hence, we fitted a generalized structural equation model with two levels of analysis (jobs and candidates) and with the multinomial logit as the link function. Because of low callback counts at higher orders of selection, we recoded the dependent variable into only three levels: never called; called in third, fourth, fifth, or sixth place; and called in first or second place. The χ 2 value reported above corresponds to a Wald test for the linear equality of all coefficients.
Full details of these results are available upon request.
The interaction effect for women and skills is −0.83 (standard error = 0.36) for the contrast between being called in a third, fourth, fifth, or sixth place or never but only −0.42 (standard error = 0.35) for the contrast between being called first or second or never.
The interaction effect for being a woman and having children is −0.47 (standard error = 0.35) for the contrast between being called in the last place and −0.77 (standard error = 0.33) for the contrast between being called with high priority and never.
The authors thank Julia Rubio, Juan Ramon Jiménez, Guillem Subirachs for their contribution to the field work. The authors also thank the Barcelona MAR Health Park Consortium for reviewing the ethical aspects of this research, and the four anonymous referees for their constructive comments on an earlier version of this article.
This research has been supported by Recercaixa2014.
Albert R. , Escot L. , Fernández-Cornejo J. A. ( 2011 ). A field experiment to study sex and age discrimination in the madrid labour market . The International Journal of Human Resource Management , 22 , 351 – 375 .
Google Scholar
Allport G. W. ( 1954 ). The Nature of Prejudice . Cambridge, MA : Addison-Wesley .
Google Preview
Baumle A. K. , Fossett M. ( 2005 ). Statistical discrimination in employment: its practice, conceptualization, and implications for public policy . American Behavioral Scientist , 48 , 1250 – 1274 .
Becker G. S. ( 1985 ). Human capital, effort, and the sexual division of labor . Journal of Labor Economics , 3 , S33 – S58 .
Benard S. , Correll S. J. ( 2010 ). Normative discrimination and the motherhood penalty . Gender & Society , 24 , 616 – 646 .
Bertrand M. , Mullainathan S. ( 2004 ). Are Emily and Greg more employable Than Lakisha and Jamal? A field experiment on labor market discrimination . American Economic Review , 94 , 991 – 1013 .
Bruhn M. , McKenzie D. ( 2009 ). In pursuit of balance: randomization in practice in development field experiments . American Economic Journal: Applied Economics , 1 , 200 – 232 .
Bygren M. , Erlandsson A. , Gähler M. ( 2017 ). Do employers prefer fathers? Evidence from a field experiment testing the gender by parenthood interaction effect on callbacks to job applications . European Sociological Review , 33 , 337 – 348 .
Byron R. A. , Roscigno V. J. ( 2014 ). Relational power, legitimation, and pregnancy discrimination . Gender & Society , 28 , 435 – 462 .
Connell R. W. ( 1995 ). Masculinities . Berkeley : University of California Press .
Correll S. J. , Benard S. , Paik I. ( 2007 ). Getting a job: is there a motherhood penalty? American Journal of Sociology , 112 , 1297 – 1339 .
Cuddy A. J. C. , Fiske S. T. , Glick P. ( 2004 ). When professionals become mothers, warmth doesn’t cut the ice . Journal of Social Issues , 60 , 701 – 718 .
England P. ( 1994 ). Neoclassical economists’ theories of discrimination. In Burstein P. (Ed.), Equal Employment Opportunity: Labor Market Discrimination and Public Policy . NewYork : Walter de Gruyter , pp. 59 – 70 .
Fernández-Muñoz Á. , Blasco-Camacho M. ( 2012 ). Estrategias de Búsqueda de Empleo . Madrid : Centro de Estudios Financieros .
Fuegen K. , Biernat M. , Haines E. , Deaux K. ( 2004 ). Mothers and fathers in the workplace: how gender and parental status influence judgments of job-related competence . Journal of Social Issues , 60 , 737 – 754 .
Gatrell C. J. ( 2011 ). ‘I’m a bad mum’: pregnant presenteeism and poor health at work . Social Science & Medicine , 72 , 478 – 485 .
Glass C. , Fodor É. ( 2011 ). Public maternalism goes to market: recruitment, hiring, and promotion in postsocialist Hungary . Gender & Society , 25 , 5 – 26 .
Glick P. , Hilt L. ( 2000 ). Combative children to ambivalent adults: the development of gender prejudice. In Eckes T. , Trautner H. M. (Eds.), The Developmental Social Psychology of Gender . Mahwah, NJ : Lawrence Erlbaum Associates Publishers , pp. 243 – 272 .
Greene W. H. ( 2012 ). Econometric Analysis . 7th Edition, Upper Saddle River : Prentice Hall .
Hays S. ( 1996 ). The Cultural Contradictions of Motherhood . New Haven : Yale University Press .
Heckman J. J. ( 1998 ). Detecting discrimination . Journal of Economic Perspectives , 12 , 101 – 116 .
Hodson G. , Dhont K. ( 2015 ). The person-based nature of prejudice: individual difference predictors of intergroup negativity . European Review of Social Psychology , 26 , 1 – 42 .
Jaret C. ( 1995 ). Contemporary Racial and Ethnic Relations . New York : Harper Collins College Publishers .
Larribeau S. , Masclet D. , Peterle E. ( 2013 ). Une mesure expérimentale de la discrimination homme/femme à l’embauche . Revue D’économie Politique , 123 , 333 – 351 .
León M. , Pavolini E. ( 2014 ). ‘Social investment’ or back to ‘familism’: the impact of the economic crisis on family and care policies in Italy and Spain . South European Society & Politics , 19 , 353 – 369 .
Lundberg S. , Startz R. ( 1983 ). Private discrimination and social intervention in competitive labor markets . American Economic Review , 73 , 340 – 347 .
Neumark D. ( 2012 ). Detecting discrimination in audit and correspondence studies . Journal of Human Resources , 47 , 1128 – 1157 .
Neumark D. , Bank R. J. , Van Nort K. D. ( 1996 ). Sex discrimination in restaurant hiring: an Audit Study . Quarterly Journal of Economics , 111 , 915 – 941 .
Petit P. ( 2007 ). The effects of age and family constraints on gender hiring discrimination: a field experiment in the French financial sector . Labour Economics , 14 , 371 – 391 .
Quadlin N. ( 2018 ). The mark of a woman’s record: gender and academic performance in hiring . American Sociological Review , 83 , 331 – 360 .
Reskin B. F. , Roos P. A. ( 1990 ). Job Queues, Gender Queues: Explaining Women’s Inroads into Male Occupations . Philadelphia : Temple University Press .
Riach P. A. , Rich J. ( 2002 ). Field experiments of discrimination in the market place . The Economic Journal , 112 , F480 – F518 .
Ridgeway C. L. , Correll S. J. ( 2004 ). Motherhood as a status characteristic . Journal of Social Issues , 60 , 683 – 700 .
Rudman L. A. , Glick P. ( 2001 ). Prescriptive gender stereotypes and Backlash toward agentic women . Journal of Social Issues , 57 , 743 – 762 .
Sahni H , Paul S.L. ( 2010 ). Women in top management and job self selection . Social Science Research Network Electronic Journal . http://dx.doi.org/10.2139/ssrn.2870673 (December 2018).
Russo N. ( 1976 ). The motherhood mandate . Journal of Social Issues , 32 , 143 – 153 .
Skyt Nielsen H. , Simonsen M. , Verner M. ( 2004 ). Does the gap in family-friendly policies drive the family gap? Scandinavian Journal of Economics , 106 , 721 – 744 .
Uggen C. et al. ( 2014 ). The edge of stigma: an experimental audit of the effects of low‐level criminal records on employment . Criminology , 52 , 627 – 654 .
Weichselbaumer D. ( 2004 ). Is it sex or personality? The impact of sex-stereotypes on discrimination in applicant selection . Eastern Economic Journal , 30 , 159 – 186 .
. | Male-dominated . | Mixed . | Female-dominated . | . | . |
---|---|---|---|---|---|
Non-Managerial | Delivery men/women 93 (374) | Waiting persons 97 (462) | Sales clerks 84 (336) | Low | Educational level |
Computer technicians 104 (416) | Estate agents 108 (432) | Office clerks 94 (376) | Medium | ||
Industrial engineers 70 (280) | Tax advisors 107 (428) | Physiotherapists 71 (296) | High | ||
Managerial | Foremen/women 34 (136) | Head chefs 108 (432) | Store managers 24 (96) | Low | |
Heads of logistics 77 (308) | Warehouse managers 58 (232) | Supervising clerks 40 (198) | Medium | ||
Marketing directors 64 (256) | Senior lawyers 108 (432) | Senior nurses 31 (130) | High |
Marta Gutierrez Ramírez Date of birth: July 2, 1977 Street X, n° - Barcelona |
---------------------------------------------------
Tax advisor
C & O Auditors
March 2014—currently
GRUPO GTA CONSULTING
February 2011 to January 2013
AUDIAXIS Taxers SL.
March 2004 to November 2006
CARSOE Industries
February 2000 to September 2001
Business Administration and Management
University of Girona
October 1994-November 1998
Resume (uploaded)
Additional information (optional):
Married with children.
I graduated in Business Administration and Management, specialized in taxation.
I am passionate about my work, and I want to continue learning.
Cristina Castillo Martínez Date of birth: December 14, 1979 Street X, n° - Barcelona |
Hewlett Packard
January 2011 to December 2012
GRUPO EMPRESARIAL RIBE ALAT SL.
Julio 2008 to December 2010
TGG Legal Servicios Jurídicos, fiscales y asesores financieros
February 2001 to September 2001
Pompeu Fabra University
September 1996 to September 1999
Married, no children.
I am a tax consultant in Barcelona, where I live with my husband. I love my job, and I am demanding with my own work.
Marc Domínguez Molina Date of birth: January 1, 1979 Street X, n° - Barcelona |
BIOCOP PRODUCTOS BIOLÓGICOS
Financial Projects DUA
February 2011 to December 2012
SEGESTION S.L.
July 2008 to December 2010
AGROFRESC, S.L.
PLETA AUDITORES
Universitat Autònoma de Barcelona (UAB)
October 1995-November 1998
Father of two kids.
Tax expert:
– Company law: advice to the company and its partners in all phases of their social life
– Commercial contracting: banking, collaboration, agency, franchise, distribution, etc.
– Bank and financial Law
José Antonio Cortés Rubio Date of birth: August 27, 1979 Street X, n° - Barcelona |
SECONDA Obras y Construcciones S.A.
Aruba Advisors
MAZARS ASOCIATE LAERS AND TAX ADVISORS
AUDITIA IBERIA
Granja d’en Roca
University of Barcelona
October 1996-November 1999
Married, without children.
I am a tax consultant and accountant. I have worked in five companies so far. I have learned a lot from all of them, and they have all supported me throughout my career to achieve current position.
*Resumes included a personalized telephone number, address, and e-mail. The names of universities and companies are real.
Author notes
Supplementary data.
Month: | Total Views: |
---|---|
March 2019 | 656 |
April 2019 | 653 |
May 2019 | 890 |
June 2019 | 753 |
July 2019 | 454 |
August 2019 | 528 |
September 2019 | 737 |
October 2019 | 1,411 |
November 2019 | 1,217 |
December 2019 | 927 |
January 2020 | 904 |
February 2020 | 1,090 |
March 2020 | 1,003 |
April 2020 | 801 |
May 2020 | 876 |
June 2020 | 1,106 |
July 2020 | 810 |
August 2020 | 858 |
September 2020 | 2,422 |
October 2020 | 2,421 |
November 2020 | 2,795 |
December 2020 | 2,218 |
January 2021 | 2,055 |
February 2021 | 2,767 |
March 2021 | 3,908 |
April 2021 | 3,370 |
May 2021 | 3,099 |
June 2021 | 1,978 |
July 2021 | 1,665 |
August 2021 | 1,507 |
September 2021 | 2,536 |
October 2021 | 2,880 |
November 2021 | 3,220 |
December 2021 | 2,395 |
January 2022 | 2,006 |
February 2022 | 2,216 |
March 2022 | 3,006 |
April 2022 | 2,593 |
May 2022 | 2,116 |
June 2022 | 1,244 |
July 2022 | 951 |
August 2022 | 772 |
September 2022 | 1,090 |
October 2022 | 1,353 |
November 2022 | 1,227 |
December 2022 | 1,011 |
January 2023 | 959 |
February 2023 | 1,066 |
March 2023 | 1,415 |
April 2023 | 1,226 |
May 2023 | 1,181 |
June 2023 | 737 |
July 2023 | 611 |
August 2023 | 672 |
September 2023 | 921 |
October 2023 | 1,242 |
November 2023 | 1,319 |
December 2023 | 1,038 |
January 2024 | 903 |
February 2024 | 887 |
March 2024 | 1,250 |
April 2024 | 1,283 |
May 2024 | 933 |
June 2024 | 662 |
Email alerts
Citing articles via.
- Recommend to your Library
Affiliations
- Online ISSN 1468-2672
- Print ISSN 0266-7215
- Copyright © 2024 Oxford University Press
- About Oxford Academic
- Publish journals with us
- University press partners
- What we publish
- New features
- Open access
- Institutional account management
- Rights and permissions
- Get help with access
- Accessibility
- Advertising
- Media enquiries
- Oxford University Press
- Oxford Languages
- University of Oxford
Oxford University Press is a department of the University of Oxford. It furthers the University's objective of excellence in research, scholarship, and education by publishing worldwide
- Copyright © 2024 Oxford University Press
- Cookie settings
- Cookie policy
- Privacy policy
- Legal notice
This Feature Is Available To Subscribers Only
Sign In or Create an Account
This PDF is available to Subscribers Only
For full access to this pdf, sign in to an existing account, or purchase an annual subscription.

- The University of Sydney
- University of Sydney Business School
- Research Publications and Outputs
Show simple item record
Gender equitable recruitment and promotion: Leading practice guide
Field | Value | Language |
dc.contributor.author | Foley, Meraiah | |
dc.contributor.author | Cooper, Rae | |
dc.contributor.author | Mosseri, Sarah | |
dc.date.accessioned | 2019-10-24 | |
dc.date.available | 2019-10-24 | |
dc.date.issued | 2019-08-01 | |
dc.identifier.citation | Foley, M; Cooper, R; and Mosseri S (2019). Gender equitable recruitment and promotion: Leading practice guide, WGEA Commissioned Research Paper, The Australian Women’s Working Futures (AWWF) Project, University of Sydney, Sydney, Australia. | en_US |
dc.identifier.uri | https://hdl.handle.net/2123/21261 | |
dc.description.abstract | Gender bias is pervasive at work and in organisations, creating inequalities at every stage of the employment cycle. Gender- based stereotypes affect which candidates get recruited for certain roles and which do not, which candidates get selected for those roles and why, how salaries are negotiated, how managers provide feedback to their employees, and which employees receive career development opportunities and career encouragement and which do not. Each of these factors compounds across women’s careers, producing and sustaining gender inequality from recruitment to selection to promotion. Decades of research has made one thing clear: gender biases are nearly always present in employment decisions, subtly influencing our assessments about who is the ‘right’ or ‘best’ person for the job. This insight paper highlights some of the research examining how gender bias operates at work and provides evidence- based suggestions for creating more equitable recruitment and promotion systems. | en_US |
dc.description.sponsorship | Workplace Gender Equality Agency; The Australian Women’s Working Futures (AWWF) Project, University of Sydney, Sydney, Australia. | en_US |
dc.language.iso | en_AU | en_US |
dc.publisher | Australian Government Workplace Gender Equality Agency | en_US |
dc.subject | Gender bias | en_US |
dc.subject | Workplace | en_US |
dc.subject | Work | en_US |
dc.subject | Recruitment | en_US |
dc.subject | Gender inequality | en_US |
dc.title | Gender equitable recruitment and promotion: Leading practice guide | en_US |
dc.type | Report, Technical | en_AU |
dc.subject.asrc | FoR::150305 - Human Resources Management | en_US |
dc.subject.asrc | FoR::150399 - Business and Management not elsewhere classified | en_US |
dc.subject.asrc | FoR::200205 - Culture, Gender, Sexuality | en_US |
dc.type.pubtype | Publisher version | en_US |
Associated file/s
Associated collections, version history.
There are no previous versions of the item available.
Use filters to refine the search results.
Clear Add New Filter Apply
Show statistical information
Export search results
The export option will allow you to export the current search results of the entered query to a file. Different formats are available for download. To export the items, click on the button corresponding with the preferred download format.
Administrators can export up to 15000 items. Regular users can export no more than 500 items.
To select a subset of the search results, click "Selective Export" button and make a selection of the items you want to export. The amount of items that can be exported at once is similarly restricted as the full export.
After making a selection, click one of the export format buttons. The amount of items that will be exported is indicated in the bubble next to export format.
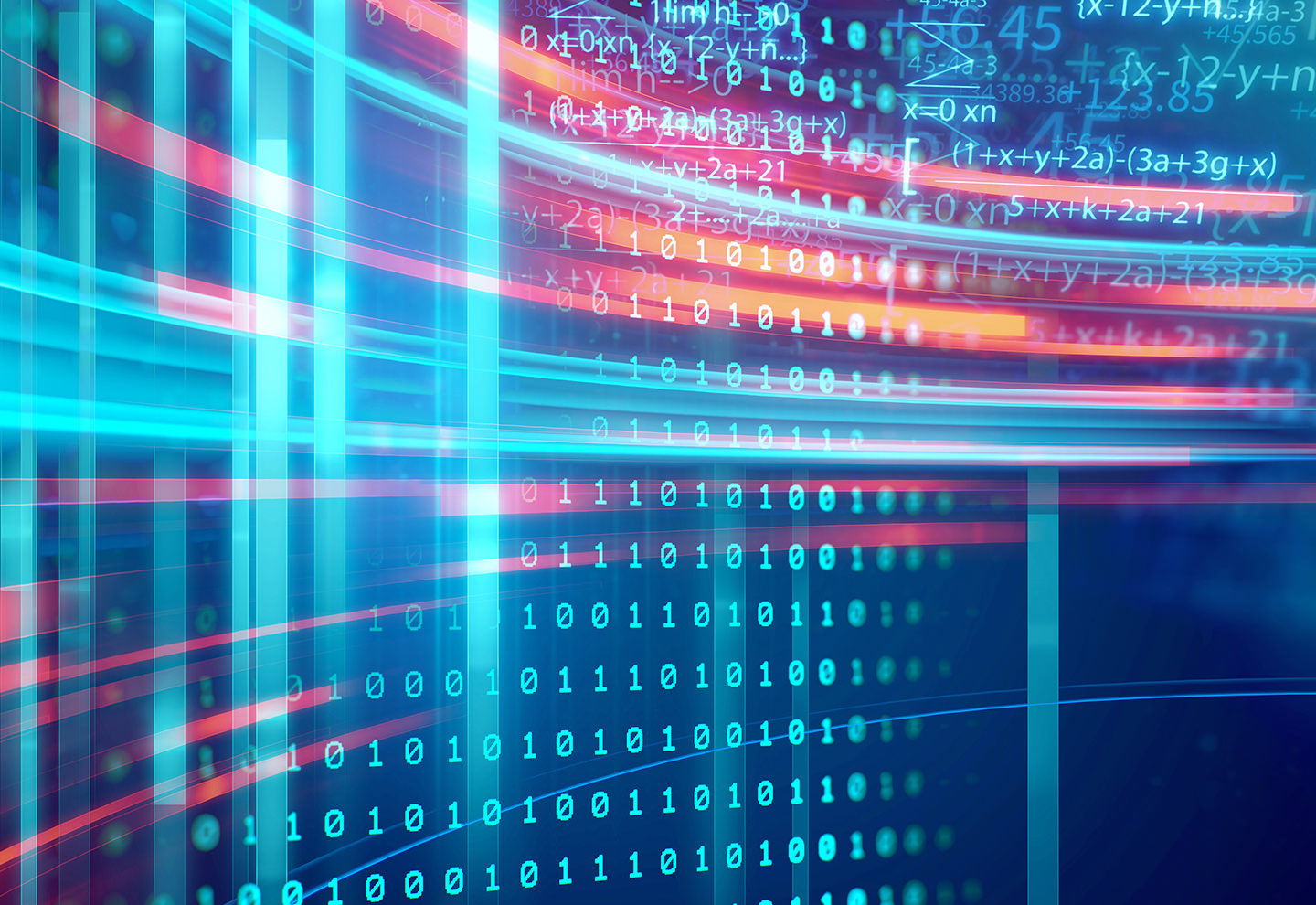
Amazon's sexist hiring algorithm could still be better than a human
Amazon decided to shut down its experimental artificial intelligence (AI) recruiting tool after discovering it discriminated against women. The company created the tool to trawl the web and spot potential candidates, rating them from one to five stars. But the algorithm learned to systematically downgrade women’s CV’s for technical jobs such as software developer.
Although Amazon is at the forefront of AI technology, the company couldn’t find a way to make its algorithm gender-neutral. But the company’s failure reminds us that AI develops bias from a variety of sources. While there’s a common belief that algorithms are supposed to be built without any of the bias or prejudices that colour human decision making, the truth is that an algorithm can unintentionally learn bias from a variety of different sources. Everything from the data used to train it, to the people who are using it, and even seemingly unrelated factors, can all contribute to AI bias.
AI algorithms are trained to observe patterns in large data sets to help predict outcomes. In Amazon’s case, its algorithm used all CVs submitted to the company over a ten-year period to learn how to spot the best candidates. Given the low proportion of women working in the company, as in most technology companies , the algorithm quickly spotted male dominance and thought it was a factor in success.
Because the algorithm used the results of its own predictions to improve its accuracy, it got stuck in a pattern of sexism against female candidates. And since the data used to train it was at some point created by humans, it means that the algorithm also inherited undesirable human traits, like bias and discrimination, which have also been a problem in recruitment for years.
Some algorithms are also designed to predict and deliver what users want to see. This is typically seen on social media or in online advertising, where users are shown content or advertisements that an algorithm believes they will interact with . Similar patterns have also been reported in the recruiting industry.
One recruiter reported that while using a professional social network to find candidates, the AI learned to give him results most similar to the profiles he initially engaged with. As a result, whole groups of potential candidates were systematically removed from the recruitment process entirely.
However, bias also appears for other unrelated reasons. A recent study into how an algorithm delivered ads promoting STEM jobs showed that men were more likely to be shown the ad, not because men were more likely to click on it, but because women are more expensive to advertise to. Since companies price ads targeting women at a higher rate (women drive 70% to 80% of all consumer purchases), the algorithm chose to deliver ads more to men than to women because it was designed to optimise ad delivery while keeping costs low.
But if an algorithm only reflects patterns in the data we give it, what its users like, and the economic behaviours that occur in its market, isn’t it unfair to blame it for perpetuating our worst attributes? We automatically expect an algorithm to make decisions without any discrimination when this is rarely the case with humans. Even if an algorithm is biased, it may be an improvement over the current status quo.
To fully benefit from using AI, it’s important to investigate what would happen if we allowed AI to make decisions without human intervention. A 2018 study explored this scenario with bail decisions using an algorithm trained on historical criminal data to predict the likelihood of criminals re-offending. In one projection, the authors were able to reduce crime rates by 25% while reducing instances of discrimination in jailed inmates.
Yet the gains highlighted in this research would only occur if the algorithm was actually making every decision. This would be unlikely to happen in the real world as judges would probably prefer to choose whether or not to follow the algorithm’s recommendations. Even if an algorithm is well designed, it becomes redundant if people choose not to rely on it.
Many of us already rely on algorithms for many of our daily decisions, from what to watch on Netflix or buy from Amazon. But research shows that people lose confidence in algorithms faster than humans when they see them make a mistake, even when the algorithm performs better overall.
For example, if your GPS suggests you use an alternative route to avoid traffic that ends up taking longer than predicted, you’re likely to stop relying on your GPS in the future. But if taking the alternate route was your decision, it’s unlikely you will stop trusting your own judgement. A follow-up study on overcoming algorithm aversion even showed that people were more likely to use an algorithm and accept its errors if given the opportunity to modify the algorithm themselves, even if it meant making it perform imperfectly.
While humans might quickly lose trust in flawed algorithms, many of us tend to trust machines more if they have human features. According to research on self-driving cars , humans were more likely to trust the car and believed it would perform better if the vehicle’s augmented system had a name, a specified gender, and a human-sounding voice. However, if machines become very human-like, but not quite, people often find them creepy , which could affect their trust in them.
Even though we don’t necessarily appreciate the image that algorithms may reflect of our society, it seems that we are still keen to live with them and make them look and act like us. And if that’s the case, surely algorithms can make mistakes too?
Maude Lavanchy is Research Associate at IMD.
This article was first published by The Conversation .
Research Information & Knowledge Hub for additional information on IMD publications
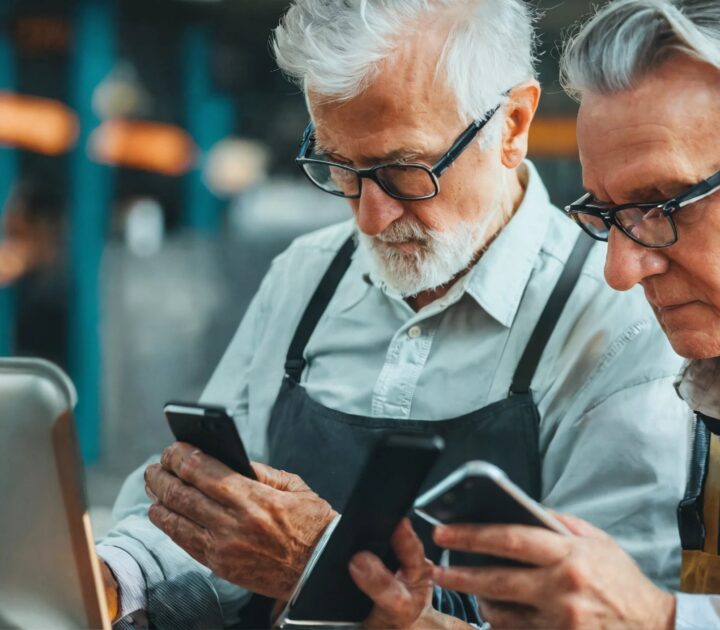
Too many organizations assume late-career workers can't keep up with new technology. In doing so, they are ignoring a hugely important labor pool.
The payment processing industry in Europe had operated in largely the same way for decades. A standard system of financial processing and clearing ...
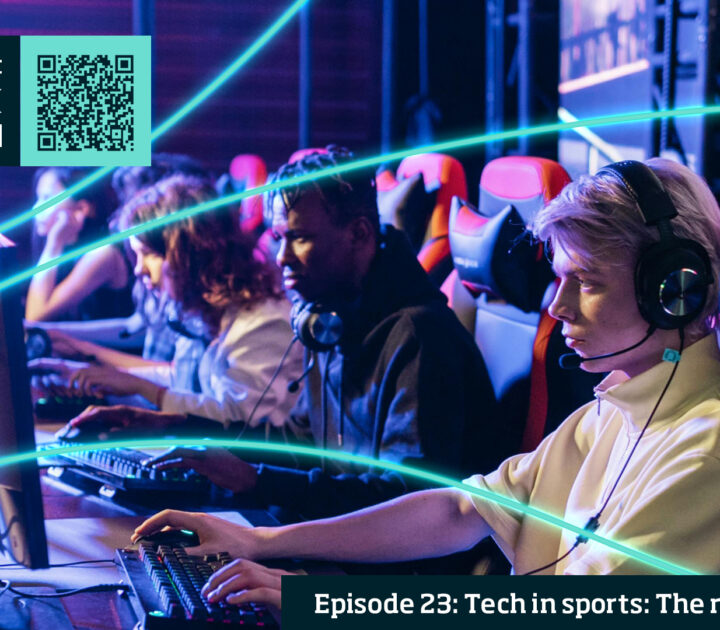
Since the dawn of video games, there have been gaming competitions. But for decades, such contests were regarded as beneath the hallowed realm of '...
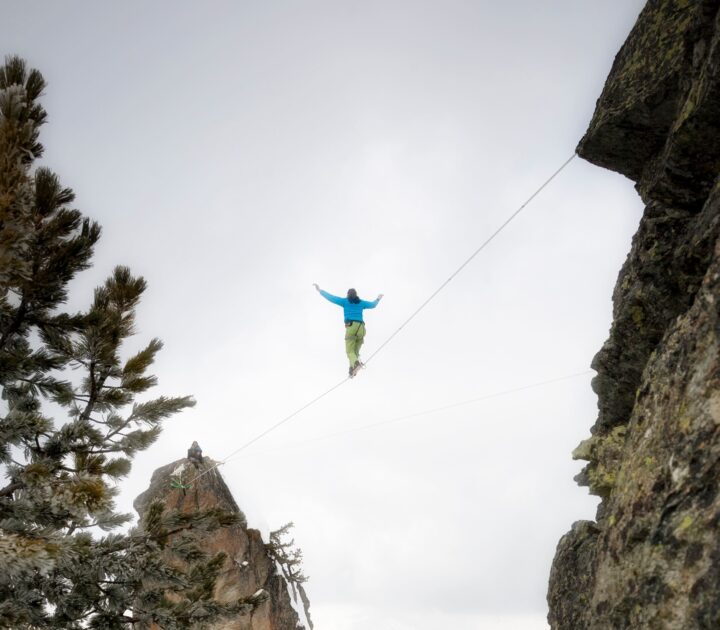
Understanding your organizational capacity for agility and responding to events accordingly is the key to success in the new business environment, ...
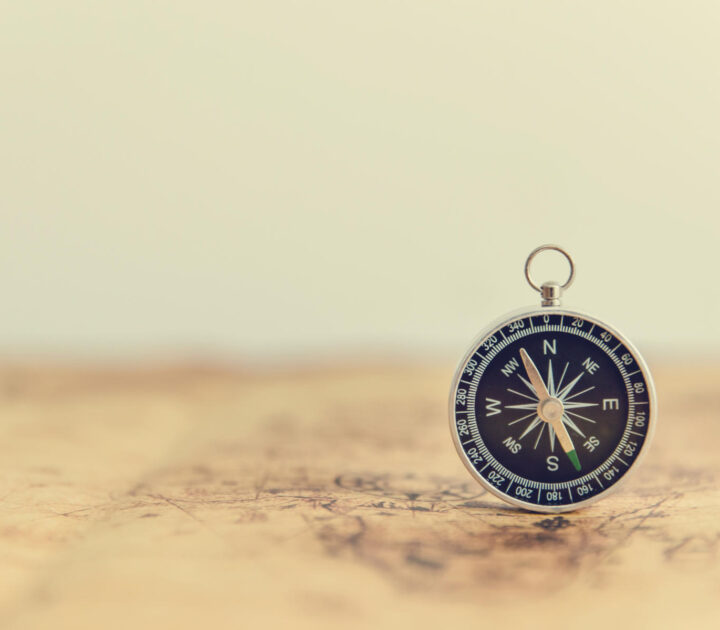
In a post-pandemic era shaped by wars, climate change, and new technologies, Maha Hosain Aziz outlines the main risks and shock events that could d...
The case study examines recent aviation safety concerns at Boeing, focusing on manufacturing issues, leadership decisions and regulatory oversight....
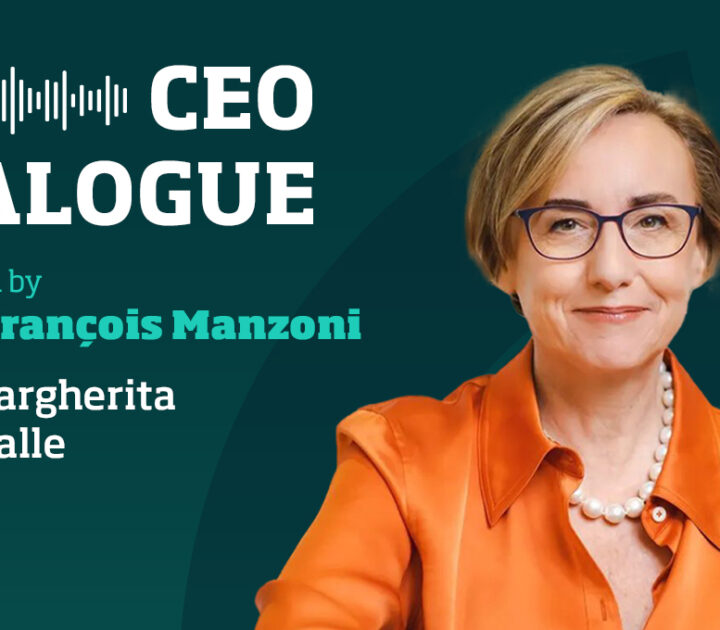
Margherita Della Valle tells IMD President Jean-François Manzoni how she is delivering radical change to reenergize Vodafone and paving the way for...
For managers and teams, this is an unhealthy rapport. Here’s how to stop rolling your eyes and start treating each other like full-fledged adults. ...
The case is seen through the eyes of the newly appointed supply chain director at a cosmetics company based in Berlin. The general manager has task...
Few Business to Business (B2B) marketplaces have succeeded. Metalshub has successfully combined a software platform as a service, with a marketplac...
Insight - Amazon scraps secret AI recruiting tool that showed bias against women
- Medium Text
Reporting By Jeffrey Dastin in San Francisco; Editing by Jonathan Weber and Marla Dickerson
Our Standards: The Thomson Reuters Trust Principles. New Tab , opens new tab
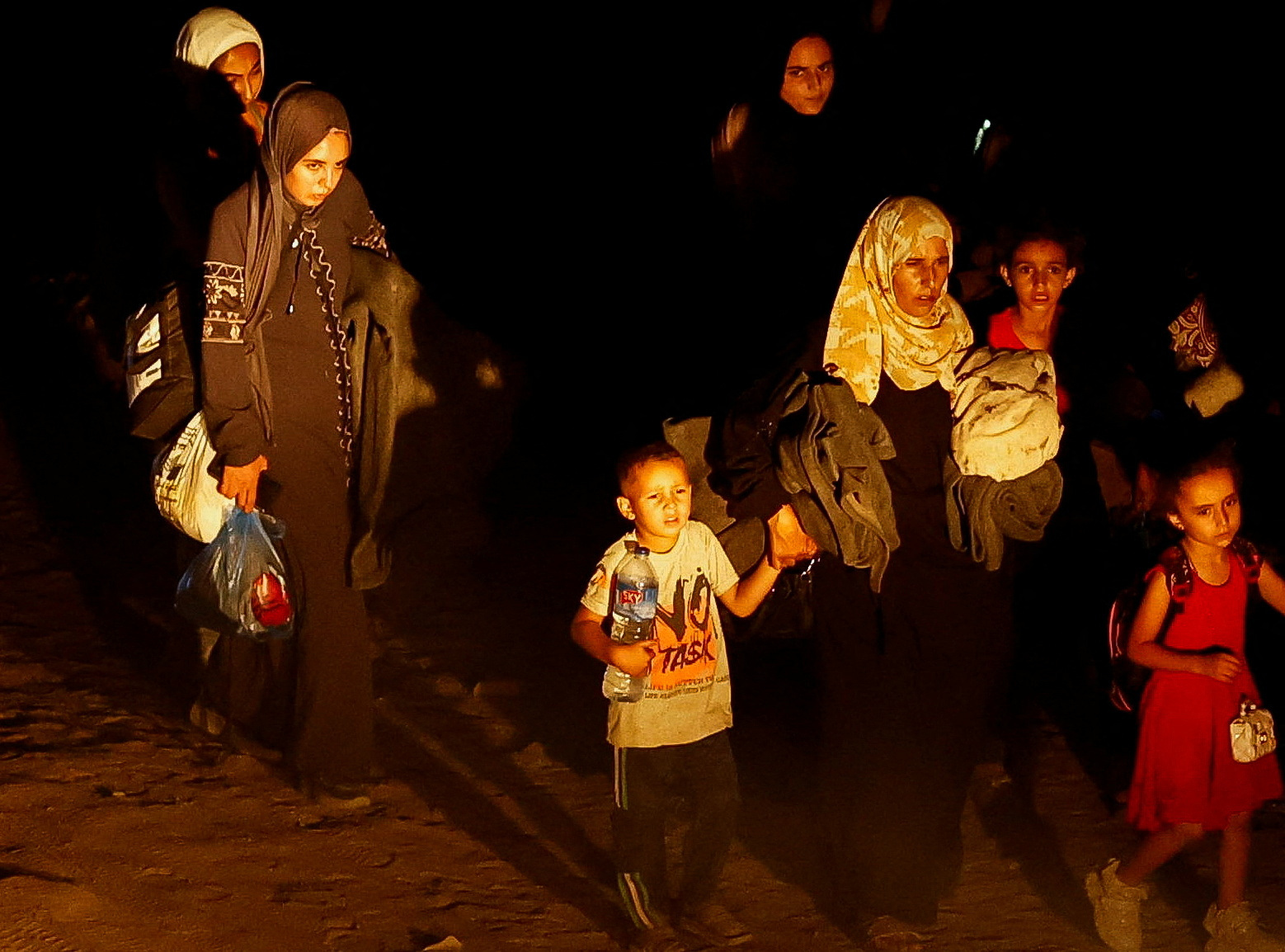
World Chevron
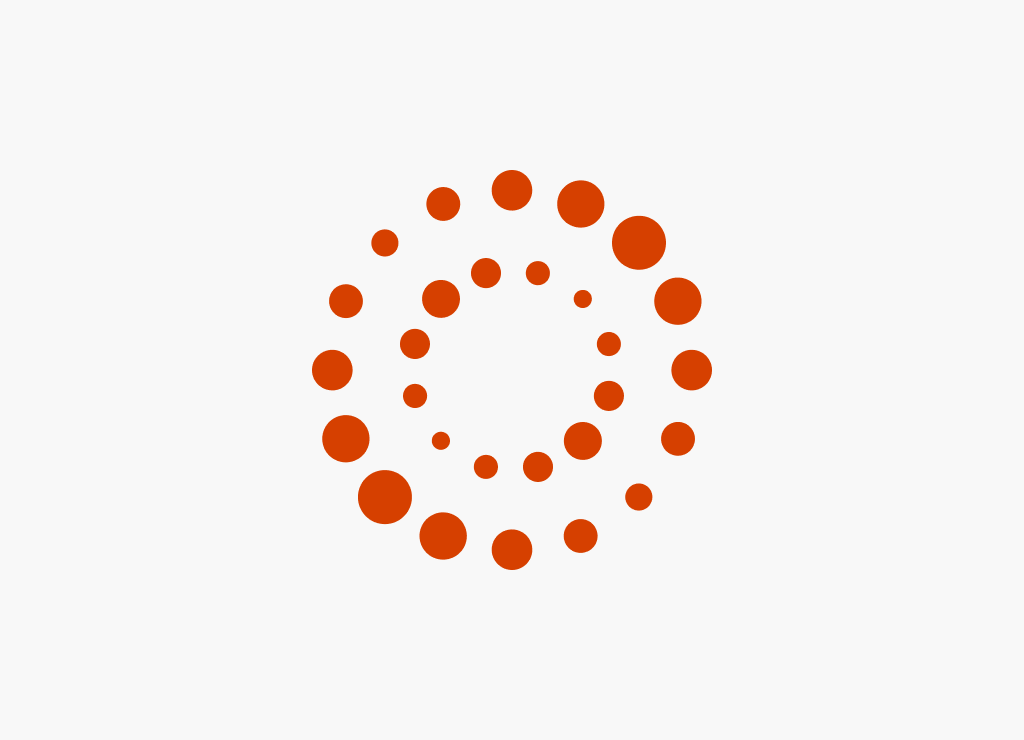
Russian official says Ukrainian shelling of Russia's Belgorod region kills one, injures 9
The governor of Russia's Belgorod region said in a statement on Monday that a civilian had been killed in Ukrainian shelling of an agricultural facility.
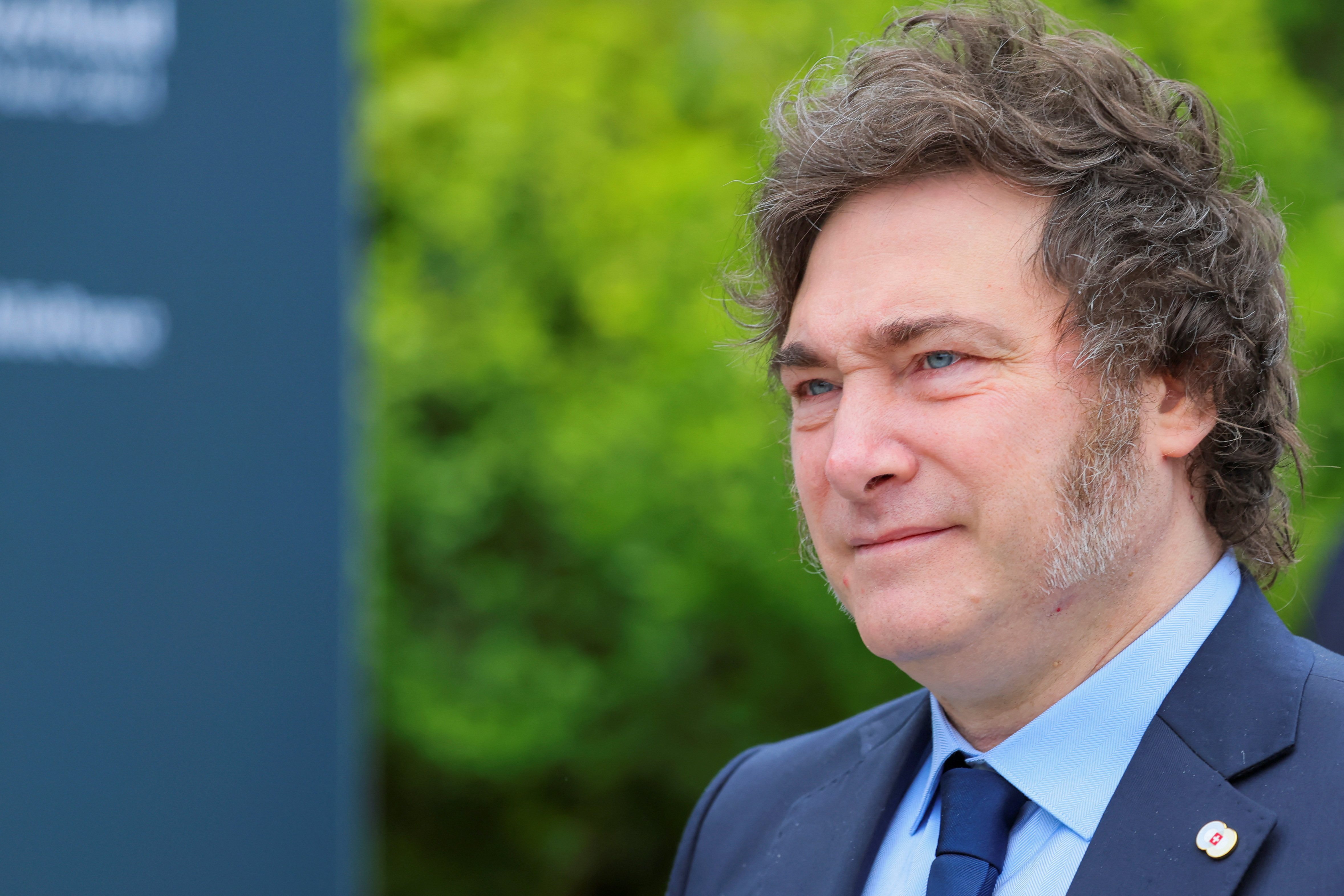
Gender Discrimination in the Hiring of Skilled Professionals in Two Male-Dominated Occupational Fields: A Factorial Survey Experiment with Real-World Vacancies and Recruiters in Four European Countries
Geschlechterdiskriminierung bei der Stellenbesetzung von Fachkräften in zwei männlich dominierten Berufen: Eine Vignettenstudie mit realen Stelleninseraten und Personalverantwortlichen in vier europäischen Ländern
- Abhandlungen
- Berufe und Geschlechterungleichheit
- Open access
- Published: 01 July 2020
- Volume 72 , pages 261–289, ( 2020 )
Cite this article
You have full access to this open access article
- Ariane Bertogg 1 ,
- Christian Imdorf 2 ,
- Christer Hyggen 3 ,
- Dimitris Parsanoglou 4 &
- Rumiana Stoilova 5
14k Accesses
14 Citations
22 Altmetric
Explore all metrics
The present article investigates gender discrimination in recruitment for two male-dominated occupations (mechanics and IT professionals). We empirically test two different explanatory approaches to gender discrimination in hiring; namely, statistical discrimination and taste-based discrimination. Previous studies suggest that, besides job applicants’ characteristics, organisational features play a role in hiring decisions. Our article contributes to the literature on gender discrimination in the labour market by investigating its opportunity structures located at the recruiter, job and company level, and how gender discrimination varies across occupations and countries.
The analysed data come from a factorial survey experiment conducted in four countries (Bulgaria, Greece, Norway and Switzerland). Real job advertisements were sampled, and the recruiters in charge of hiring for these positions ( n = 1,920) rated up to ten hypothetical CVs (vignettes). We find gender discrimination in Bulgaria and Greece and to a lesser degree in Switzerland, but not in Norway. The degree of gender discrimination appears to be greater in mechanics than in IT. Multivariate analyses that test a number of opportunity structures for discrimination suggest that mechanisms of statistical discrimination rather than those of taste-based discrimination might be at work.
Zusammenfassung
Im vorliegenden Beitrag wird die Geschlechterdiskriminierung im Rekrutierungsprozess in zwei männlich dominierten Berufen (Mechanikerinnen und Mechaniker, Informatikerinnen und Informatiker) untersucht. Dabei werden verschiedene Erklärungsansätze für das Vorkommen einer systematischen Benachteiligung von Frauen empirisch überprüft, nämlich statistische Diskriminierung und Taste-based-Diskriminierung. Frühere Studien haben ergeben, dass nicht nur individuelle Eigenschaften von Bewerberinnen und Bewerbern sondern auch organisationale Charakteristika eine Rolle spielen. Die Studie erweitert die Forschungsliteratur zu Geschlechterdiskriminierung, indem ihre Opportunitätsstrukturen auf Ebene von Personalreferentinnen und Personalreferenten, Stellen und Betriebe untersucht werden und ihre kontextspezifische Wirkungsweise in unterschiedlichen Berufen sowie Ländern aufgezeigt wird.
Die Analysen basieren auf einer Vignettenstudie, die in vier Ländern durchgeführt wurde (Bulgarien, Griechenland, Norwegen und Schweiz). Dazu wurden reale Stellenanzeigen gesampelt und die zuständigen Personalverantwortlichen ( n = 1920) befragt. Jede befragte Person bewertete bis zu zehn hypothetische Lebensläufe (Vignetten). Die Ergebnisse zeigen, dass Geschlechterdiskriminierung vor allem in Bulgarien, Griechenland und zu einem geringeren Teil auch in der Schweiz vorkommt, nicht jedoch in Norwegen. Darüber hinaus ist das Ausmaß der Geschlechterdiskriminierung größer im Mechanikerberuf als in IT-Berufen. Die multivariaten Analysen, die mehrere Opportunitätsstrukturen von Diskriminierung untersuchen, belegen, dass eher Mechanismen der statistischen Diskriminierung als jene der Taste-based-Diskriminierung am Werk sind.
Similar content being viewed by others
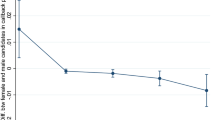
Mind the Job: The Role of Occupational Characteristics in Explaining Gender Discrimination
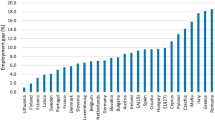
Assessing the role of gender in hiring: a field experiment on labour market discrimination
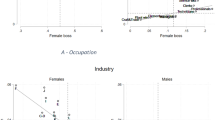
What if your boss is a woman? Evidence on gender discrimination at the workplace
Avoid common mistakes on your manuscript.
1 Introduction
The educational attainment of women has considerably improved owing to educational expansion. Today, girls and women outperform boys and men in school and higher education respectively. Moreover, women’s enrolment in subjects previously deemed the domains of men has increased considerably (DiPrete and Buchmann 2013 ). Likewise, the considerable growth of the service sector (Hakim 1996 ) has changed labour market structures in favour of women’s employment (Charles 2003 ). Nevertheless, labour markets remain highly segregated by gender. Researchers have shown links between horizontal gender segregation—the unequal distribution of gender across occupations—and vertical segregation, that is, where female-dominated jobs pay less and offer fewer opportunities for professional development (England 2010 ; Leuze and Strauß 2016 ). Further, organisational constraints may push women into “family-friendly” occupations, which offer flexibility or enable part-time work in order to combine employment with domestic activities (Levanon et al. 2009 ).
Sociologists recurrently rely upon three explanations for the persistence of horizontal gender segregation. First, horizontal gender segregation is partly the result of self-selective behaviour. Women may be attracted to “family-friendly” occupations (e.g., in the service sector) rather than occupations in Science, Technology, Engineering and Mathematics (STEM, see Herman et al. 2013 ; Mastekaasa and Smeby 2008 ). Second, employers’ preferences for applicants of a certain gender—independent of the job candidates’ skills and abilities—can perpetuate horizontal gender segregation (Kricheli-Katz 2019 ). Such employer behaviour is usually known as gender discrimination in hiring (Birkelund et al. 2019 ; Kübler et al. 2018 ; Riach and Rich 2006 ). Third, horizontal segregation may be the outcome of aggregation processes such as the de-valuation of female-dominated occupations (Levanon et al. 2009 ; Leuze and Strauß 2016; Ochsenfeld 2014 ).
In this article, we provide further insight into the second field of explanations. For many decades, extensive economic and sociological literature has focused on gender discrimination in hiring. The two most prominent explanations—Becker’s ( 1957 ) work on taste-based discrimination and the theory of statistical discrimination (Arrow 1973 ; Phelps 1972 )—are rooted in economics. As such, both theoretical approaches assume that propensities to discriminate are constant over time and across social contexts (Keuschnigg and Wolbring 2016 ). The conditions under which employers’ preferences translate into unequal treatment of job candidates remain underexplored. Reskin ( 2003 ), for instance, has argued that prejudices and stereotypes may only unfold if organisational contexts and practices allow them to do so. Peterson and Saporta ( 2004 ) have termed this phenomenon the “opportunity structure for discrimination”.
These opportunity structures have a range of occupational characteristics. According to Kübler et al. ( 2018 ), female candidates are subject to some degree of discrimination in male-dominated, particularly STEM, occupations. The degree of gender discrimination in this male-dominated field may vary according to the extent of gender-stereotyping attached to a given occupation (Yavorsky 2019 ). STEM occupations are relevant to study, as the phenomenon of the “leaky pipeline”, i.e. the decreasing proportion of women at the various qualification levels and career stages, is particularly salient (Blickenstaff 2005 ; Herman et al. 2013 ). Exactly how this phenomenon differs among the specific occupations within the broader STEM cluster remains under-researched, as does the degree to which gender discrimination can be explained by recruiter preferences and the characteristics of the vacancy and company. Last but not least, little is known about how discrimination varies across country contexts (Quillian et al. 2019 ).
We take the sociological critique of neoclassic economic models of discrimination as a starting point and contribute to the existing body of literature by analysing the opportunity structures for gender discrimination in different occupational and national contexts. We provide a case study of recruiter behaviour in the hiring process in four countries for two male-dominated occupations—namely, mechanics and information technology (IT) professionals, both technical occupations that nevertheless vary in terms of required social skills (Barone 2011 ), status (Kricheli-Katz 2019 ) and educational pathways. We address the following questions:
1. How do recruiters hiring mechanics and IT professionals assess male and female applicants differently?
2. Through what mechanisms at the recruiter, job, company and country levels can gender discrimination in hiring be explained?
Our empirical analyses draw on data stemming from the Employer Survey (WP7) of the NEGOTIATE project (Imdorf et al. 2019 ). The Employer Survey consists of an internationally comparative factorial survey experiment and overcomes previous difficulties in measuring discrimination across national contexts (Quillian et al. 2019 ). Real job advertisements were sampled in Bulgaria (BG), Greece (GR), Norway (NO), and Switzerland (CH). The remainder of this article is structured as follows. First, we describe mechanisms of gender discrimination in hiring and contextual factors explaining variation therein. Thereafter, we describe the research design, data, and the methods applied. Subsequently we present and discuss the empirical findings. In the last part, we summarise our findings and conclude the article with a discussion.
2 Mechanisms of Gender Discrimination in Hiring
2.1 economic theories and research strategies to study gender discrimination in hiring.
Sociological analysis of gender discrimination in hiring and its methodology have developed considerably since the early proposition of two general types of labour market discrimination models advanced by neoclassical economists: Gary Becker’s ( 1957 ) taste-for-discrimination model and the model of statistical discrimination (Arrow 1973 ; Phelps 1972 ). Becker assumes that employers, fellow workers or customers aim for a certain profile regarding candidates’ social and psychological characteristics and seek to avoid members of certain groups—for example, men who feel uncomfortable being supervised by women. The model of statistical discrimination proposes that employers use either valid information or stereotypes about average group characteristics in order to reduce asymmetric information and predict individual worker ability and productivity—for example, assuming women and mothers to be absent from the workplace more often owing to family duties.
Guryan and Charles ( 2013 ) point out that, after an initial debate in the 1970s and 1980s about whether one of the two models better described (gender) discrimination, the literature has only recently returned to the question of whether taste-based or statistical discrimination is a more appropriate description of the phenomenon of discrimination. These more contemporary analyses of discrimination differ from the early neoclassical approaches both in their definition and in their measurement of discrimination (Keuschnigg and Wolbring 2016 ). Economists initially defined discrimination as a difference in earnings between two groups of workers of equal productivity—the gender gap in wages, for instance—and applied regression-based methods to prove their theoretical assumptions. But such regression-based approaches have been criticized for their limited ability to isolate the portion of economic inequality that may be ascribed to discrimination (Guryan and Charles 2013 ).
This criticism has initiated a shift to alternative methods, particularly field experiments and measurements of discrimination. Two common methods shall be briefly mentioned: first, in-person audit studies, in which actors are sent to job interviews pretending to be candidates (see, for instance, Neumark et al. 1996 ; who found evidence of discrimination against women applying to be waiting staff in high-priced restaurants in the USA). Second, correspondence tests, where a set of fictitious resumés is sent to real job openings, with call-back rates being the dependent variable (e.g. Riach and Rich 2006 for a British study that found significant discrimination against men in “female occupations” and against women in “male occupations”; for an extensive list of recent correspondence experiments, see Baert 2018 ). Use of these two designs has shifted the focus of analysis from the worker’s economic outcome to the employer’s behaviour (Guryan and Charles 2013 ). Discrimination is now measured as differences in hiring behaviour.
Field experiments allow researchers to test more precisely specific assumptions by either the taste-based discrimination or the statistical discrimination model (see, for example, Weichselbaumer 2004 ). However, Keuschnigg and Wolbring ( 2016 ) argue that neither correspondence tests nor in-person audits manage to identify causal mechanisms behind discriminatory behaviour. While field experiments enable the measurement of discrimination in employment, they can hardly explain why employers discriminate based on gender, ethnicity or age (Imdorf 2017 ). Moreover, those methods can pose serious ethical concerns (Keuschnigg and Wolbring 2016 , p. 190).
In contrast, factorial survey experiments (FSEs, or vignette studies), bypass such ethical concerns, and allow for simultaneously testing key assumptions underlying different models of discrimination. They constitute multidimensional experimental designs that implement vignettes representing hypothetical objects or situations (Auspurg and Hinz 2015 ). FSEs have been used in labour market research to gain knowledge about both recruiters’ and job seekers’ behaviour (Abraham et al. 2013 ; Van Belle et al. 2018 ). In the respective applications, recruiters receive brief descriptions of fictitious job applicants that vary with regard to specific attributes, and they are asked if they would consider hiring such a person. The experimental manipulation of the stimuli presented to the respondents allows researchers to combine several dimensions of candidates’ characteristics in an orthogonal design. Accordingly, the major advantage of such a design is its high internal validity, as the vignette characteristics do not correlate with each other. So far, only a few studies have applied FSEs in dissecting the mechanisms of gender discrimination when employers hire. Kübler et al. ( 2018 ) have found that the degree of discrimination against women is the lower the higher the share of women in an occupation. At the same time, men may also experience discrimination in female-dominated occupations (Birkelund et al. 2019 ).
2.2 Sociological Perspectives on Gender Discrimination in Hiring
Economic analysis has made extensive use of the models of taste-based discrimination and statistical discrimination. However, both concepts are limited as long as they reduce employers’ decisions to assumptions about job candidates’ individual ability and productivity. Models that assume that taste-based or statistical discrimination helps to maximise companies’ productivity neglect the complexity of social coordination and dependencies in the workplace that frame a company’s productivity too (Imdorf 2017 ). Sociological reasoning facilitates the understanding of “collective models of discrimination”, where “groups act collectively against each other” (Autor 2008 , p. 2). From this perspective, productivity can be seen “as the outcome of social relations at the workplace, rather than solely the outcome of workers’ skills” (Shih 2002 , p. 102). Collective mechanisms can be assumed in situations in which the recruiter wants to ensure that the prospective employee will be an integral part of the work group. At the core of this exclusionary organisational process is the need for the “social fit” of job candidates. For instance, a candidate’s presumed ability to integrate into a work organisation dominated by one sex may be perceived conditional on that person’s sex (Bygren and Kumlin 2005 ). A qualitative study in the male-dominated Swiss automotive occupational field indicates that employers may refrain from hiring female apprentices as they fear that young women may distract the predominantly male workforce (Imdorf 2013 ). Kricheli-Katz ( 2019 ) finds evidence that women’s entrance into high-status occupations poses a threat to the identity and interests of high-occupational-status men. In fact, Becker’s ( 1957 ) model of taste-based discrimination, whereby an employer must pay a wage premium to men in order to win them to work in a gender-integrated setting, may be compatible with the assumption that employers respond to the interests of male employees when they exclude women from their job categories in the hiring process (Bielby and Baron 1986 ). Taken together, we can expect that in the two male-dominated occupations under study, female applicants are rated less positively than male applicants. Our first hypothesis thus reads as follows: female candidates in the occupational fields of mechanics and IT professionals have lower recruitment chances than male candidates (H1).
3 Contextual Factors Explaining Gender Discrimination in Hiring
Bielby and Baron ( 1986 ) assume a variation across jobs and establishments in the desire and ability of male workers to exclude women given the diversity of organisational and technical arrangements. Similarly, Reskin ( 2003 ) proposes that prejudices and stereotypes only unfold if organisational contexts and practices allow for it. It is therefore of interest to better understand under which conditions discriminatory preferences translate into unequal treatment (Keuschnigg and Wolbring 2016 ). These conditional contexts are located at various levels, such as recruiter, vacancy, company, occupation, or country. In the following section, we sketch a number of relevant opportunity structures for gender discrimination at different levels.
3.1 Recruiter, Job and Company Characteristics
At the recruiter level , Cole et al. ( 2004 ) have analysed whether recruiters’ and applicants’ gender influence recruiters’ judgments regarding applicants’ resumés, which reflects a form of taste-based discrimination. They found that female recruiters perceived male resumés to report more work experience than female resumés, whereas male recruiters perceived female applicants as having more extracurricular interests than male applicants. Gorman ( 2005 ) analysed hiring processes in large US law firms and found evidence that organisational decision makers favour candidates of their own gender. Generally, female decision makers fill more vacancies with women than do male decision makers. Kricheli-Katz ( 2019 ) finds that female managers value female job candidates more highly than male managers when hiring for previously male-dominated high-status occupations. Hence, gender discrimination patterns may differ according to the recruiters’ own gender.
At the job level , multiple characteristics of a vacancy may influence gender discrimination in hiring. Statistical discrimination may increase with a higher requirement of work experience , a job criterion that can be used as a gendered sorting mechanism (Ranson and Reeves 1996 ). As the authors show, female candidates are more likely to be accepted for computer professional jobs that do not require much work experience. Economic job dimensions include the importance of filling a vacancy , the salary of the position and the job status (in terms of authority and/or seniority). Women are often discriminated against in the hiring for senior or high-wage positions (Fernandez and Mors 2008 ; Riach and Rich 2006 ; Petit 2007 ; Yavorsky 2019 ). On the one hand, hiring for jobs with higher costs increases the degree of risk aversion on the recruiter’s side, with risk-averse employers being more prone to activating stereotypes (Reskin and Branch McBrier 2000 ). On the other hand, women may have better chances of being recruited for part-time positions, as such jobs are viewed as being most compatible with (future) motherhood (Herman et al. 2013 ; Pedulla 2016 ). In accordance with neoclassical economic assumptions, jobs with flexible schedules may decrease gender discrimination in hiring, as they are accompanied by presumed lower indirect labour costs of mother workers (e.g., in view of anticipated absence owing to parental duties; see Anker 1997 ). In particular, jobs that allow for home-office work may reduce the organisational opportunity structure for discrimination in hiring (for a critical account, see Van Echtelt et al. 2009 ).
With regard to the company’s characteristics , previous research concerning the size of the company are inconclusive. On the one hand, Bygren and Kumlin ( 2005 ) find evidence that large work organisations tend to make more gender-neutral recruitments. On the other hand, Baert et al. ( 2018 ), in an experimental study of gender discrimination in hiring for higher skilled occupations in Belgium, did not find any evidence of a link between firm size and discrimination. However, their study does provide suggestive evidence that discrimination may be lower in public and non-profit organisations than in for-profit organisations. As far as the firms’ economic performance is concerned, they find no association between the economic well-being of the company and recruiters’ tendency to discriminate. One could, however, assume that recruiters are more risk averse and therefore more prone to statistically discriminating when they hire under difficult economic conditions (Baert et al. 2015 ). Taken together, we can formulate the more general assumption, that gender discrimination varies with recruiter, vacancy- and company-specific opportunity structures (H2).
3.2 Occupation and Country Characteristics
Further opportunity structures for gender discrimination in hiring can be assumed to exist on more aggregate levels, such as the occupational field and the country. With regard to the occupational field, diverse research has shown that the degree of gender discrimination and the mechanisms behind it are heterogeneous and vary with occupational characteristics (Baert 2018 ; Petit 2007 ). A fundamental characteristic underlying gender discrimination is the gender ratio within an occupation . Discrimination against candidates of one gender has been found to occur in occupations dominated by the other gender (for women: Kübler et al. 2018 ; for men: Glick et al. 1988 ; Weichselbaumer 2004 ). The proportion of women in the occupational field of mechanics is smaller than that for IT professionals (see below), suggesting a higher potential for gender discrimination in the former field.
Glick et al. ( 1988 ), in correspondence with Goffman’s ( 1977 ) account of institutional genderism, have argued that gender discrimination is influenced by occupational stereotypes within each occupational field to different degrees. Indeed, Gorman’s ( 2005 ) findings show that when selection criteria include a greater number of gender stereotypes, candidates of the opposite gender constitute a smaller proportion of new hires. With respect to more objective occupational job requirements such as heavy lifting and/or other physical efforts, the work of mechanics may be associated with higher physical (muscular) strength than IT work, disqualifying women from the former in the eyes of recruiters more than from the latter (Anker 1997 ). As Imdorf ( 2013 ) shows, physical strength can still be a criterion for hiring apprentices in mechanics in Switzerland. IT, in contrast, includes a variety of quite diverse jobs, with some of them requiring considerable soft and communicative skills, which are in line with female stereotypes (Barone 2011 ). Moreover, work in IT can—at least partly—be carried out at home. IT work may thus offer greater flexibility both with regard to the time schedule and place than mechanics. Taken together, the different opportunity for flexibility, as well as the different physical and social skill requirements and respective gender stereotypes in the two occupational fields, may result in a higher tendency of risk-averse recruiters to discriminate against women in the field of mechanics than in the IT sector. Overall, we can thus assume gender discrimination in hiring to be stronger in the occupational field of mechanics than in the field of information technology (H3).
We expect H3 to be valid in all four countries under investigation. In Norway, horizontal gender segregation has been stable for the last two decades (Barth et al. 2014 ). Both IT (74% males), and even more so mechanics (93% males), are solidly male-dominated occupations (NAV Statistics 2019 ). In Switzerland, too, the female work force in IT (22%) is larger than that among mechanics (13%, BfS 2019 ). In Bulgaria, female representation in the field of mechanics (25%) and IT (27%; Eurostat 2018 ; National Statistical Institute 2019 ) is somewhat higher. However, women’s disadvantage with regard to IT positions becomes substantial after they have become mothers (Stoilova 2008 ). In the Greek labour market, vertical and horizontal gender segregation have only slightly declined (Dermanakis 2005 ; Kantaraki et al. 2008 ). In IT, women account for about 11% of the workforce (European Commission 2019 ); in the field of mechanics, the share is between 8 and 11% (ELSTAT 2019 ). These figures are broadly in line with the estimated percentages of female workers in both occupational fields yielded in an expert survey in the four countries, conducted during the NEGOTIATE project, which we introduce below.
Nevertheless, the level of occupational gender discrimination in hiring is likely to vary among the four countries, which constitute different configurations of national characteristics such as the economy, educational and gender equality policies, and gender norms. These contextual characteristics, too, may constitute opportunity structures for gender discrimination in hiring. Economic conditions affect recruiters’ hiring behaviour through labour market tightness (i.e. a shortage of qualified candidates) and youth unemployment. Both less (Baert et al. 2015 ) and more (Mooi-Reci and Ganzeboom 2015 ) gender discrimination have been reported in occupations characterised by tight labour market conditions. With regard to the educational system , training for IT jobs predominantly takes place in institutions of higher education, whereas training for mechanics is usually organised at the upper-secondary level of vocational education and training (VET). Either VET can be solely school based (as in Greece or Bulgaria) or it also takes place in companies (dual VET, as in Norway and Switzerland). Where training is more employer oriented and standardised, VET certificates signal occupational skills more reliably. Hence, recruiters’ assumptions about applicants’ skills should be less based on gender stereotypes in the latter two than in the former two countries.
Policies promoting gender equality, such as antidiscrimination policies, may prevent discriminatory behaviour. Implementing antidiscrimination policies (e.g., through ombudspersons) reduces opportunities for discriminatory behaviour in hiring and facilitates the documentation and processing of such behaviour (Teigen 1999 ; Peterson and Saporta 2004 ). These measures are particularly well-developed in Scandinavian countries, which in our case concerns Norway. Countries that promote gender equality by means of paid parental leave and publicly financed childcare infrastructure have a higher female and maternal labour market participation rate (Leitner 2003 ). These policies often also reflect gender norms . Where mothers are more integrated into the labour market, such as in Norway and Bulgaria, it can be argued that employers are less risk-averse to hiring women.
Overall, we can thus formulate the tentative assumption that gender discrimination against female applicants may be stronger in Bulgaria and Greece than in Switzerland and especially in Norway. However, as educational and social policies as well as economic and cultural contexts might be intertwined, four countries are far too few to test any cross-national hypotheses properly.
4 Data and Method
4.1 data and sample.
Our data were obtained in the context of the Horizon 2020-funded project “NEGOTIATE—Overcoming early job-insecurity in Europe” Footnote 1 . Within this project, a multinational online recruiter survey was conducted in Bulgaria, Greece, Norway and Switzerland across five occupational fields (mechanics, finance, nursing, gastronomy and information technology). Footnote 2 The study participants (recruiters) were sampled through real-world vacancies, which affords the study a very high external validity. To ensure that our fictional candidates adequately fit into the profiles of the vacancies across countries, the latter were sampled according to job titles based on selected four-digit ISCO-08 codes. Footnote 3 During a period of six weeks in spring 2016, open jobs were sampled in the four countries through online job portals, official labour office websites, and companies’ own websites. The recruiters in charge of the 12,147 vacancies sampled were contacted. In total, 2,885 recruiters have participated in the survey (NEGOTIATE 2020 ).
4.2 Study Design
The survey consisted of multiple parts. On the one hand, a vignette study (FSE) was implemented. Each recruiter was presented with ten hypothetical CVs of skilled young workers (eight in the case of Greece), and asked to rate them according to the likelihood of being considered for the sampled vacancy. The vignette was displayed graphically, with the CV built into a timeline (for an example in German, see Fig. A.1 in the Online Appendix). A total of 20,634 vignettes were assessed by recruiters. On the other hand, in a standard survey mode, recruiters were asked about characteristics with regard to the vacancy, the company and themselves.
The vignettes used in the study, representing the fictitious CVs of job candidates, were designed to vary in four dimensions: unemployment duration and timing of unemployment (seven categories), educational and professional trajectories (nine categories), gender (male, female), and a dichotomous nation-specific experimental variable. Nationality (in each country: being a native and belonging to the ethnic majority) and time since leaving formal education (a total of five years, during all of which the candidate was available to the labour market) were held constant in the experiment. This yields a vignette universe of 252 (7*9*2*2) possible combinations. Implausible combinations of unemployment histories and career trajectories were excluded from the vignette universe, as they led to irritation, as two pre-tests have shown. Hence, the design is not perfectly orthogonal (for a correlation matrix see Table A.4 in the Online Appendix).
Based on response rate estimates derived from the pre-tests, a D-efficient sample (Auspurg and Hinz 2015 ) of 162 (CH and NO) and 90 (BG and GR) different vignettes was drawn and allocated, for each country separately, to 10 (BG, GR) and 18 (CH, NO) vignette decks with eight (GR) and ten (BG, CH, NO) vignettes each. Footnote 4 Randomisation of the vignettes into decks was conducted in order to maximise D‑efficiency (for a calculation of D‑efficiency for the various options, see Table A.5. in the Online Appendix). The respective vignette decks were allocated to the recruiters through randomisation (NEGOTIATE 2020 ).
4.3 Dependent Variable and Analytical Strategy
Recruiters were asked to rate each of the hypothetical CVs. The exact wording was “How likely is it that you would consider a person with the resumé displayed above for the advertised job?” The answering scale ranges from 0 (“practically zero”) to 10 (“excellent”). It was further transformed by adding “1”, and then logged in order to remove skewness. Footnote 5 In the following, we rely on these transformed ratings (ranging from 0 to 2.4) as our dependent variable. We apply multilevel linear models (Maas and Hox 2005 ; Rabe-Hesketh and Skrondal 2012 ) with vignettes (first-level units) nested in vacancies (second-level units). Because the vacancies were sampled for each of the five occupations separately in the NEGOTIATE project, the data set technically consists of 20 separate vignette experiments. We thus decided to estimate eight separate models: one for each country (BG, CH, GR, NO) and occupational field (mechanics, IT).
To test our hypotheses, we conduct our analyses in two steps. In a first step, we assess “raw” gender discrimination as the coefficient of vignette gender on recruitment chances (controlling only for candidate characteristics, Table 1 ). By comparing coefficients across models, we test for differences in gender discrimination between countries and occupations (see Table A.6 in the Online Appendix). In a second step, we include interaction terms between vignette gender and the various opportunity structures at the recruiter, vacancy and company levels (see Table 2 ). This measure allows us to figure out whether recruiters’ “tastes” or preferences are generally applicable or whether they are held against male or female candidates in specific contexts. As interaction terms are not straightforward to interpret, we present them as average marginal effects (AMEs) (Figs. 2 , 3 , 4 , 5 and 6 ). For the sake of brevity, we limit their graphical illustration to significant interaction terms.
4.4 Operationalisation of the Independent Variables
The focus of this study is on the effect of the vignette’s gender. Five further variables were controlled. They include a vignette’s unemployment duration (none, 10 months, 20 months) and educational level (secondary, upper secondary, tertiary). Moreover, we include three dummy variables that were constructed to measure the vertical and horizontal match between the vignette and the requirements of the vacancy. Vertical match was measured as a dummy variable (1: candidate’s educational level matches job requirements, 0: under- or over-qualified). Horizontal match was measured with two dummy variables representing two stages, namely (1) the fit of the candidate’s educational specialisation with the vacancy (1: obtained in the respective occupation) and (2) the fit of the candidate’s work experience (1: obtained in the respective occupational field). We also include an interaction term between these two dummy variables.
Our data are structured in a hierarchical way. Recruiters were approached through sampled real-life vacancies. The sample was restricted to one vacancy and recruiter per company. Hence, the levels of vacancy, recruiter and company overlap. We thus have a two-level structure (candidates nested in recruiters/vacancies/companies). Regarding recruiters’ “tastes”, we measure the candidate’s fit into the team as a hiring criterion (0: not important to 4: very important), recruiters’ unemployment resentment (no, it depends, yes) as well as recruiters’ gender (1: male). We further include variables that comprise specific features of the vacancy such as whether the vacancy was already filled (1: yes), the importance of filling the vacancy (1: not important to 4: very important), the recruiter’s perceived difficulty in filling the vacancy (1: very easy to 4: very difficult), the necessity of work experience for the vacancy (many years, some years, not necessary), the status of the advertised job in the company hierarchy (trainee, employee, executive function), the wage of the advertised position (measured in country- and occupation-specific quartiles), and whether the advertised position is full time or part time (1: part time). Finally, we include variables that describe the organisational structure of the company, including firm size and economic performance. For the latter, recruiters were asked to compare their company’s current economic performance with the economic performance in the previous year (answering categories: improved, stable, worsened, non-profit oriented). To avoid methodological effects, we include two dummy variables as controls that pertain to the order of appearance (primacy, 1: first or second vignette shown) and its deliberate selection into the deck (“fixed vignette”, which was included to exhibit a good fit with the real-world vacancy).
5.1 Gender Discrimination
Figure 1 illustrates the AME for vignette gender (female) on hiring chances across the four countries and two occupations (for coefficients see Table 1 ). As the bars indicate, female candidates have a lower likelihood of being hired in three countries. In Bulgaria, female candidates for a vacancy in mechanics are rated about 43% (b = −0.432 *** ) Footnote 6 less positively than their otherwise comparable (ceteris paribus) male competitors. In Greece, female candidates for a vacancy in mechanics are rated about 18% less positively, in Switzerland and Bulgaria, female candidates for vacancies in IT are rated about 5% less positively. No gender discrimination in recruiters’ ratings can be observed in Norway.
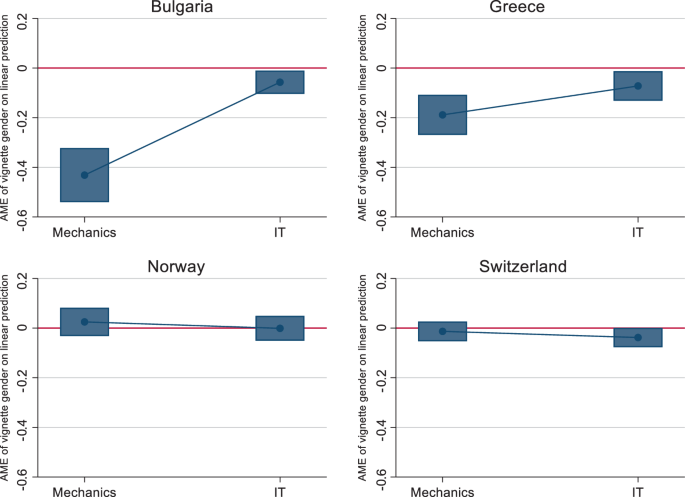
Gender discrimination by occupational field and country. Source: Negotiate Employer Survey (NEGOTIATE 2020 ). Multilevel linear regressions, with control variables (Table 1 ). Average marginal effect (AME) of vignette gender (female), bars represent 95% confidence intervals
The coefficients for vignette gender in mechanics significantly differ between the countries, with the exception of the comparison between Switzerland and Norway. The coefficients for the occupational field of IT do not significantly differ between the countries (see Table A.6 for respective test statistics). Our data thus only partly support our first hypothesis (H1). Rather, it seems that (the extent of) gender discrimination depends on national and occupational contexts.
The results also only partly confirm our assumption that the extent of gender discrimination is greater in mechanics than in IT (H3). Although our findings indicate stronger discrimination in mechanics versus IT for both Greece and Bulgaria, the difference between the coefficients is only significant in Bulgaria. Finally, the effects of the control variables (see Table 1 ) highlight the crucial relevance of vertical and horizontal match in all four countries, a finding in line with previous research (Shi et al. 2018 ).
5.2 Gender-Relevant Effects of Opportunity Structures
This section explores how higher level factors influence gendered hiring decisions. Based on the ideas of Reskin ( 2003 ) and Peterson and Saporta ( 2004 ), we assume that gender discrimination is contingent upon opportunity structures (H2). We test this second hypothesis by estimating interaction terms between the vignette’s gender and such factors at the recruiter, job and company levels. For ease of interpretability, the results are depicted as average marginal effects (AMEs, Figs. 2 , 3 , 4 , 5 and 6 ), limited to the significant effects. The AMEs were obtained under the control of other vignette characteristics, but not controlling for the respective other opportunity structures. To check the robustness of our results, all gender-specific mechanisms were also estimated simultaneously in one model (see Table 2 ).
Regarding the need for work experience (Fig. 2 ), we find evidence of female candidates being more critically scrutinised than male candidates if (some) work experience is required (as, for instance, in Switzerland in IT). Here, recruiters may use work experience as a gendered sorting mechanism (Ranson and Reeves 1996 ). In contrast, in Bulgaria (both occupations) and Greece (mechanics), women are discriminated against if no previous work experience is required. As VET is school based in these countries, recruiters’ risk aversion may play a role. Applicants who need on-the-job training require employers to make a financial commitment, and (potential) motherhood could diminish the returns on these investments.
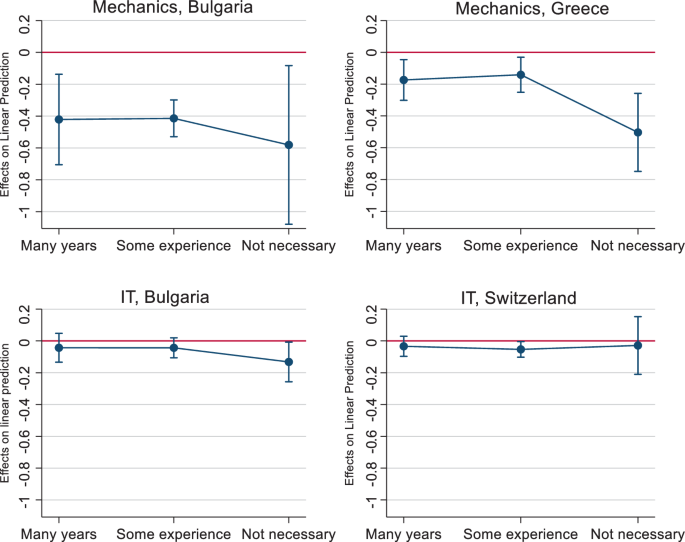
Gender discrimination over the need for job experience. Separate models for each occupation in each country. Average marginal effects with 95% confidence intervals for interaction effects between vignette gender and opportunity structure, controlling for candidate characteristics in Table 1 . Only figures for significant interaction terms are presented. For coefficients see Table A.3 in the Online-Appendix
Next, we turn to the position in the company’s hierarchy a successful applicant would occupy (Fig. 3 ). In many contexts, female candidates for trainee or employee positions receive lower ratings than their otherwise comparable male competitors (as for mechanics in Bulgaria and Greece, IT employees in Bulgaria and Switzerland and trainees in mechanics in Norway). What becomes clear, however, is that female candidates seem to “lose” their gender disadvantage in the hiring for executive positions—with the exception of IT in Greece. Qualities that are interpreted as typically feminine, such as communications skills, may be useful for ascending the ladder in a male-dominated professional environment such as IT (see also Major et al. 2007 ). This model is particularly striking in Norway, where, even when controlling for a position’s wages, female candidates appear to be actively promoted (see Table 2 ).
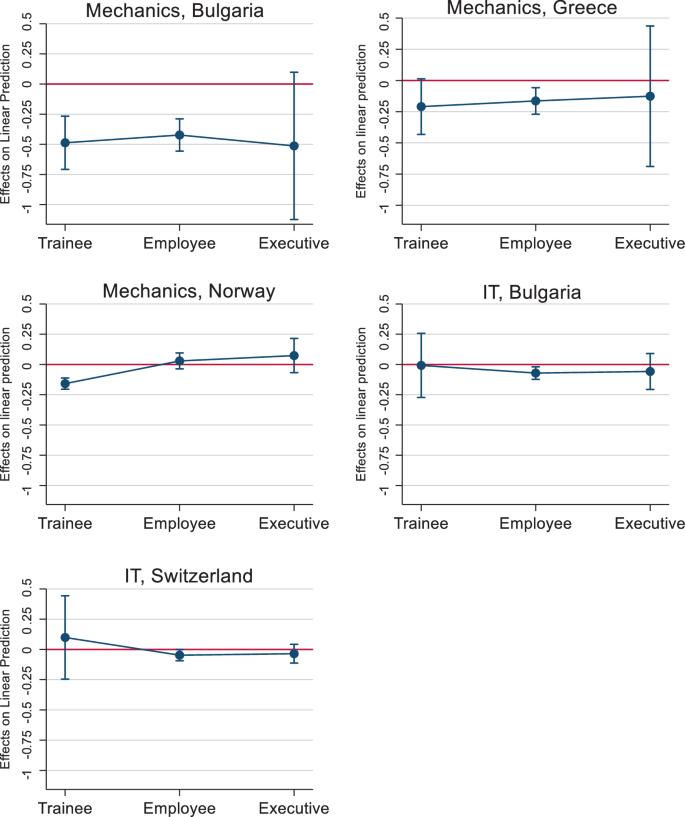
Gender discrimination according to job status. Separate models for each occupation in each country. Average marginal effects with 95% confidence intervals for interaction effects between vignette gender and opportunity structure, controlling for candidate characteristics in Table 1 . Only figures for significant interaction terms are presented. For coefficients, see Table A.3 in the Online Appendix
Regarding wages (Fig. 4 )—in line with our assumptions and the theoretical idea of risk aversion (Reskin and Branch McBrier 2000 )—we find evidence that female candidates may be discriminated against in higher paying jobs in IT in Switzerland. In Bulgaria, women are discriminated against across all wage groups in the field of mechanics. Another pattern runs against our expectations. Contrary to our assumption that lower-paid jobs (particularly in IT) would offer some flexibility and be preferentially given to women (and future mothers), we observe that recruiters hiring for such jobs in Greece and Bulgaria discriminate more strongly against female applicants than those hiring for higher-paid jobs. This result is, however, in line with Yavorsky’s ( 2019 ) finding that women are more likely to be discriminated against in manual jobs than in academic jobs.
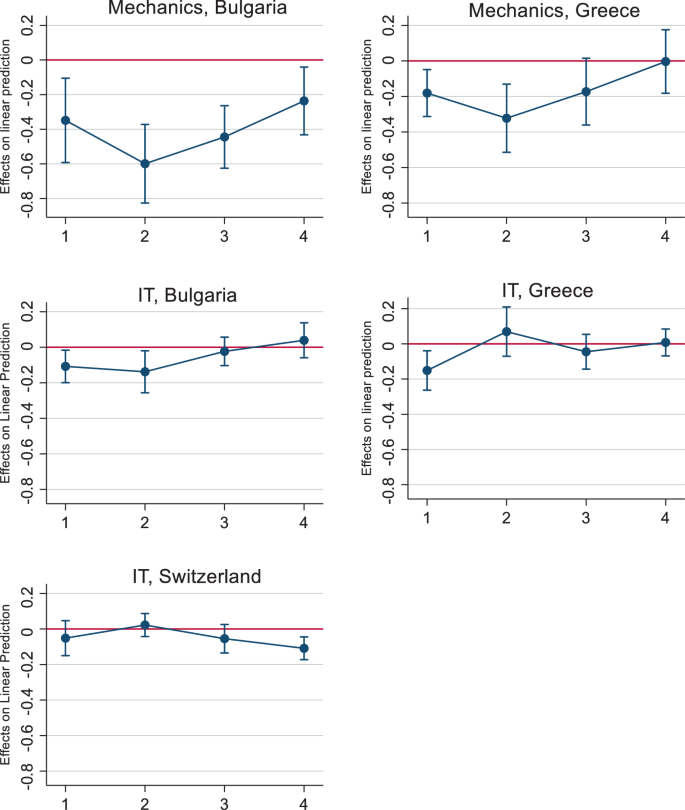
Gender discrimination according to wage quartiles of the vacancy. Separate models for each occupation in each country. Average marginal effects with 95% confidence intervals for interaction effects between vignette gender and opportunity structure, controlling for candidate characteristics in Table 1 . Only figures for significant interaction terms are presented.. For coefficients see Table A.3 in the Online Appendix
Figure 5 displays the effects of gendered recruitment decisions on full-time and part-time positions. According to our theoretical expectations, part-time positions would be more likely to be filled with female candidates. For the most part, however, part-time status does not seem to influence hiring decisions in favour of female candidates. Nevertheless, we do find evidence that gender discrimination is more likely in the hiring for full-time positions (as is the case for mechanics in Greece and Bulgaria and IT professionals in Bulgaria and Switzerland).
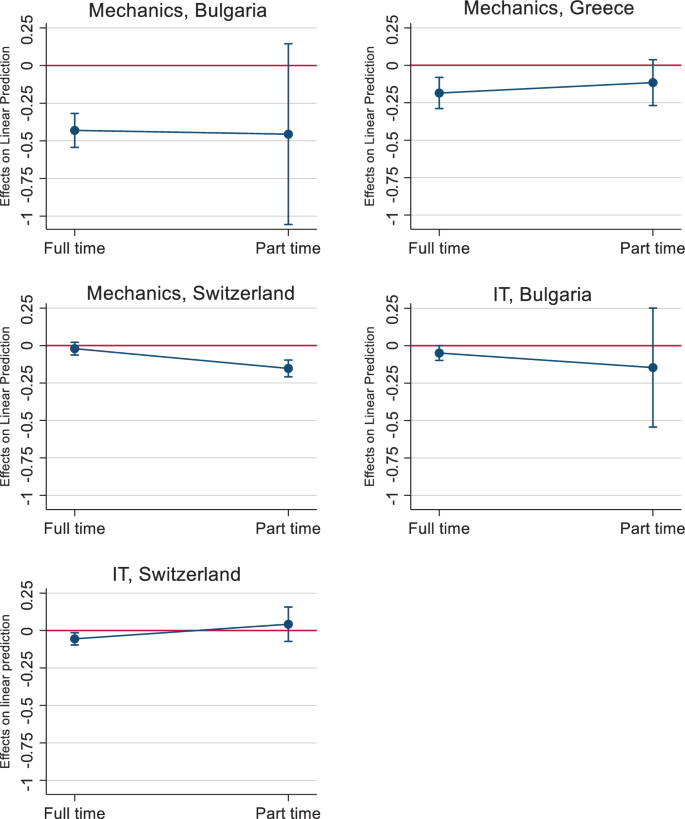
Gender discrimination according to full-time and part-time work. Separate models for each occupation in each country. Average marginal effects with 95% confidence intervals for interaction effects between vignette gender and opportunity structure, controlling for candidate characteristics in Table 1 . Only figures for significant interaction terms are presented. For coefficients see Table A.3 in the Online Appendix
With regard to company characteristics, we turn to the company’s economic performance (Fig. 6 ). In line with our expectations, we find that female applicants are discriminated against if the company’s economic performance has worsened (as is the case for IT professionals in Bulgaria and Norway and for mechanics in Bulgaria). However, we also find evidence for discrimination of female candidates in non-profit companies, as is the case for mechanics’ jobs in Norway and Greece. Finally, if the company’s economic performance has remained stable, in Greece and Bulgaria, female candidates are less likely to be hired for mechanics’ jobs, whereas in Norway they are more likely to be hired for the same jobs.
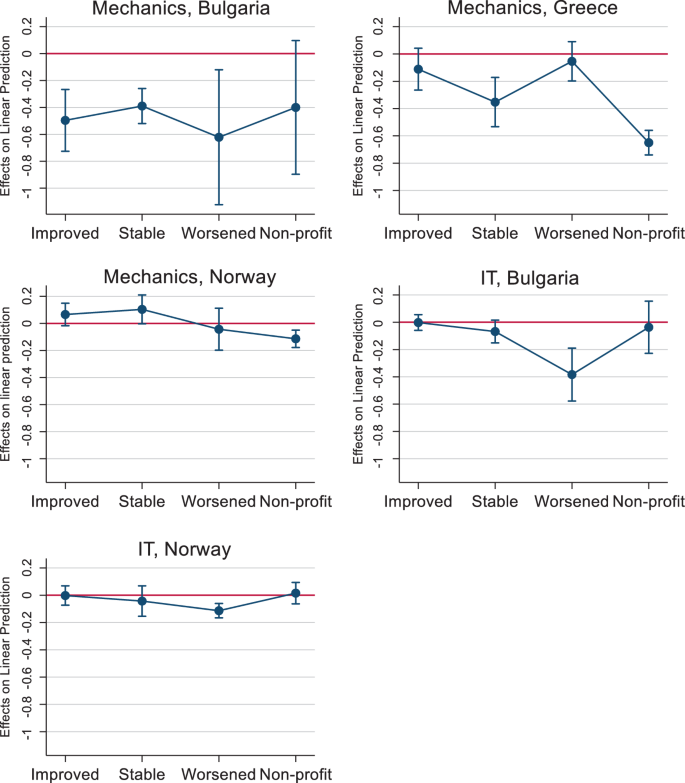
Gender discrimination according to economic performance of the company. Separate models for each occupation in each country. Average marginal effects with 95% confidence intervals for interaction effects between vignette gender and opportunity structure, controlling for candidate characteristics in Table 1 . Only figures for significant interaction terms are presented.. For coefficients see Table A.3 in the Online Appendix
In sum, our findings partly confirm our second hypothesis (H2), from which we assumed that the degree of gender discrimination in hiring is moderated by recruiter-, vacancy- and company-specific structures. Contrary to H2, we do not find evidence that recruiter characteristics such as recruiters’ gender or unemployment resentments moderate gender discrimination in hiring for skilled jobs, nor do we find conclusive evidence that either company size, the importance of or difficulty in filling the vacancy, or the organisational prerequisite of social fit, have an impact. We thus observe several, sometimes ambivalent, patterns.
6 Summary and Conclusion
Research has repeatedly documented women’s impeded access to STEM occupations and indicated gender discrimination in hiring for male-dominated occupations. This article highlights that gender discrimination in hiring for two male-dominated jobs varies between countries and occupations and depends on specific opportunity structures at the job and company levels. Applying one of the first internationally comparative factorial surveys in the field of hiring discrimination, our results strengthen Quillian et al.’s ( 2019 ) recent finding that patterns of discrimination vary across country contexts. Our results further support sociological claims that prejudices and/or stereotypes can only be upheld if organisational contexts and practices allow for it (Reskin 2003 ) or if there is an opportunity structure for discrimination (Peterson and Saporta 2004 ).
Overall, we find that female applicants have lower recruitment chances compared with their male counterparts, but this phenomenon is not equally pronounced in all contexts. As our results have shown, the degree of discrimination against female candidates not only varies between countries, but also between occupations within countries. The strongest gender discrimination was found in Bulgaria in the field of mechanics, followed by Greece in the field of mechanics, and Greece and Switzerland in the field of IT. No discriminatory behaviour against female candidates was found in Norway, which is in line with Birkelund et al. ( 2019 ).
With regard to country differences, our findings confirm the assumption that gender discrimination is higher in Bulgaria and Greece than in Switzerland and Norway. Owing to low case numbers at the country level, however, we cannot disentangle the specific relevance of different country-level factors. We can only assume that the absence of gender discrimination in Norway is due to its distinctive gender equity and anti-discrimination policies combined with employers’ trust in the educational signals of skilled workers. The absence of gender discrimination in the field of mechanics in Switzerland may be facilitated by its highly standardised dual-VET system, which prevents recruiters from using gender as an indication of occupational skills, whereas in IT, where training is for the most part organised at the tertiary level, gender stereotypes still seem to apply. In contrast to Switzerland, gender discrimination seems to be stronger in the field of mechanics than in information technology in Bulgaria and Greece. In those two country contexts, where the signals of vocational certificates are less reliable gender stereotypes can become salient in anticipating occupational skills. Recruiters may assume that the more flexible IT jobs are more accessible for skilled women, whereas jobs in mechanics tend to be full time, with little room for temporal and spatial flexibilisation in manufacturing companies. Supposing further gender stereotypes with regard to the physical and social skill requirements of the two occupations, recruiters may be more risk averse about female candidates when hiring mechanics than when hiring IT professionals.
We have further explored various mechanisms at the recruiter, vacancy and company levels that may mediate gender discrimination. Our analyses revealed a number of economic mechanisms (representing opportunity structures for gender discrimination at the levels of the vacancy and of the company), whose influence on employment chances depends on the gender of the applicant. These mechanisms apply to work experience required, job status, wages, full-time or part-time work, and the economic performance of the company, as well as its economic orientation (i.e. public versus private). Recruiters’ characteristics, on the other hand, have not shown such gender-specific effects. The direction and significance of these gender-specific opportunity structures remain multifaceted and sometimes ambivalent. Our findings indicate that such mechanisms have a different impact, or even take different directions, depending on the occupational and country context (for a summary, see Table 3 ).
Overall, our findings suggest that the mechanisms underlying gender discrimination might be multifaceted and highly contextual. Not only do they depend on opportunity structures and gender-specific occupational stereotypes or skill expectations, these mechanisms further vary substantially between countries and occupations. Future research should thus both theorise and measure these mechanisms in a differentiated way and consider that the salience of these mechanisms may vary on several contextual levels. The practical implication of our overall finding is that, to be both effective and economical, anti-gender discrimination policy must be target-orientated to occupational contexts where there is scope for discriminatory practices in hiring. As such, an in-depth understanding of the discriminatory mechanisms is exigent. The mechanisms of gender discrimination in the hiring for skilled jobs for which we have found evidence (Table 3 ) lend support to the model of statistical discrimination rather than to the model of taste-based discrimination. Even though we sometimes identify diverging patterns, a number of findings point to economic risk aversion by recruiters in hiring IT professionals and mechanics. For instance, when the hiring is related to certain direct costs (e.g. higher wages, worsening economic performance), or to indirect training-related costs and insecure returns (e.g. trainee positions, unskilled and low-wage jobs, particularly in countries with less established VET), female candidates are passed over for male candidates.
Our study has several limitations: it is confined to the analysis of two male-dominated occupations, and our results need further validation with regard to other STEM occupations. Moreover, the occupation- and country-specific mechanisms we have discussed might not hold for female-dominated or gender-neutral professions. We also need to be aware that most of the resumés in the vignette experiment showed a rather “bad fit” with the requirements of the sampled jobs. It would be preferable to apply the factorial survey methodology in the study of gender discrimination in hiring with less interference from competing research aims, and to use the remaining vignette dimensions to directly address theory-driven discrimination mechanisms. Finally, we still need a better understanding of how we can explain statistical gender discrimination effects with opportunity structures. Beside the replication of our occupational and international comparative results, several more ambivalent findings warrant greater scrutiny.
https://negotiate-research.no .
A scientific use file of the NEGOTIATE employer survey data is available through the Norwegian Centre for Research Data (NSD) at https://doi.org/10.18712/NSD-NSD2644-V1 .
The ISCO codes used were 7230 and 7233 for Mechanics, and 2511–2513, as well as 2521–2523 for IT professionals.
Although ten decks à ten vignettes were planned for Greece, only eight vignettes were shown in the survey due to technical implementation issues (for more information see NEGOTIATE 2020 ).
Given the experimental design, which is aimed at maximum variation, most vignettes in a deck exhibit only a poor fit with the advertised job. Thus, the distribution of the ratings on the original scale (0–10) is right-skewed.
Since the dependent variable was logged, it can be interpreted as the percentage change in the recruiter’s rating with a one-unit change, i.e. the difference between female and male applicants, in the independent variable.
Abraham, Martin, Katrin Auspurg, Sebastian Bähr, Corinna Frodermann, Stefanie Gundert, and Thomas Hinz. 2013. Unemployment and willingness to accept job offers: Results of a factorial survey experiment. Journal for Labour Market Research 46:283–305.
Google Scholar
Anker, Richard. 1997. Theories of occupational segregation by sex: An overview. International Labour Review 136:315–339.
Arrow, Kenneth. 1973. The Theory of Discrimination. In Discrimination in Labor Market, eds. Orley Ashenfelter and Albert Rees, 3–33. Princeton: Princeton University Press.
Auspurg, Katrin, and Thomas Hinz. 2015. Factorial Survey Experiments. Thousand Oaks: SAGE Publications.
Autor, David. 2008. The Economics of Discrimination I . Lecture Note. Massachusetts. MIT. 14.663.
Baert, Stijn. 2018. Hiring Discrimination: An Overview of (Almost) All Correspondence Experiments since 2005. In Audit Studies: Behind the Scenes with Theory, Method, and Nuance . Methodos Series (Methodological Prospects in the Social Sciences), Vol 14. Ed. Michael S. Gaddis. Cham: Springer.
Baert, Stijn, Bart Cockx, Niels Gheyle and Cora Vandamme. 2015. Is There Less Discrimination in Occupations Where Recruitment Is Difficult? Industrial and Labor Market Review 68:467–500.
Baert, Stijn, Ann-Sofie De Meyer, Yentl Moerman and Eddy Omey. 2018. Does size matter? Hiring discrimination and firm size. Journal of Manpower 39:550–566.
Barone, Carlo. 2011. Some Things Never Change: Gender Segregation in Higher Education across Eight Nations and Three Decades. Sociology of Education 84:157–176.
Barth, Erling, Inel Hardoy, Pal Schøne and Kjersti M. Østbakken. 2014. Hva betyr høy yrkesdeltakelse for kjønnssegregering? [What does high labour market participation mean for gender segregation?]. In Kjønnsdeling og etniske skiller på arbeidsmarkedet , eds. Liza Reisel and Mari Teigen. Oslo: Gyldendal Akademisk.
Becker, Gary S. 1957. The Economics of Discrimination . Chicago: The University of Chicago Press.
Van Belle, Eva, Valentina Di Stasio, Ralf Caers, Marijke De Couck and Stijn Baert. 2018. Why Are Employers Put Off by Long Spells of Unemployment? European Sociological Review 34:1–17.
BfS, Bundesamt für Statistik. 2019. Beschäftigte nach Wirtschaftsabteilungen und Geschlecht. https://www.bfs.admin.ch/bfs/de/home/statistiken/kataloge-datenbanken/tabellen.assetdetail.8167804.html (Accessed: 24 June 2019).
Bielby, William T., and James N. Baron. 1986. Men and Women at Work: Sex Segregation and Statistical Discrimination. American Journal of Sociology 91:759–799.
Birkelund, Gunn E., Avra Janz and Edvard Nergård Larsen. 2019. Do males experience hiring discrimination in female-dominated occupations? An overview of field experiments since 1996. GEMM Working Paper. Retrieved from: http://gemm2020.eu/wp-content/uploads/2018/12/Gender-Discrimination-a-summary-1.pdf (Accessed: 29 May 2019).
Blickenstaff, Jacob C. 2005. Women and science careers: Leaky pipeline or gender filter? Gender and Education 17: 369–86.
Bygren, Magnus, and Johanna Kumlin. 2005. Mechanisms of Organizational Sex Segregation: Organizational Characteristics and the Sex of Newly Recruited Employees. Work and Occupations 32:39–65.
Charles, Maria. 2003. Deciphering sex segregation: vertical and horizontal inequalities in ten national labour markets. Acta Sociologica 46:267–287.
Cole, Michael S., Hubert S. Field and William F. Giles. 2004. Interaction of Recruiter and Applicant Gender in Resume Evaluation: A Field Study. Sex Roles 51:597–608.
Dermanakis, Nikos E. 2005. The horizontal-occupational segregation in the Greek labour market. Research Centre for Gender Equality . Statistical Bulletin 3.
DiPrete, Thomas A., and Claudia Buchmann. 2013. The rise of women: The growing gender gap in education and what it means for American schools . New York: Russell Sage Foundation.
Van Echtelt, Patricia, Arie Glebbeek, Suzan Lewis and Siegwart Lindenberg. 2009. Post-Fordist Work: A Man’s World? Gender and Working Overtime in the Netherlands. Gender & Society 32:188–214.
ELSTAT, Hellenic Statistical Authority. 2019. Labour Force Survey —annual data. http://nsi.bg/en/content/15277/%D0%BC%D0%B5%D1%82%D0%B0%D0%B4%D0%B0%D0%BD%D0%BD%D0%B8/labour-force-survey-annual-data (Accessed: 9 July 2019).
England, Paula. 2010. The Gender Revolution Uneven and Stalled. Gender & Society 24:149–66.
European Commission. 2019. Women in Digital Scoreboard 2019—Greece. https://ec.europa.eu/digital-single-market/en/news/women-digital-scoreboard-2019-country-reports (Accessed: 23 June 2019)
Eurostat. 2018. Employed ICT specialists by sex , http://appsso.eurostat.ec.europa.eu/nui/show.do?dataset=isoc_sks_itsps&lang=en (Accessed: 18 June 2019).
Fernandez, Roberto M., and Marie Louise Mors. 2008. Competing for jobs: Labor queues and gender sorting in the hiring process. Social Science Research 37:1061–1080.
Glick, Peter, Cari Zion and Cynthia Nelson. 1988. What mediates sex discrimination in hiring decisions? Journal of Personality and Social Psychology 55:178–186.
Goffman, Erving. 1977. The arrangement between the sexes. Theory and Society 4:301–311.
Gorman, Elizabeth H. 2005. Gender Stereotypes, Same-Gender Preferences, and Organizational Variation in the Hiring of Women: Evidence from Law Firms. American Sociological Review 70:702–728.
Guryan, Jonathan, and Kerwin Kofi Charles. 2013. Taste-based or Statistical Discrimination: The Economics of Discrimination Returns to its Roots. The Economic Journal 123:417–432.
Hakim, Catherine. 1996. The sexual division of labour and women’s heterogeneity. British Journal of Sociology 47:178–188.
Herman, Clem, Suzanne Lewis and Anne Laure Humbert. 2013. Women Scientists and Engineers in European Companies: Putting Motherhood under the Microscope. Gender, Work and Organization 20:467–479.
Imdorf, Christian, 2013. Lorsque les entreprises formatrices sélectionnent en fonction du genre. Le recrutement des apprenti(e)s dans le secteur de la réparation automobile en Suisse. Revue française de pédagogie 183: 59–70.
Imdorf, Christian, 2017. Understanding discrimination in hiring apprentices: How training companies use ethnicity to avoid organisational trouble. Journal of Vocational Education and Training 69:405–423.
Imdorf, Christian, Lulu P. Shi, Stefan Sacchi, Robin Samuel, Christer Hyggen, Rumiana Stoilova, Gabriela Yordanova, Pepka Boyadjieva, Petya Illieva-Trichkova, Dimitris Parsanaglou and Aggeliki Yfanti. 2019. Scars of early job insecurity across Europe: Insights from a multi-country employer study. In Youth Unemployment and Job Insecurity in Europe: Problems, Risk Factors and Policies , eds. Björn Hvinden, Christer Hyggen, Mi Ah Schøyen and Tomáš Sirovátka, 93–116. Cheltenham: Edward Elgar.
Kantaraki, Maroula, Maria Pagkaki and Evgenia Stamatelopoulou. 2008. Gender occupational segregation (vertical and horizontal): Discrimination and Inequality against women in education . Athens: Research Centre for Gender Equality.
Keuschnigg, Marc, and Tobias Wolbring. 2016. The Use of Field Experiments to Study Mechanisms of Discrimination. Analyse & Kritik 1:179–201.
Kricheli-Katz, Tamar. 2019. Us versus Them: The Responses of Managers to the Feminization of High-Status Occupations. Socius 5:1–15.
Kübler, Dorothea, Julia Schmid and Roland Stüber. 2018. Gender Discrimination in Hiring Across Occupations: A Nationally Representative Vignette Study. Labor Economics 55:215–229.
Leitner, Sigrid. 2003. Varieties of familism: The caring function of family in comparative perspective. European Societies 5:353–375.
Leuze, Kathrin, and Susanne Strauß. 2016. Why do occupations dominated by women pay less? How ‘female-typical’ work tasks and workingtime arrangements affect the gender wage gap among higher education graduates. Work, employment and society 30:802–820.
Levanon, Asaf, Paula England and Paul Allison. 2009. Occupational Feminization and Pay: Assessing Causal Dynamics Using 1950–2000 U.S. Census Data. Social Forces 88:865–892.
Maas, Cora J. M., and Joop J. Hox. 2005. Sufficient Sample Sizes for Multilevel Modeling. Methodology 1:86–92.
Major, Debra, Donald D. Davies, Janis Sanchez, Heather J. Downey and Lisa M. Germano. 2007. Myths and realities in the IT workplace: Gender differences and similarities in climate perceptions. In Women and Minorities in Science, Technology, Engineering and Mathematics. Upping the Numbers , eds. Ronald J. Burke and Mary C. Mathis, 71–90. Cheltenham: Edward Elgar.
Mastekaasa, Arne, and Jens-Christian Smeby. 2008. Educational choice and persistence in male- and female-dominated fields. Higher Education 55:189–202. https://doi.org/10.1007/s10734-006-9042-4 .
Article Google Scholar
Mooi-Reci, Irma, and Harry B. Ganzeboom. 2015. Unemployment scarring by gender: Human capital depreciation or stigmatization? Longitudinal evidence from the Netherlands, 1980–2000. Social Science Research 52:642–658.
National Statistical Institute, Republic of Bulgaria. 2019. Labour Force Survey. Persons employed 15+ (one-digit groups of individual occupations, occupational status, sex). http://www.statistics.gr/en/statistics/-/publication/SJO01/- (Accessed: 9 July 2019).
NAV Statistics 2019. Arbeidsgiver- og arbeidstakerregisteret [Register of employers and employees in Norway]. Utdanning 2014: Likestilling i norsk arbeidsliv [Gender equality in Norway]. https://utdanning.no/likestilling (Accessed 23. June 2019).
NEGOTIATE (2020). NEGOTIATE Employer Survey. Scientific Use File. Data Documentation (2nd version). Oslo: OsloMet.
Neumark, David, Roy J. Bank and Kyle D. Van Nort, 1996. Sex Discrimination in Restaurant Hiring: An Audit Study. The Quarterly Journal of Economics 111:915–941.
Ochsenfeld, Fabian. 2014. Why Do Women’s Fields of Study Pay Less? A Test of Devaluation, Human Capital, and Gender Role Theory. European Sociological Review 30:536–548.
Pedulla, David S. 2016. Penalized or Protected? Gender and the Consequences of Nonstandard and Mismatched Employment Histories. American Sociological Review 81:262–289.
Petersen, Trond, and Ishak Saporta. 2004. The Opportunity Structure for Discrimination. American Journal of Sociology 109:852–901.
Petit, Pascale. 2007. The Effects of Age and Family Constraints on Gender Hiring Discrimination: A Field Experiment in the French Financial Sector. Labour Economics 14:371–391.
Phelps, Edmund S. 1972. The Statistical Theory of Racism and Sexism. The American Economic Review 62:659–661.
Quillian, Lincoln, Anthony Heath, Devah Pager, Arnfinn H. Midtbøen, Fenella Fleischmann and Ole Hexel. 2019. Do Some Countries Discriminate More than Others? Evidence from 97 Field Experiments of Racial Discrimination in Hiring. Sociological Science 6:467–496.
Rabe-Hesketh, Sophia, and Anders Skrondal. 2012. Longitudinal and Multilevel Modelling using Stata, Volume I: Continuous Responses. College Station: Stata Press.
Ranson, Gillian, and William Joseph Reeves. 1996. Gender, Earnings and Proportions of Women. Lessons from a High-Tech Occupation. Gender & Society 10 :168–184.
Reskin, Barbara F. 2003. Including Mechanisms in Our Models of Ascriptive Inequality. American Sociological Review 68:1–21.
Reskin, Barbara F., and Debra Branch McBrier. 2000. Why Not Ascription? Organizations’ Employment of Male and Female Managers. American Sociological Review 65:210–233.
Riach, Peter A., and Judith Rich. 2006. An Experimental Investigation of Sexual Discrimination in Hiring in the English Labor Market. The B.E. Journal of Economic Analysis & Policy 6. doi:10.2202/1538-0637.1416.
Shi, Lulu P., Christian Imdorf, Robin Samuel and Stefan Sacchi. 2018. How Unemployment Scarring Affects Skilled Young Workers: Evidence from a Factorial Survey of Swiss Recruiters. Journal for Labour Market Research 52:7. https://doi.org/10.1186/s12651-018-0239-7 .
Shih, Johanna. 2002. ‘... Yeah, I Could Hire This One, But I Know It’s Gonna Be a Problem’: How Race, Nativity and Gender Affect Employers’ Perceptions of the Manageability of Job Seekers. Ethnic and Racial Studies 25 :99–119.
Stoilova, Rumiana. 2008. Impact of Gender on the Occupational Group of Programmers in Bulgaria. Sociological Problems Special Issue “Changes of work and the Knowledge–based society—the realities in South-Eastern Europe”: 94–117.
Teigen, Mari. 1999. Documenting Discrimination: A Study of Recruitment Cases Brought to the Norwegian Gender Equality Ombud. Gender, Work and Organization 6:91–105.
Weichselbaumer, Doris. 2004. Is it sex or personality? The impact of sex stereotypes on discrimination in applicant selection. Eastern Economic Journal 30:159–186.
Yavorsky, Jill E. 2019. Uneven Patterns of Inequality: An Audit Analysis of Hiring-Related Practices by Gendered and Classed Contexts. Social Forces , online first: doi: 10.1093/sf/soy123.
Download references
Acknowledgements
The authors would like to thank the three anonymous reviewers, the guest editors, as well as Robin Samuel and Tamara Gutfleisch for helpful comments on earlier versions of this manuscript.
This study was partly funded by the Horizon 2020 project “Negotiating early job-insecurity and labour market exclusion in Europe–NEGOTIATE” (Horizon 2020, Societal Challenge 6, H2020–YOUNG-SOCIETY-2014, YOUNG-1-2014).
Open Access funding provided by Projekt DEAL.
Author information
Authors and affiliations.
Department of History, Sociology, Empirical Educational Research and Sport Science, University of Konstanz, Universitätsstr. 10, 78464, Konstanz, Germany
Ariane Bertogg
Institute of Sociology, Leibniz University Hannover, Schneiderberg 50, 30167, Hannover, Germany
Christian Imdorf
Norwegian Social Research, Oslo Metropolitan University, Stensberggata 26, 0170, Oslo, Norway
Christer Hyggen
Department of Social Policy, Panteon University of Social and Political Science, 136 Syggrou Ave., 17671, Athens, Greece
Dimitris Parsanoglou
Institute of Philosophy and Sociology, Bulgarian Academy of Sciences, Moskovska 13A, 1000, Sofia, Bulgaria
Rumiana Stoilova
You can also search for this author in PubMed Google Scholar
Corresponding author
Correspondence to Ariane Bertogg .
Caption Electronic Supplementary Material
11577_2020_671_moesm1_esm.docx.
The electronic supplementary materials contain figures and tables with further data description of the sample and of the design of the Factorial Survey Experiment as well as the models that serve as a basis for Figures 2 to 6.
Rights and permissions
Open Access This article is licensed under a Creative Commons Attribution 4.0 International License, which permits use, sharing, adaptation, distribution and reproduction in any medium or format, as long as you give appropriate credit to the original author(s) and the source, provide a link to the Creative Commons licence, and indicate if changes were made. The images or other third party material in this article are included in the article’s Creative Commons licence, unless indicated otherwise in a credit line to the material. If material is not included in the article’s Creative Commons licence and your intended use is not permitted by statutory regulation or exceeds the permitted use, you will need to obtain permission directly from the copyright holder. To view a copy of this licence, visit http://creativecommons.org/licenses/by/4.0/ .
Reprints and permissions
About this article
Bertogg, A., Imdorf, C., Hyggen, C. et al. Gender Discrimination in the Hiring of Skilled Professionals in Two Male-Dominated Occupational Fields: A Factorial Survey Experiment with Real-World Vacancies and Recruiters in Four European Countries. Köln Z Soziol 72 (Suppl 1), 261–289 (2020). https://doi.org/10.1007/s11577-020-00671-6
Download citation
Published : 01 July 2020
Issue Date : September 2020
DOI : https://doi.org/10.1007/s11577-020-00671-6
Share this article
Anyone you share the following link with will be able to read this content:
Sorry, a shareable link is not currently available for this article.
Provided by the Springer Nature SharedIt content-sharing initiative
- Vignette Study
- Comparative study
Schlüsselwörter
- Rekrutierung
- Stellenausschreibungen
- Faktorieller Survey
- Internationaler Vergleich
- Find a journal
- Publish with us
- Track your research
Our privacy statement has changed. Changes effective July 1, 2024.
Why Amazon’s Automated Hiring Tool Discriminated Against Women
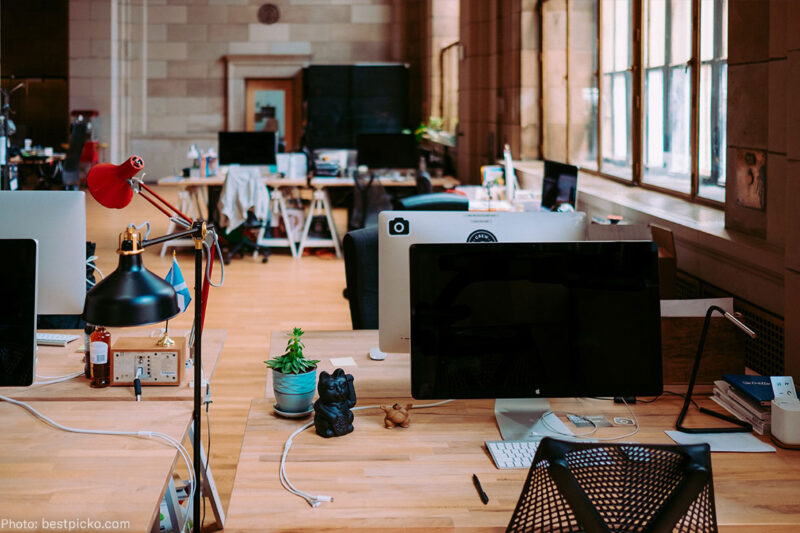
In 2014, a team of engineers at Amazon began working on a project to automate hiring at their company. Their task was to build an algorithm that could review resumes and determine which applicants Amazon should bring on board. But, according to a Reuters report this week, the project was canned just a year later, when it became clear that the tool systematically discriminated against women applying for technical jobs, such as software engineer positions.
It shouldn’t surprise us at all that the tool developed this kind of bias. The existing pool of Amazon software engineers is overwhelmingly male, and the new software was fed data about those engineers’ resumes. If you simply ask software to discover other resumes that look like the resumes in a “training” data set, reproducing the demographics of the existing workforce is virtually guaranteed.
In the case of the Amazon project, there were a few ways this happened. For example, the tool disadvantaged candidates who went to certain women’s colleges presumably not attended by many existing Amazon engineers. It similarly downgraded resumes that included the word “women’s” — as in “women’s rugby team.” And it privileged resumes with the kinds of verbs that men tend to use, like “executed” and “captured.”
Fortunately, Amazon stopped using the software program when it became clear the problem wasn’t going to go away despite programmers’ efforts to fix it. But recruiting tools that are likely similarly flawed are being used by hundreds of companies large and small, and their use is spreading.
HOW ARTIFICIAL INTELLIGENCE IS CHANGING THE WORKPLACE
There are many different models out there. Some machine learning programs — which learn how to complete a task based on the data they’re fed — scan resume text, while others analyze video interviews or performance on a game of some kind. Regardless, all such tools used for hiring measure success by looking for candidates who are in some way like a group of people (usually, current employees) designated as qualified or desirable by a human . As a result, these tools are not eliminating human bias — they are merely laundering it through software.
And it’s not just gender discrimination we should be concerned about. Think about all the ways in which looking at resume features might similarly cluster candidates by race: zip code, membership in a Black student union or a Latino professional association, or languages spoken. With video analysis, patterns of speech and eye contact have cultural components that can similarly lead to the exclusion of people from particular ethnic or racial groups. The same goes for certain physical or psychological disabilities.
We’ve seen these types of problems with artificial intelligence in many other contexts . For example, when we used Amazon’s facial recognition tool to compare members of Congress against a database of mugshots, we got 28 incorrect matches — and the rate for false matches was higher for members of color. This is due, in part, to the fact that the mugshot database itself had a disproportionately high number of people of color because of racial biases in the criminal justice system.
These tools are not eliminating human bias — they are merely laundering it through software.
Algorithms that disproportionately weed out job candidates of a particular gender, race, or religion are illegal under Title VII, the federal law prohibiting discrimination in employment. And that’s true regardless of whether employers or toolmakers intended to discriminate — “disparate impact discrimination” is enough to make such practices illegal.
But it can be difficult to sue over disparate impact, particularly in “failure-to-hire” cases. Such lawsuits are very rare because it’s so hard for someone who never got an interview to identify the policy or practice that led to her rejection.
That’s why transparency around recruiting programs and other algorithms used by both companies and the government is so crucial. Many vendors who market these hiring tools claim that they test for bias and in fact are less biased than humans. But their software is proprietary, and there’s currently no way to verify their claims. In some cases, careful work by outside auditors may be able to uncover bias, but their research is thwarted by various obstacles. We’re challenging one such obstacle — a federal law that can criminalize testing of employment websites for discrimination.
But even this kind of outside research can’t give us the full picture. We need regulators to examine not only the software itself but also applicant pools and hiring outcomes for companies that deploy the software. The Equal Employment Opportunity Commission, the federal agency that enforces laws against job discrimination, has begun to explore the implications of algorithms for fair employment, and we urge the agency to do more. EEOC should issue guidance for employers considering using these tools, detailing their potential liability for biased outcomes and steps they can take to test for and prevent bias. It should also include questions about data-driven bias in all of its investigations.
Big-data algorithms will replicate and even magnify the biases that exist in society at large — unless they are designed and monitored very, very carefully. The right kind of oversight is required to make sure that happens.
Learn More About the Issues on This Page
- Women's Rights
- Women's Rights in the Workplace
- Privacy & Technology
Related Content
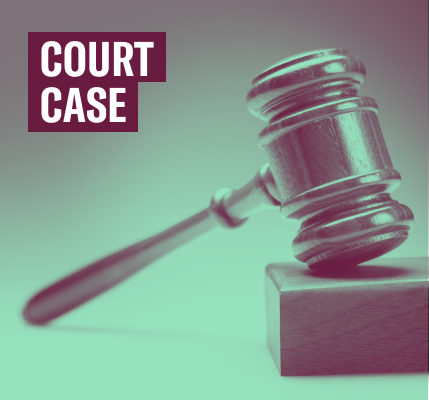
Gonzalez v. Ramirez et al.
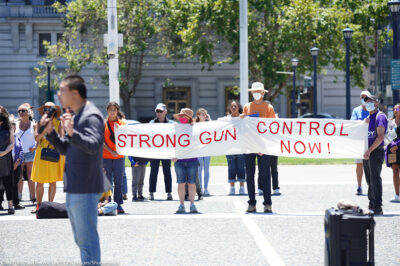
The Supreme Court's Gun Decision Is Not a Victory for Women
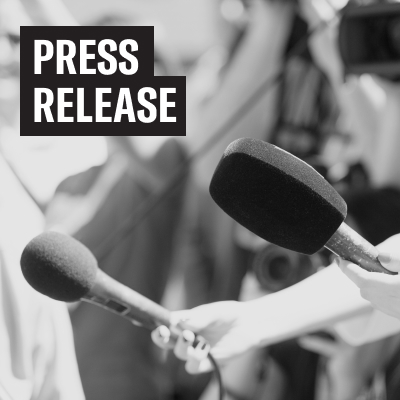
ACLU Comment on Supreme Court Decision Upholding Restrictions on Gun Possession by Individuals Subject to Domestic Violence Restraining Orders
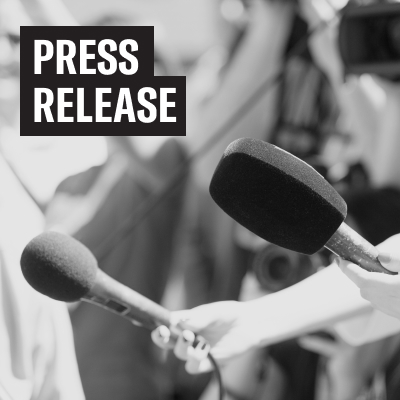
Civil Rights Groups Submit Brief in Support of Landmark Pregnant Workers Fairness Act
- English ES | Español 中文 | Chinese
Popular Pages
- Sustainability
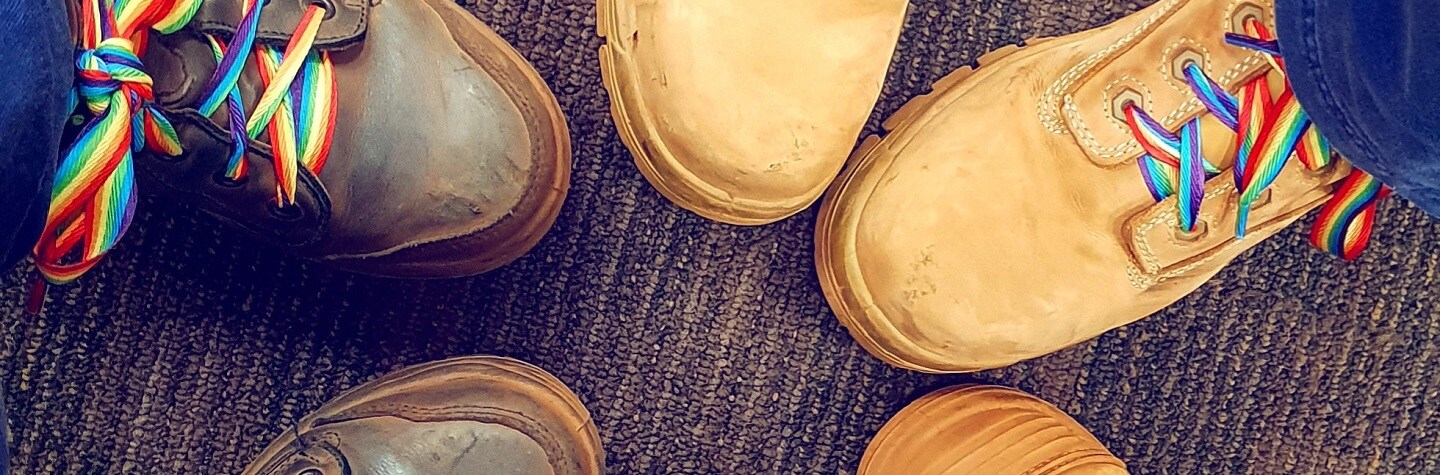
Transgender and gender diverse recruitment drive delivers results
Share this page.
Choose your network to share this page with. A new window will open.
06 September 2022
BHP’s first recruitment drive designed to engage with transgender and gender diverse talent has delivered results for the Maintenance and Engineering Centre of Excellence (MECoE) based in Santiago, Chile.
With the MECoE having achieved and sustained gender balance for employees since May 2021, the team wanted to widen their inclusion and diversity recruitment to a much broader talent pool. After connecting with Jasper 1 , BHP’s LGBT+ ally employee inclusion group, a position within the team was dedicated to the recruitment of a trans diverse person.
This was also an opportunity to address an important topic within the Minerals Americas region and highlight the challenges and opportunities that could be created with a more diverse and inclusive workforce.
As the first trans diverse recruitment campaign for BHP globally, the campaign provided opportunity to determine if any inherent biases against trans diverse people existed within existing recruitment processes.
Using BHP’s standard recruitment processes as a base, any perceived biases were identified and removed. A recruitment checklist was also developed to address any issues specific to trans diverse talent.
The result was an updated process and for MECoE, the appointment of a successful candidate. A simplified checklist was also developed for use by leaders during the onboarding process.
The MECoE has now been approached by other trans diverse people looking for opportunities to join the team.
1 Jasper’s aim is to drive a safe, inclusive and supportive work environment for everyone by providing advice on ways to reduce bias and ensure LGBT+ people are respected and valued irrespective of their sexual or gender identity.
Related Content
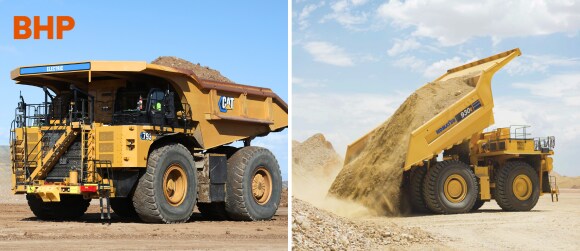
Decarbonisation Investor Presentation
BHP will be hosting an investor roundtable on decarbonisation to provide an update on our progress and plans to achieve our medium and long-term targets and goals.
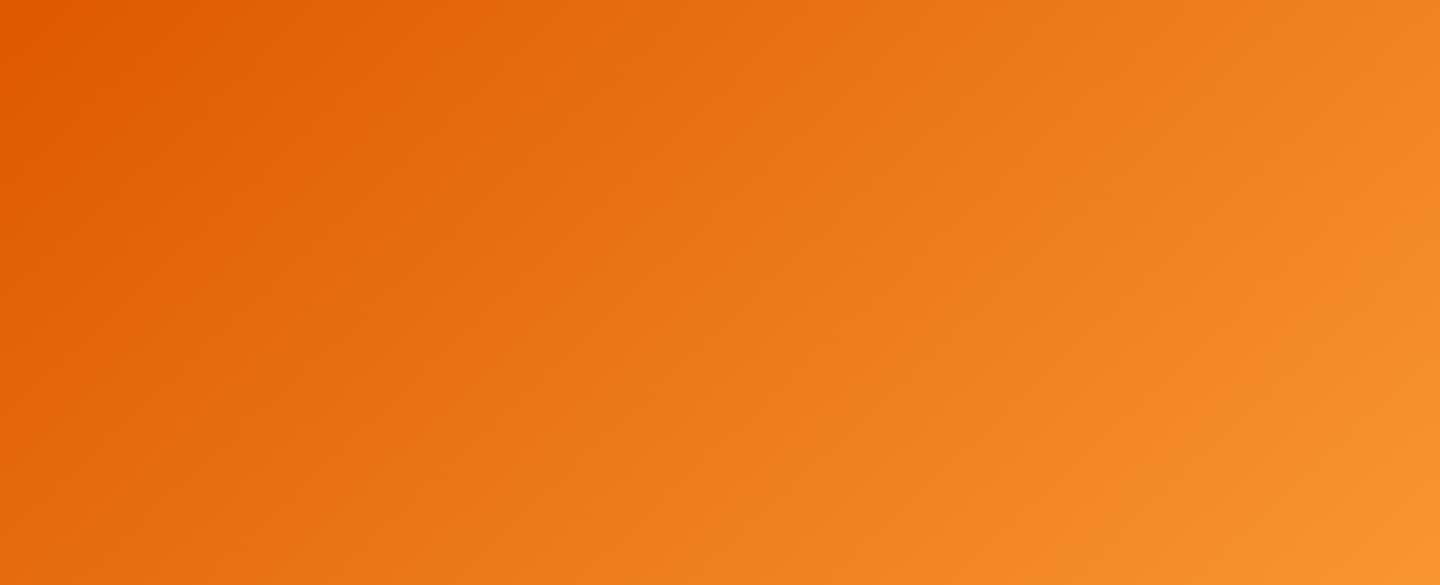
Bank of America Securities 2024 Global Metals, Mining and Steel Conference
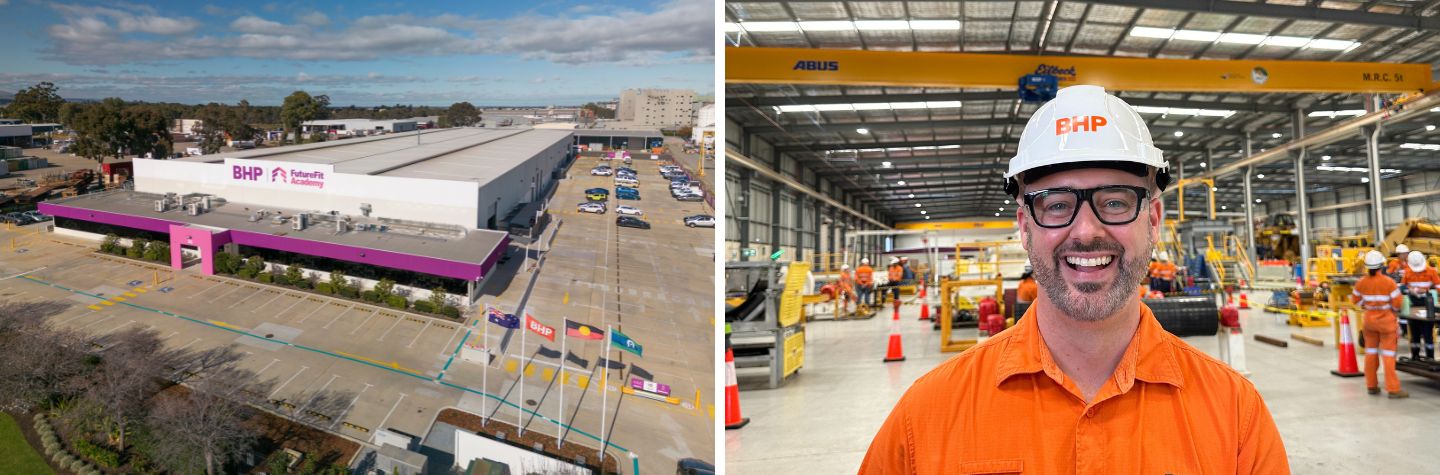

Meet Karol from Future Fit Academy in Perth
Meet Karol, a Trainor, from our Future Fit Academy in Perth.
IEEE Account
- Change Username/Password
- Update Address
Purchase Details
- Payment Options
- Order History
- View Purchased Documents
Profile Information
- Communications Preferences
- Profession and Education
- Technical Interests
- US & Canada: +1 800 678 4333
- Worldwide: +1 732 981 0060
- Contact & Support
- About IEEE Xplore
- Accessibility
- Terms of Use
- Nondiscrimination Policy
- Privacy & Opting Out of Cookies
A not-for-profit organization, IEEE is the world's largest technical professional organization dedicated to advancing technology for the benefit of humanity. © Copyright 2024 IEEE - All rights reserved. Use of this web site signifies your agreement to the terms and conditions.
Conference Proceedings
Gender Bias in AI Recruitment Systems: A Sociological-and Data Science-based Case Study
S Njoto, M Cheong, R Lederman, A McLoughney, L Ruppanner, A Wirth
International Symposium on Technology and Society, Proceedings | IEEE | Published : 2022
DOI: 10.1109/ISTAS55053.2022.10227106
This paper explores the extent to which gender bias is introduced in the deployment of automation for hiring practices. We use an interdisciplinary methodology to test our hypotheses: observing a human-led recruitment panel and building an explainable algorithmic prototype from the ground up, to quantify gender bias. The key findings of this study are threefold: identifying potential sources of human bias from a recruitment panel's ranking of CVs; identifying sources of bias from a potential algorithmic pipeline which simulates human decision making; and recommending ways to mitigate bias from both aspects. Our research has provided an innovative research design that combines social science ..
University of Melbourne Researchers
Citation metrics.
- DOI: 10.1109/ISTAS55053.2022.10227106
- Corpus ID: 261331473
Gender Bias in AI Recruitment Systems: A Sociological-and Data Science-based Case Study
- Sheilla Njoto , M. Cheong , +3 authors Anthony Wirth
- Published in International Symposium on… 10 November 2022
- Sociology, Computer Science
Figures and Tables from this paper
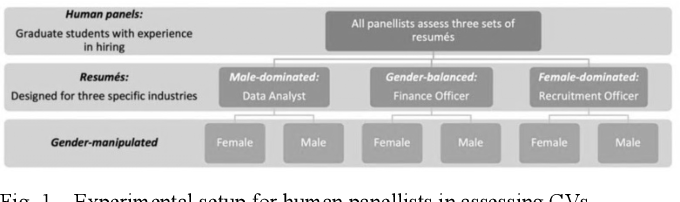
2 Citations
Professional presentation and projected power: a case study of implicit gender information in english cvs, d r a f t investigating gender and racial biases in dall-e mini images, 60 references, bias in bios: a case study of semantic representation bias in a high-stakes setting, mitigating bias in algorithmic hiring: evaluating claims and practices, battling algorithmic bias, mitigating gender bias in natural language processing: literature review, does fair ranking improve minority outcomes understanding the interplay of human and algorithmic biases in online hiring, gender discrimination in hiring: evidence from a cross-national harmonized field experiment, data statements for natural language processing: toward mitigating system bias and enabling better science, weapons of math destruction: how big data increases inequality and threatens democracy, whitened résumés, data and its (dis)contents: a survey of dataset development and use in machine learning research, related papers.
Showing 1 through 3 of 0 Related Papers
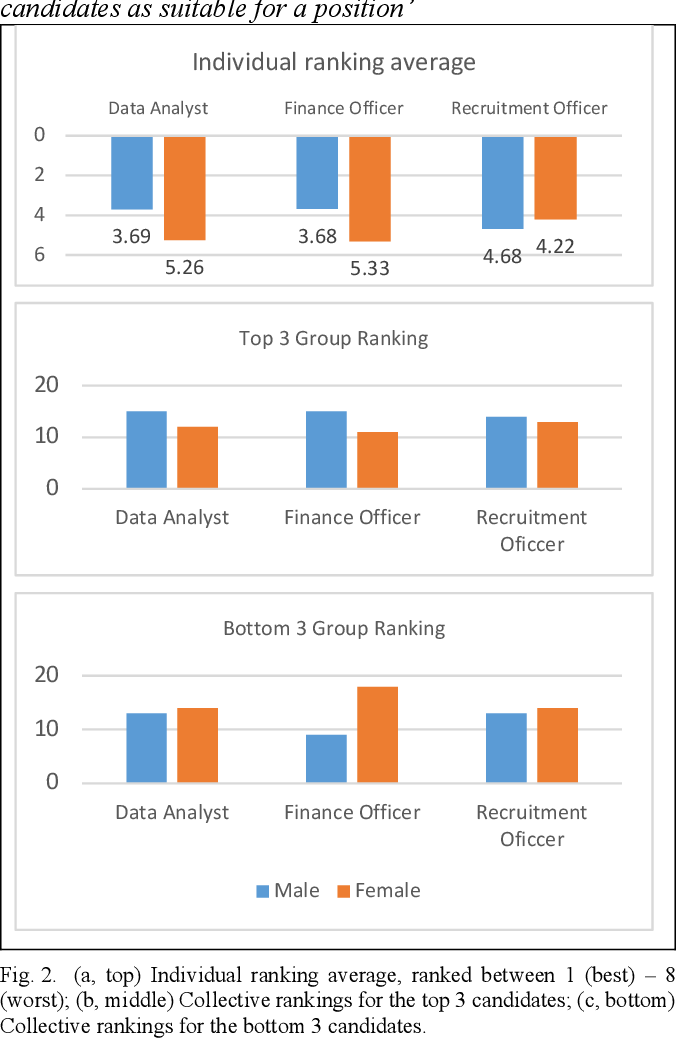
Published in International Symposium on Technology and Society 2022
Sheilla Njoto M. Cheong Reeva M. Lederman A. McLoughney Leah Ruppanner Anthony Wirth

- TECHNOLOGY BUSINESS ENTERPRISE COMMS START-UPS ALL
- SCIENCE INNOVATION MACHINES CLIMATE ALL
- CAREERS ADVICE PEOPLE EMPLOYERS JOBS NEWS ALL
- FUTURE HUMAN
- MORE VIDEO ADVERTISE FOLLOW US CONTACT ABOUT COOKIE & PRIVACY POLICY
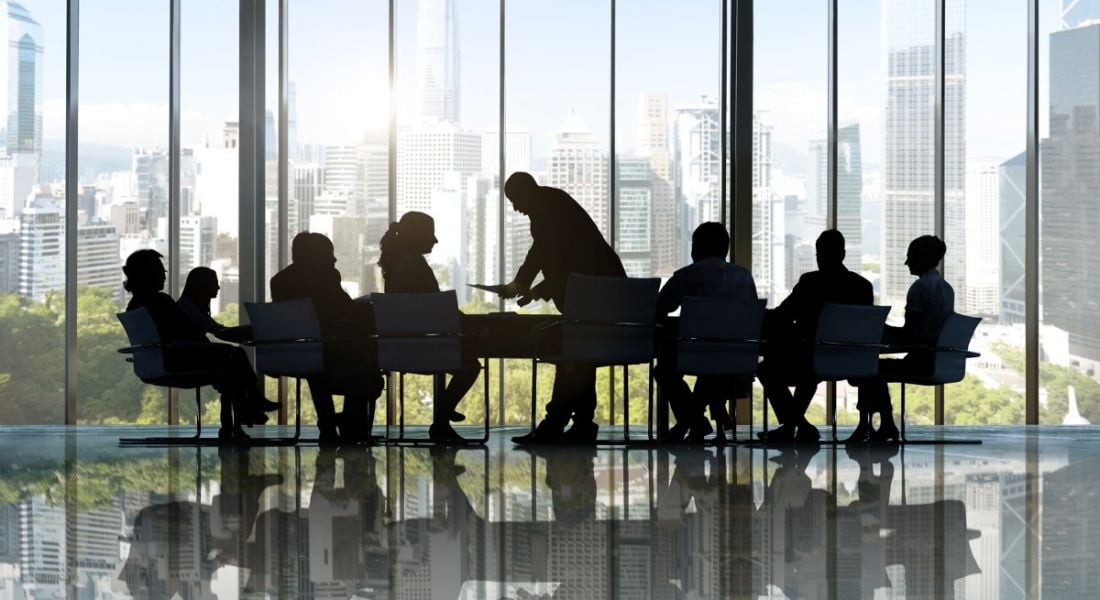
5 case studies of companies trying to correct the gender gap
by Eva Short
28 Nov 2018
The gender gap is one of the most contentious diversity issues in the world of work. These are just a few of the companies who have taken action to try and correct the issue.
It is no secret that the tech industry has struggled with a diversity problem. The problem spans all levels of business, from how female and minority employees are compensated, to their representation at all employee levels. However, the promise of a turning point is on the horizon as more companies start to pay attention and address the issue.
Research has continually shown that diverse teams outperform non-diverse teams financially. Knowing this, HR professionals at top firms have started to prioritise diversity in recruitment above all else.
In April 2018, the first slew of gender pay gap data was released in the UK following a law that was passed mandating large enterprises to do so. Prior to this, Iceland brought in legislation requiring organisations to provide proof that men and women are being compensated fairly, or face daily fines .
Many companies have taken steps to address gender gap issues. In a few cases, such as with tech giant Google , the efforts were found to be lacking. Yet in other cases, changes made have led to significant progress in addressing gender disparity. Here are some of the companies who have recently made inroads in this area, and how they did it.
Duolingo CEO and co-founder Luis von Ahn took to Twitter in October to highlight how the company had achieved a 50:50 ratio for new software engineer hires. Grimly, yet perhaps unsurprisingly, the response the company received was dominated by, in Von Ahn’s words, “men angrily arguing discrimination, and that we should hire the best people instead”.
Duolingo just tweeted about how we achieved a 50% female ratio of new engineering college graduate hires. We're very proud of this. I'm disappointed that the top comments were all from men angrily arguing discrimination, and that we should hire the best people instead. Idiots. https://t.co/WHjq2WnKzH — Luis von Ahn (@LuisvonAhn) October 11, 2018
The co-founder took great issue with this idea that promoting diversity somehow compromises quality. According to Von Ahn, all female hires had “either perfect or near-perfect GPAs from the best universities in the world, with stellar recommendations, and aced our very thorough interview process”.
Duolingo achieved its 50:50 ratio through a multipronged, data-driven approach. It only recruited from colleges with more than the US national average (18pc) of women enrolled in their computer science programmes, such as Duke, Cornell, Harvard and MIT. It then reached out to the women groups at each school and went along to any network events it held. It sponsored the 2017 Grace Hopper Conference and had all its female engineers attend. Finally, Duolingo says it put all its interviewers through unconscious bias training.
Duolingo has expressed a continued commitment to promoting diversity and gender parity in the workplace through both internal and external action.
Since Salesforce started examining its pay gap in 2016, it has shelled out $6m in order to correct compensation imbalances.

Join a culture that offers a world of possibilities

Always at the cutting edge of new innovation

The most actionable data to drive superior results

We impact life and health with science
This action aligns with promises that Salesforce CEO Marc Benioff made in 2015 in an interview with HuffPost. “My job is to make sure that women are treated 100pc equally at Salesforce in pay, opportunity and advancement,” Benioff said, noting that while he did not know what the gender pay gap was at the time, he was determined to find out and act accordingly.
Salesforce committed to undertaking regular pay audits, reflecting the fact that pay equity is, as executive VP of global employee success Cindy Robbins put it , “a moving target”.
In March 2018, Bloomberg editor in chief John Micklethwait announced a new staff policy regarding outside speaking engagement. So as to promote gender equality both within the newsroom and outside it, it is now a requirement that at least one woman is on any panel in order for a Bloomberg journalist to participate. “At the risk of stating the obvious, the woman could be you,” Micklethwait noted.
If this condition is not met, journalists will be required to decline, though there seems to be an appeals system in place if a journalist feels their participation on an all-male panel is necessary. “But I think this is a standard that we should be able to uphold on the vast majority of occasion,” Micklethwait concluded.
At cloud-based HR and payroll software company Gusto, the journey to gender parity began when software engineer Julia Lee asked Gusto co-founder and chief technology officer Edward Kim for a meeting. In it, she flagged that she was the only woman on the engineering team and disclosed her previous experiences of being dismissed due to her gender. Kim was receptive, and made a point to examine the gender breakdowns of other tech companies.
The results were dismal to say the least, so Kim met with Gusto’s HR team to come up with a strategy to address the issue. First, it elected to move away from using ‘masculine’ phrases such as “ninja rockstar coder” in its job ads. For the first six months of 2018, it focused solely on recruiting female engineers, though made a point to equally consider any men who approached the company so it would not breach anti-discrimination laws.
Like Duolingo, it sent representatives to the Grace Hopper conference. Now, Gusto reports that 51pc of its staff are women and more than 24pc of its engineers are women.
It also submitted to gender pay auditing by human resources firm Mercer, which found no disparity. It offers 16 weeks’ paid leave complete with generous grocery and housecleaning benefits for a primary parent.
Nike has had a year peppered with controversy in the world of gender, culminating when four women hit the sportswear company with a lawsuit over alleged discrimination. The women maintain that Nike violated US equal pay laws and fostered a work environment that allowed for sexual harassment, The Guardian reported in August of this year.
Prior to the suit being filed, Nike responded to the issues raised by ousting a number of high-profile executives in what was termed a “ harassment reckoning ”. A month before the suit was filed, Nike HR chief Monique Matheson admitted in a staff memo obtained by The Wall Street Journal that the company had failed women and that it wants to “to create a culture of true inclusion” and that, in order to do this, it needs to “improve representation of women and people of colour”. That same month, the company revealed that it planned to adjust the pay of 7,000 of its employees after an internal compensation review in order to address pay disparities.
Nike is arguably in the more nascent stages of dealing with its issues. These steps are more about putting out fires than they are about instituting structural change, but it’s an excellent start from the footwear giant.
Related: diversity , software engineers , equality , Salesforce
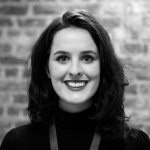
Eva Short was a journalist at Silicon Republic, specialising in the areas of tech, data privacy, business, cybersecurity, AI, automation and future of work, among others.
10 things you need to know, direct to your inbox
Sign up for the Daily Brief, Silicon Republic's weekday digest of essential sci-tech news
More from careers
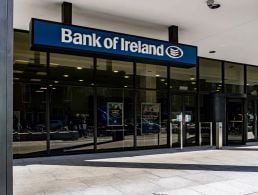
Your browser may not be supported by this website. Please upgrade to the latest version provided by your browser vendor.
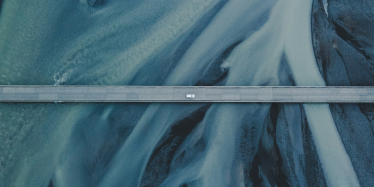
Advancing Gender Equality Through Targeted Interventions: A Case Study on Small Hydropower (SHP)
Challenges and opportunities for women and girls
By Eva Kremere
Subscribe to stay up to date with our latest articles
Women and girls worldwide face significant challenges in accessing equal opportunities, particularly in STEM (science, technology, engineering and mathematics) and the energy sector. Despite progress, gender disparity persists, with women often underrepresented in technical roles and leadership positions. These are further exacerbated by sociocultural norms, biases and structural barriers – such as lower literacy rates and limited access to education and training.
These challenges, however, also present unique opportunities. Addressing the specific needs of women and girls through targeted interventions can significantly advance gender equity. Empowering women in sectors like energy not only contributes to gender equality but also enhances economic development, community resilience and overall sustainability.
Figure 1. A woman working as an apprentice in a tailor’s shop connected to the hydroelectric grid in Democratic Republic of Congo 1
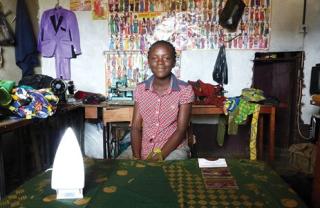
Approaches to promoting gender equity
Promoting gender equity requires a multifaceted approach involving various stakeholders. For example, gender-responsive policy reforms are needed to encourage female participation in traditionally male-dominated sectors. This includes educational reforms, incentives for organizations to promote gender diversity and support for gender-focused initiatives. Such initiatives could include capacity-building – another critical component – providing targeted training for women and girls with a focus on technical skills and leadership development. Raising awareness of opportunities in the STEM and energy sectors can also demonstrate career pathways for women and girls, and forge a connection between education and the workplace.
Community leaders and stakeholders should also be fully engaged in advocating for and supporting women’s participation in non-traditional sectors. This often challenges cultural norms and biases that are limiting women’s opportunities. Additionally, it is crucial that project investment criteria prioritize gender-inclusivity and equality. In addition to setting targets or minimum thresholds for female employment and leadership, this approach can incentivize training and capacity-building beyond the project itself.
Incorporating the needs of women
Small hydropower (SHP) projects offer a compelling case study on how targeted interventions can promote gender equity. The hydropower sector is expected to employ 3.7 million people by 2050, emphasizing the potential for more gender-inclusive job opportunities 2 SHP projects around the world have already been instrumental in challenging gender roles and creating opportunities for women in the energy sector, particularly in remote and rural areas. Nevertheless, women currently represent only 25% of the hydropower sector’s labour force, highlighting the need for more targeted interventions.
To overcome challenges, the report emphasizes the need for targeted actions by various stakeholders in the SHP sector 3 :
- Policymakers: Develop gender-responsive policies and incentives to encourage women’s participation in STEM and SHP sectors. This includes reforms in education and support for organizations promoting gender diversity in their workforce.
- SHP Developers and Industry Leaders: Adopt gender-sensitive approaches in project planning and execution. This involves establishing training and mentorship programmes to empower women for higher-level positions in SHP, going beyond traditional roles.
- Investors: Reorient investment criteria to focus on SHP projects that demonstrate a commitment to gender equality, particularly in creating technical job opportunities for women.
- Community Leaders: Advocate for women’s active involvement in SHP projects, ensuring that their contributions are valued and their potential fully realized.
Positive outcomes and lessons learned
A recent report on the SHP sector confirms that SHP projects have significantly increased employment opportunities for women in various roles, ranging from technical positions to leadership roles. Examples from Zambia and China illustrate how SHP projects have transformed local job markets by providing diverse career paths for women. Training programmes have equipped women with essential technical and leadership skills, enabling them to take on higher-level positions and contribute meaningfully to the sector. By involving women in SHP projects, communities have benefited from increased productivity and economic development. Women's involvement has also promoted greater community resilience and sustainability.
However, the report also identifies several lessons learned. For example, overcoming sociocultural barriers is crucial but complex. It needs to begin at the grass-roots level, engaging community leaders and promoting gender-sensitive approaches to change perceptions and encourage a wider acceptance of women in technical roles. Capacity-building programmes must also be tailored to the specific needs of women, considering factors such as literacy rates and language barriers. Providing training in local languages and accommodating women's schedules can enhance participation and effectiveness. Moreover, SHP investments should be evaluated not only on technical and financial merits, but also on their social impact – particularly regarding gender equality. This holistic approach ensures that projects contribute to sustainable and equitable development.
SHP projects demonstrate how targeted interventions can advance gender equality while promoting sustainable energy development. By incorporating the needs of women into project design and execution, SHP projects have created significant opportunities for women in the energy sector. The positive outcomes and lessons learned from these initiatives provide valuable insights for future efforts to promote gender equity across various sectors. As the world continues to address the challenges of climate change and social inequality, integrating gender-focused strategies into sustainable development projects offers a pathway towards a more inclusive and equitable future.
- Eva Kremere is expert in small hydropower projects at the Division of Climate & Technology Partnerships of the United Nations Industrial Development Organization (UNIDO).
Disclaimer: The views expressed in this article are those of the authors based on their experience and on prior research and do not necessarily reflect the views of UNIDO ( read more ).
- UNIDO,ICSHP (2019) World Small Hydropower Development Report 2019. Case study: MUTWANGA SMALL HYDROPOWER PLANT IN THE DEMOCRATIC REPUBLIC OF THE CONGO: VIRUNGA’S BOLD HYDROPOWER PLAN, TRANSFORMING LIVES IN A CONFLICT AREA. United Nations Industrial Development Organization, Vienna, Austria; International Center on Small Hydro Power, Hangzhou, China, available from: https://www.unido.org/wshpdr
- IHA (2021) How to guide on Environment and Social assessment. https://www.hydropower.org/publications/how-to-guide-on-environmental-and-social-assessment-and-management
- UNIDO, ICSHP (2022). World Small Hydropower Development Report 2022. United Nations Industrial Development Organization, Vienna, Austria; International Center on Small Hydro Power, Hangzhou, China. Available at www.unido.org/WSHPDR2022
Have your say
What is your opinion on the IAP?
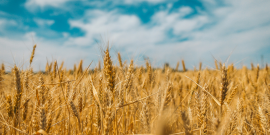
Ukraine in times of war: still time for greening industrial production
Sustainability and economic performance can be a winning partnership, even in a crisis.
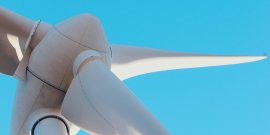
Promoting global sustainability by investing in the energy transition of developing countries
Developed countries should provide more resources for developing countries’ green transition to boost global climate efforts.
You are using an outdated browser. Please upgrade your browser to improve your experience.

- LinkedIn LinkedIn
- Twitter Twitter
- Facebook Facebook
Discrimination and bias in AI recruitment: a case study
31 October 2023
Barely a day goes by without the media reporting the potential benefits of or threats from AI. AI is being used more and more in workplace decisions: to make remuneration and promotion decisions; to allocate work; award bonuses; manage performance and make dismissal decisions. One of the common concerns is the propensity of AI systems to return biased or discriminatory outcomes. By working through a case study about the use of AI in recruitment, we examine the risks of unlawful discrimination and how that might be challenged in the employment tribunal.
Our case study begins with candidates submitting job applications which are to be reviewed and “profiled” by an AI system (the automated processing of personal data to analyse or evaluate people, including to predict their performance at work). We follow this through to the disposal of resulting employment tribunal claims from the unsuccessful candidates, and examine the risks of unlawful discrimination in using these systems. What emerges are the practical and procedural challenges for claimants and respondents (defendants) litigation procedures are ill-equipped for an automated world.
Bias and discrimination
Before looking at the facts, we consider the concepts of bias and discrimination in automated decision-making.
The Discussion Paper published for the AI Safety Summit organised by the UK government and held at Bletchley Park on 1 and 2 November highlighted the risks of bias and discrimination and commented:
“ Frontier AI models can contain and magnify biases ingrained in the data they are trained on, reflecting societal and historical inequalities and stereotypes. These biases, often subtle and deeply embedded, compromise the equitable and ethical use of AI systems, making it difficult for AI to improve fairness in decisions. Removing attributes like race and gender from training data has generally proven ineffective as a remedy for algorithmic bias, as models can infer these attributes from other information such as names, locations, and other seemingly unrelated factors. ”
What is bias and what is discrimination?
Much attention has been paid to the potential for bias and discrimination in automated decision-making. Bias and discrimination are not synonymous but often overlap. Not all bias amounts to discrimination and not all discrimination reflects bias.
A solution can be biased if it leads to inaccurate or unfair outcomes. A solution can be discriminatory if it disadvantages certain groups. A solution is unlawfully discriminatory if it disadvantages protected groups in breach of equality law.
How can bias and discrimination taint automated decision-making?
Bias can creep into an AI selection tool in a number of ways. For example, there can be: historical bias; sampling bias; measurement bias; evaluation bias; aggregation bias; and deployment bias.
To give a recent example, the shortlist of six titles for the 2023 Booker Prize included three titles by authors with the first name “Paul”. An AI programme asked to predict works to be shortlisted for this prize, is likely to identify being called “Paul” as a key factor. Of course, being called Paul will not have contributed to their shortlisting and identification of this as a determining factor amounts to bias. In doing so, the AI tool would be identifying a correlating factor which had not actually been a factor in the shortlisting; the tool’s prediction would therefore be biased as it would be inaccurate and unfair. In this case this bias is also potentially discriminatory as Paul is generally a male name and possibly also discriminatory on grounds of ethnicity and religion.
An algorithm can be tainted by historical bias or discrimination. AI algorithms are trained using past data. A recruitment algorithm takes data from past candidates and there will always be a risk of under-representation of particular groups in that training data. Bias and discrimination is even more likely to arise from the definition of success which the algorithm seeks to replicate based on successful recruitment in the past. There is an obvious risk of past discrimination being embedded in any algorithm.
This process presents the risk of random correlations being identified by AI algorithm, and there a several reported examples of this happening. One example from several years ago is an algorithm which identified being called Jared as one of the strongest correlators of success in a job. Correlation is not always causation.
An outcome may potentially be discriminatory but not be unfair or inaccurate and so not biased. If, say, a recruitment application concluded that a factor in selecting the best candidates was having at least ten years’ relevant experience, this would disadvantage younger candidates and a younger candidate may be excluded even if, in all other respects, they would be a strong candidate. This would be unlawful if it could just be justified on the facts. It would not, however, necessarily be a biased outcome.
There has been much academic debate on the effectiveness of AI in eliminating the sub-conscious bias of human subjectivity. Supporters argue that any conscious or sub-conscious bias is much reduced by AI. Critics argue that AI merely embeds and exaggerates historic bias.
Currently in the UK there are no AI specific laws regulating the use of AI in employment. The key relevant provisions at present are equality laws and data privacy laws. We have written about these in detail here . This case study focuses on discrimination claims under the Equality Act 2010.
The case study
Acquiring shortlisting tool
Money Bank gets many hundreds of applicants every year for its annual recruitment of 20 financial analysts to be based in its offices in the City of London. Shortlisting takes time and costly HR resources. Further, Money Bank is not satisfied with the suitability of the candidates shortlisted each year.
Money Bank, therefore, acquires an AI shortlisting tool, GetBestTalent, from a leading provider, CaliforniaAI, to incorporate into its shortlisting process.
CaliforniaAI is based in Silicon Valley in California and has no business presence in the UK. Money Bank is attracted by CaliforniaAI’s promises that GetBestTalent will identify better candidates, more quickly and more cheaply than by relying on human-decision makers. Money Bank is also reassured that CaliforniaAI’s publicity material states that GetBestTalent has been audited to ensure that it is bias and discrimination-free.
Money Bank was sued recently by an unsuccessful job applicant claiming that they were unlawfully discriminated against when rejected for a post. This case was settled but proved costly and time-consuming to defend. Money Bank wants, at all costs, to avoid further claims.
Data protection impact assessment
Money Bank’s Data Protection Officer (DPO) conducts a data protection impact assessment (DPIA) into the proposed use by Money Bank of GetBestTalent given the presence of various high-risk indicators, including the innovative nature of the technology and profiling. Proposed mitigations following this assessment include bolstering transparency around the use of automation by explaining clearly that it will form part of the shortlisting process; ensuring that a HR professional will review all successful applications; and confirming with CaliforniaAI that the system is audited for bias and discrimination. On that basis, the DPO considers that the shortlisting decisions are not “solely automated” and is satisfied that Money Bank’s proposed use of the system complies with UK data protection laws (this case study does not consider the extent to which the DPO is correct in considering Money Bank’s GDPR obligations to have been satisfied in this case).
Money Bank enters into a data processing agreement with CaliforniaAI that complies with UK GDPR requirements. Money Bank also notes that CaliforniaAI is self-certified as compliant with the UK extension to the EU-US Data Privacy Framework.
AI and recruitment
GetBestTalent is an off-the-shelf product and CaliforniaAI’s best seller. It has been developed for markets globally and used for many years though it is updated by the developers periodically. The use of algorithms, and the use of AI in HR systems specifically, is not new but has been growing rapidly in recent years. It is being used at different stages of the recruitment process but one of the most common applications of AI by HR is to shortlist vast numbers of candidates down to a manageable number.
AI shortlisting tools can be bespoke (developed specifically for the client); off-the-shelf; or based on an off-the-shelf system but adapted for the client. GetBestTalent algorithm is based on “supervised learning” where the input data and desired output are known and the machine learning method identifies the best way of achieving the output from the inputted data. This application is “static” in that it only changes when CaliforniaAI developer’s make changes to the algorithm. Other systems, known as dynamic systems, can be more sophisticated and continuously learn how to make the algorithm more effective at achieving its purpose.
Sifting applicants
This year 800 candidates apply for the 20 financial analyst positions at Money Bank. Candidates are all advised that Money Bank will be using automated profiling as part of the recruitment process.
Alice, Frank and James are unsuccessful, and all considered themselves strong candidates with the qualifications and experiences advertised for the role. Alice is female, Frank is black, and James is 61 years old. Each is perplexed at their rejection and concerned that their rejection was unlawfully discriminatory. All three are suspicious of automated decision-making and have had read or heard about concerns about these systems.
Discrimination claims in the employment tribunal
Alice, Frank and James each contact Money Bank challenging their rejection. Money Bank asks one of its HR professionals, Nadine, to look at each of the applications. There is little obvious to differentiate these applications from the shortlisted candidates - and Nadine cannot see that they are obviously stronger - so confirms the results of the shortlisting process.
The Bank responds to Alice, Frank and James saying that it has reviewed the rejections, and that it uses a reputable AI system which they are reassured does not discriminate unlawfully but they do not have any more information as the criteria used are developed by the algorithm and are not visible to Money Bank. The data processing agreement between Money Bank and CaliforniaAI requires CaliforniaAI (as processor) to assist Money Bank to fulfil its obligation (as controller) to respond to rights requests, but does not specifically require CaliforniaAI to provide detailed information on the logic behind the profiling nor its application to individual candidates.
Alice, Frank and James all start employment tribunal proceedings in the UK claiming, respectively sex, race and age discrimination in breach of the UK’s Equality Act. They:
- claim direct and indirect discrimination against Money Bank; and
- sue CaliforniaAI for inducing and/or causing Money Bank to discriminate against them.
Despite CaliforniaAI having no business presence in the UK and despite the process being more complicated, the claimants can bring proceedings against an overseas party in the UK employment tribunal.
Unless the claimants are aware of each other’s cases, in reality, these cases are likely to proceed independently. However, for the purposes of this case study, all three approach the same lawyer who successfully applies for the cases to be joined and heard together.
Alice, Frank and James recognise that, despite their suspicions, they will need more evidence to back up their claims. They, therefore, contact Money Bank and CaliforniaAI asking for disclosure of documents with the data and information relevant to their rejections.
They also write to Money Bank and California AI with data subject access requests (DSARs) making similar requests for data. These requests are made by reason of their rights under UK data protection law over which the employment tribunal has no jurisdiction so is independent of their employment tribunal claims.
Seeking disparate impact data
In order to seek to establish discrimination, each candidate requests data:
- Alice asks Money Bank for documents showing the data on both the total proportion of candidates, and the proportion of successful candidates, who were women. This is needed to establish her claim of indirect sex discrimination.
- Frank asks for the same in respect of the Black, Black British, Caribbean or African ethnic group.
- James asks for the data for both over 60-year-olds and over 50-year-olds.
They also ask CaliforniaAI for the same data from all exercises in which GetBestTalent has been used globally.
Would a tribunal order a disclosure request of this nature? In considering applications for the provision of information or the disclosure of documents or data, an employment tribunal must consider the proportionality of the request. It is more likely to grant applications which require extensive disclosure or significant time or cost to provide the requested information where the sums claimed are significant.
In this case, Money Bank has the information sought about the sex, ethnicity and age of both all candidates and of those who were successful which it records as part of its equality monitoring procedures. Providing it, therefore, would not be burdensome. In other cases, the employer may not have this data. CaliforniaAI has the means to extract the data sought, at least from many of the uses of GetBestTalent. However, it would be a time-consuming and costly exercise to do this.
Both respondents refuse to provide any of the data sought. Money Bank argues that this is merely a fishing exercise as none of the claimants have any evidence to support a discrimination claim. They also argue that the system has been audited for discrimination and, therefore, the clams are vexatious. CaliforniaAI also regards the information sought as a trade secret (of both itself and its clients) and also relies on the time and cost involved in gathering it.
In response the claimants apply to the employment tribunal for an order requiring the respondents to provide the data and information requested.
The tribunal orders Money Bank to provide the claimants with the requested documents. It declines, however, to make the order requested against CaliforniaAI.
In theory, the tribunal has the power to make the requested order against CaliforniaAI. Although, it cannot make the order against an overseas person which is not a party to the litigation, in this case California AI is a party. However, the tribunal regards the request as manifestly disproportionate and gives this request short shrift.
The disparate impact data does not amount to the individuals’ personal data so is not relevant to their DSARs.
Seeking equality data
The claimants also request from Money Bank documents showing the details of: a) the gender, ethnic and age breakdown of the Bank’s workforce in the UK (as the case may be); b) the equality training of the managers connected with decision to use the GetBestTalent solution; and c) any discrimination complaints made against Money Bank in the last five years and the outcome.
Money Bank refuses all requests as it argues that the claim relates to the discriminatory impact of CaliforniaAI’s recruitment solution so that all these other issues are irrelevant. It could provide the information relatively easily but is mindful that the Bank has faced many discrimination claims in recent years and has settled or lost a number so does not want to highlight this.
The tribunal refuses to grant the requests for the equality data as it considers it unnecessary for the claimants to prove their case. The claimants will, however, still be able to point to Money Bank’s failure to provide this information in seeking to draw inferences. The tribunal also refuses the request for details of past complaints (though details of tribunal claims which proceeded to a hearing are available from a public register).
The tribunal does ask Money Bank to provide details of the equality training provided to the relevant managers as it was persuaded that this is relevant to the issues to be decided.
This information does not amount to the individuals’ personal data so is not relevant to their DSARs.
Disclosing the algorithm and audit
The claimants also asked CaliforniaAI to provide it with:
- a copy of the algorithm used in the shortlisting programme;
- the logic and factors used by the algorithm in achieving is output (i.e. explainability information relating to their individual decisions); and
- the results of the discrimination audit.
In this case, CaliforniaAI has the information to explain the decisions, but this is not auto-generated (as it can be with some systems) or provided to Money Bank. Money Bank’s contract with CaliforniaAI does not explicitly require it to provide this information.
CaliforniaAI refuses to provide any of the requested information on the basis that these amount to trade secrets and also that the code would be meaningless to the claimants. The claimants counter that expert witnesses should be able to consider the code as medical experts would where complex medical evidence is relevant to tribunal proceedings.
The tribunal judge is not persuaded by the trade secret argument. If disclosed the code would be in the bundle of documents to which observers from the general public would have access (though couldn’t copy or remove). The tribunal has wide powers to regulate its own procedure and, in theory, could take steps in exceptional cases to limit public access to trade secrets.
However, the tribunal decides not to order disclosure of the code on the grounds of proportionality. It spends more time deliberating over the “explainability” information and the details of the auditing of the system.
Ultimately, it decides not to require disclosure of either. It considers that, in so far as the direct discrimination claims are concerned, it requires more than the assertion by the claimants that they have been directly discriminated against to make the requested order proportionate. If the sums likely to be awarded had been greater, it may well have reached a different decision here. In so far as Alice’s indirect claim is concerned, the explainability information and audit are more likely to be relevant to Money Bank’s defence than Alice’s claim so leaves it Money Bank to decide whether or not to disclose it.
Arguably, UK GDPR requires Money Bank to provide the explainability information in response to the data subject access request, and for Money Bank’s data processing agreement with CaliforniaAI to oblige the American company to provide this. However, both respond to the DSAR refusing to provide this information (this case study does not consider the extent to which they might be justified in doing so under UK GDPR).
What did the data show?
The data provided by Money Bank shows that of the 800 job applicants: 320 were women (40%) and 480 were men (60%); 80 described their ethnicity as Black, Black British, Caribbean or African (10%); and James was the only applicant over the age of 50.
Of the 320 women, only four were successful (20% of total shortlisted) whereas 16 men were shortlisted (80% of shortlisted). Of the 80 applicants from Frank’s ethnic group, three were appointed (15% of successful applicants). Therefore, the data shows that the system had a disparate impact against women but not against Black, Black British, Caribbean or African candidates. There was no data to help James with an indirect discrimination claim.
320 | 40% | 4 | 20% | |
80 | 10% | 3 | 15% | |
1 | <1% | 0 | - |
After consideration of the data, Frank and James abandon their indirect discrimination claims.
Establishing discrimination: who needs to prove what?
Indirect discrimination
Alice needs to establish:
- a provision, criterion or practice (PCP);
- that the PCP has a disparate impact on women;
- that she is disadvantaged by the application of the PCP; and
- that the PCP is not objectively justifiable.
Alice relies on the AI application used by Money Bank as her PCP.
If the decision to reject her had been “explainable” then, as is the case with most human-decisions, the PCP could also be the actual factor which disadvantaged her.
Putting this into practice, let’s say it could have been established from the explainability information that the algorithm had identified career breaks as a negative factor. Alice has had two such breaks and might, in such circumstances, allege that this was unlawfully indirectly discriminatory. A tribunal may well accept that such a factor disadvantages women without needing data to substantiate this. Money Bank would then need to show either that this had not disadvantaged Alice or that such a factor was objectively justifiable.
Neither defence would be easy in this case. It is possible that the respondents could run on a counterfactual to show that Alice had not been disadvantaged by her career breaks. This would mean applying the application to an alternative set of facts – so here, running it against Alice’s application but without career breaks to show she would not have been shortlisted in any event.
In our case, however, Money Bank does not have an explanation for Alice’s failure to be shortlisted.
2. Disparate impact
Alice relies on the data to show a disparate impact.
The respondents could seek to argue that there is no disparate impact based on the auditing undertaken by CaliforniaAI using larger numbers of aggregate audits and argue that the Money Bank’s data reflects a random outcome. A tribunal is certain not to accept this argument at face value. Further, the legal tests in the UK and the US are not the same so any auditing in the US will be of reduced value.
In such a case, the respondents could seek to introduce data from its verification testing or the use of the platform by other employers. This may then require expert evidence on the conclusions to be drawn from the audit data.
In our case, neither the audit nor evidence of the impact of GetBestTalent on larger numbers are before the tribunal. Indeed, here, California AI refused to disclose it.
3. Disadvantages protected group and claimant
Alice does not have to prove why a PCP disadvantages a particular group. The Supreme Court in Essop v Home Office (2017) considered a case where black candidates had a lower pass rate than other candidates under a skills assessment test. The claimants were unable to explain why the test disadvantaged that group, but this was not a bar to establishing indirect discrimination.
The PCP (the GetBestTalent solution) clearly disadvantages Alice personally as her score was the reason she was not shortlisted.
4. Justification
Alice satisfies the first three steps in proving her case.
The burden will then pass to Money Bank to show that the use of this particular application was justified – that it was a proportionate means of achieving a legitimate aim.
What aims could Money Bank rely on? Money Bank argues that its legitimate aim is decision-making which is quicker, cheaper, results in better candidates and discriminates less than with human-made decisions.
Saving money is tricky: it cannot be a justification to discriminate to save money, but this can be relevant alongside other aims. Nonetheless, Money Bank is likely to establish a legitimate aim for the introduction of automation in its recruitment process based on the need to make better and quicker decisions and avoid sub—conscious bias. The greater challenge will be showing that the use of this particular solution was a proportionate means of achieving those aims.
In terms of the objective of recruiting better candidates, Money Bank would have to do more than merely assert that the use of GetBestTalent meant higher quality short-listed candidates. It might, for example, point to historic problems with the quality of successful candidates. This would help justify automation, but Money Bank would still have to justify the use of this particular system.
Money Bank seeks to justify its use of GetBestTalent and satisfy its proportionality by relying on its due diligence. However, it did no more than ask the question of CaliforniaAI who reassured Money Bank that it had been audited.
It also points to the human oversight where a HR professional reviews all candidates which the system proposes to shortlist to verify this decision. The tribunal is unimpressed with the human oversight as this did not extend to oversight of all the unsuccessful applications.
Pulling this together, would a tribunal accept the use of this platform satisfied the objective justification test? This is unlikely. In all likelihood, Alice would succeed, and the matter would proceed to a remedies hearing to determine her compensation.
Direct discrimination
Alice is also pursuing a direct sex discrimination claim and Frank and James, not deterred by the failure to get their indirect discrimination claims off the ground, have also continued their direct race and age discrimination claims respectively. The advantage for Alice in pursuing a direct discrimination claim is that this discrimination (unlike indirect discrimination) cannot be justified, and the fact of direct discrimination is enough to win her case.
Each applicant has to show that they were treated less favourably (i.e., not shortlisted) because of their protected characteristic (sex, race, age respectively). To do this, the reason for the decision not to shortlist must be established.
They have no evidence of the reason, but this does not necessarily defeat their claims. Under UK equality law, the burden of proof can, in some circumstances, transfer so that it is for the employer to prove that it did not discriminate. To prove this, the employer would then have to establish the reason and show that it was not the protected characteristic of the claimant in question. In this case, this would be very difficult for Money Bank as it does not know why the candidates were not shortlisted.
What is required for the burden of proof to transfer? The burden of proof will transfer if there are facts from which the court could decide that discrimination occurred. This is generally paraphrased as the drawing of inferences of discrimination from the facts. If inferences can be drawn, the employer will need to show that there was not discrimination.
Prospects of success
Looking at each claimant in turn:
- Frank : he will struggle to draw inferences as there is no disparate impact inferring any less favourable treatment. The absence of any disparate impact does not mean that Frank could not have been directly discriminated against but without more his claim in unlikely to get anywhere. He does not have an explanation for the basis of the decision or the ethnic breakdown of its current workforce. He has limited information about Money Bank’s approach to equality. He can’t prove facts which in the absence of an explanation show prima facie discrimination so his claim fails.
- James : his claim is unlikely to be rejected as quickly as Frank’s as the data doesn’t help prove or disprove his claim. James could try to rely on the absence of older workers in the workforce, any lack of training or monitoring and past claims if had the information as well as the absence of an explanation for his rejection but, in reality, this claim becomes pretty hopeless.
- Alice : her may be on stronger ground. She can point to the disparate impact data as a ground for inferences but this will not normally be enough on its own to shift the burden of proof. Alice can also point to the opaque decision-making. Money Bank could rebut this if the decision was sufficiently “explainable” so the reason for Alice’s rejection could be identified. However, it cannot do so here. The dangers of inexplicable decisions are obvious.
Would the disparate impact and opaqueness be enough to draw inferences? Probably not – particularly if Alice does not have any of the equality data or information about past discrimination claims referred to above and the equality training information does not show a total disregard to equality. She could try and get information about these on cross-examination of witnesses and could point to MoneyBank’s failure to provide the equality data as grounds for drawing inferences and reversing the burden of proof. However, after carefully balancing the arguments, the tribunal decides in our case that Alice can’t prove facts which, in the absence of an explanation, show prima facie discrimination. This means that her direct discrimination claim fails.
If inferences had been drawn and Money Bank had been required to demonstrate that the protected characteristic in question had not been the reason for its decision, Money Bank would have argued that it anonymises the candidate data and ensures age, sex and ethnicity of candidates is omitted and that, therefore, the protected characteristic could not have informed the decision. However, as studies have shown how difficult it is to supress this, the tribunal would give this argument short shrift. If inferences had been drawn, Alice would have, in all likelihood, succeeded with her direct discrimination claim as well as her indirect discrimination claim.
Causing or inducing discrimination
If Money Bank is liable then CaliforniaAI is likely to also be liable for causing/inducing this unlawful discrimination by supplying the system on which Money Bank based its decision. CaliforniaAI cannot be liable if Money Bank is not liable.
The case of Alice, Frank and James highlights the real challenges for claimants winning discrimination claims where AI solutions have been used in employment decision-making. The case also illustrates the risks and pitfalls for employers using such solutions. It illustrates how both existing data protection and equality laws are unsuited for regulating automated employment decisions.
Looking forward, as the UK and other countries are debating over the appropriate level of regulation over AI in areas such as employment. It is to be hoped that these regulations recognise and embrace the inevitably of increased automation but, at the same time, ensure that individuals’ rights are protected effectively.
Related items
Related services, artificial intelligence (ai) – your legal experts.
AI has been all over the headlines in recent months. Generative AI can already produce written content, images and music which is often extremely impressive, if not yet perfect – and it is increasingly obvious that in the very near future, AI’s capabilities will revolutionise the way we work and live our lives.
- Central & Eastern Europe
- Central & South America
- Middle East & Asia Pacific
- North America
- Western Europe
- Argentina Bruchou & Funes de Rioja Bruchou & Funes de Rioja
- Australia Corrs Chambers Westgarth Corrs Chambers Westgarth
- Austria Schima Mayer Starlinger Schima Mayer Starlinger
- Bahrain Al Tamimi & Co (Bahrain) Al Tamimi & Co (Bahrain)
- Belgium Claeys & Engels Claeys & Engels
- Brazil Veirano Advogados Veirano Advogados
- Bulgaria BOYANOV & Co. BOYANOV & Co.
- Canada Mathews Dinsdale Mathews Dinsdale
- Chile Munita & Olavarría Munita & Olavarría
- China Fangda Partners Fangda Partners
- Colombia Brigard Urrutia Brigard Urrutia
- Bahtijarević & Krka" data-country="31"> Croatia Divjak Topić Bahtijarević & Krka Divjak Topić Bahtijarević & Krka
- & Associates LLC" data-country="30"> Cyprus George Z. Georgiou & Associates LLC George Z. Georgiou & Associates LLC
- Czech Republic Randl Partners, advokátní kancelář, s.r.o. Randl Partners, advokátní kancelář, s.r.o.
- Denmark Norrbom Vinding Norrbom Vinding
- Estonia COBALT (Estonia) COBALT (Estonia)
- Finland Dittmar & Indrenius Dittmar & Indrenius
- France Capstan Avocats Capstan Avocats
- Germany Kliemt.HR Lawyers Kliemt.HR Lawyers
- Greece KREMALIS LAW FIRM KREMALIS LAW FIRM
- Hong Kong Lewis Silkin (Hong Kong) Lewis Silkin (Hong Kong)
- Hungary Bozsonyik-Fodor Legal Bozsonyik-Fodor Legal
- India Kochhar & Co. Kochhar & Co.
- Ireland Lewis Silkin Ireland LLP Lewis Silkin Ireland LLP
- Israel Herzog Fox & Neeman Herzog Fox & Neeman
- Italy Toffoletto De Luca Tamajo Toffoletto De Luca Tamajo
- Japan Anderson Mori & Tomotsune Anderson Mori & Tomotsune
- Kazakhstan AEQUITAS Law Firm AEQUITAS Law Firm
- Latvia COBALT (Latvia) COBALT (Latvia)
- Lithuania COBALT (Lithuania) COBALT (Lithuania)
- Luxembourg CASTEGNARO CASTEGNARO
- Malta Ganado Advocates Ganado Advocates
- Mexico Basham, Ringe y Correa S.C. Basham, Ringe y Correa S.C.
- Netherlands Blom Veugelers Zuiderman Advocaten Blom Veugelers Zuiderman Advocaten
- Netherlands Bronsgeest Deur Advocaten Bronsgeest Deur Advocaten
- New Zealand Kiely Thompson Caisley Kiely Thompson Caisley
- Norway Advokatfirmaet Hjort DA Advokatfirmaet Hjort DA
- Peru Vinatea y Toyama Vinatea y Toyama
- Poland Raczkowski Raczkowski
- Portugal pbbr pbbr
- Romania Nestor Nestor Diculescu Kingston Petersen (NNDKP) Nestor Nestor Diculescu Kingston Petersen (NNDKP)
- Saudi Arabia Al Tamimi & Co (Saudi Arabia) Al Tamimi & Co (Saudi Arabia)
- Serbia Karanovic & Partners Karanovic & Partners
- Singapore Rajah & Tann Singapore Rajah & Tann Singapore
- Slovakia NITSCHNEIDER & PARTNERS NITSCHNEIDER & PARTNERS
- Slovenia ŠELIH & PARTNERJI Law Firm ŠELIH & PARTNERJI Law Firm
- South Korea Yulchon LLC Yulchon LLC
- Spain Sagardoy Abogados Sagardoy Abogados
- Sweden Elmzell Advokatbyrå Elmzell Advokatbyrå
- Switzerland Blesi & Papa Blesi & Papa
- Thailand Rajah & Tann Thailand Rajah & Tann Thailand
- Turkiye Bener Law Office Bener Law Office
- Ukraine Vasil Kisil & Partners Vasil Kisil & Partners
- United Arab Emirates Al Tamimi & Co (UAE) Al Tamimi & Co (UAE)
- United Kingdom Sackers Sackers
- United Kingdom Lewis Silkin Lewis Silkin
- Venezuela D'Empaire D'Empaire
- Our Lawyers
- Learn & Connect
- Global HR Law Guide
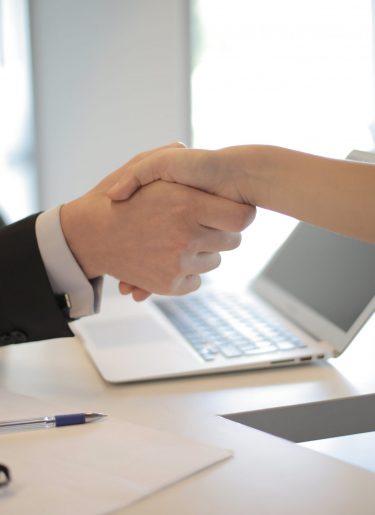
Discrimination and bias in AI recruitment: a case study
Introduction.
Our case study begins with candidates submitting job applications which are to be reviewed and ‘profiled’ by an AI system (the automated processing of personal data to analyse or evaluate people, including to predict their performance at work). We follow this through to the disposal of resulting employment tribunal claims from the unsuccessful candidates, and examine the risks of unlawful discrimination in using these systems. What emerges are the practical and procedural challenges for claimants and respondents (defendants) arising from litigation procedures that are ill-equipped for an automated world.
Bias and discrimination
Before looking at the facts, we consider the concepts of bias and discrimination in automated decision-making.
The Discussion Paper published for the AI Safety Summit organised by the UK government and held at Bletchley Park on 1 and 2 November highlighted the risks of bias and discrimination and commented:
What is bias and what is discrimination?
Much attention has been paid to the potential for bias and discrimination in automated decision-making. Bias and discrimination are not synonymous but often overlap. Not all bias amounts to discrimination and not all discrimination reflects bias.
A solution can be biased if it leads to inaccurate or unfair outcomes. A solution can be discriminatory if it disadvantages certain groups. A solution is unlawfully discriminatory if it disadvantages protected groups in breach of equality law.
How can bias and discrimination taint automated decision-making?
Bias can creep into an AI selection tool in a number of ways. For example, there can be: historical bias; sampling bias; measurement bias; evaluation bias; aggregation bias; and deployment bias.
To give a recent example, the shortlist of six titles for the 2023 Booker Prize included three titles by authors with the first name ‘Paul’. An AI programme asked to predict works to be shortlisted for this prize, is likely to identify being called ‘Paul’ as a key factor. Of course, being called Paul will not have contributed to their shortlisting and identification of this as a determining factor amounts to bias. In doing so, the AI tool would be identifying a correlating factor which had not actually been a factor in the shortlisting; the tool’s prediction would therefore be biased as it would be inaccurate and unfair. In this case this bias is also potentially discriminatory as Paul is generally a male name and possibly also discriminatory on grounds of ethnicity and religion.
An algorithm can be tainted by historical bias or discrimination. AI algorithms are trained using past data. A recruitment algorithm takes data from past candidates and there will always be a risk of under-representation of particular groups in that training data. Bias and discrimination is even more likely to arise from the definition of success which the algorithm seeks to replicate based on successful recruitment in the past. There is an obvious risk of past discrimination being embedded in any algorithm.
This process presents the risk of random correlations being identified by AI algorithm, and there a several reported examples of this happening. One example from several years ago is an algorithm which identified being called Jared as one of the strongest correlates of success in a job. Correlation is not always causation.
An outcome may potentially be discriminatory but not be unfair or inaccurate and so not biased. If, say, a recruitment application concluded that a factor in selecting the best candidates was having at least ten years’ relevant experience, this would disadvantage younger candidates and a younger candidate may be excluded even if, in all other respects, they would be a strong candidate. This would be unlawful if it could not be justified on the facts. It would not, however, necessarily be a biased outcome.
There has been much academic debate on the effectiveness of AI in eliminating the sub-conscious bias of human subjectivity. Supporters argue that any conscious or sub-conscious bias is much reduced by AI. Critics argue that AI merely embeds and exaggerates historic bias.
Currently in the UK there are no AI specific laws regulating the use of AI in employment. The key relevant provisions at present are equality laws and data privacy laws. This case study focuses on discrimination claims under the Equality Act 2010.
The case study
Acquiring the shortlisting tool.
Money Bank gets many hundreds of applicants every year for its annual recruitment of 20 financial analysts to be based in its offices in the City of London. Shortlisting takes time and costly HR resources. Further, Money Bank is not satisfied with the suitability of the candidates shortlisted each year.
Money Bank, therefore, acquires an AI shortlisting tool, GetBestTalent, from a leading provider, CaliforniaAI, to incorporate into its shortlisting process.
CaliforniaAI is based in Silicon Valley in California and has no business presence in the UK. Money Bank is attracted by CaliforniaAI’s promises that GetBestTalent will identify better candidates, more quickly and more cheaply than by relying on human-decision makers. Money Bank is also reassured that CaliforniaAI’s publicity material states that GetBestTalent has been audited to ensure that it is bias and discrimination-free.
Money Bank was sued recently by an unsuccessful job applicant claiming that they were unlawfully discriminated against when rejected for a post. This case was settled but proved costly and time-consuming to defend. Money Bank wants, at all costs, to avoid further claims.
Data protection impact assessment
Money Bank’s Data Protection Officer (DPO) conducts a data protection impact assessment (DPIA) into the proposed use by Money Bank of GetBestTalent given the presence of various high-risk indicators, including the innovative nature of the technology and profiling. Proposed mitigations following this assessment include bolstering transparency around the use of automation by explaining clearly that it will form part of the shortlisting process; ensuring that an HR professional will review all successful applications; and confirming with CaliforniaAI that the system is audited for bias and discrimination. On that basis, the DPO considers that the shortlisting decisions are not ‘solely automated’ and is satisfied that Money Bank’s proposed use of the system complies with UK data protection laws (this case study does not consider the extent to which the DPO is correct in considering Money Bank’s GDPR obligations to have been satisfied in this case).
Money Bank enters into a data processing agreement with CaliforniaAI that complies with UK GDPR requirements. Money Bank also notes that CaliforniaAI is self-certified as compliant with the UK extension to the EU-US Data Privacy Framework.
AI and recruitment
GetBestTalent is an off-the-shelf product and CaliforniaAI’s best seller. It has been developed for markets globally and used for many years though it is updated by the developers periodically. The use of algorithms, and the use of AI in HR systems specifically, is not new but has been growing rapidly in recent years. It is being used at different stages of the recruitment process but one of the most common applications of AI by HR is to shortlist vast numbers of candidates down to a manageable number.
AI shortlisting tools can be bespoke (developed specifically for the client); off-the-shelf; or based on an off-the-shelf system but adapted for the client. GetBestTalent algorithm is based on ‘supervised learning’ where the input data and desired output are known and the machine learning method identifies the best way of achieving the output from the inputted data. This application is ‘static’ in that it only changes when CaliforniaAI developer’s make changes to the algorithm. Other systems, known as dynamic systems, can be more sophisticated and continuously learn how to make the algorithm more effective at achieving its purpose.
Sifting applicants
This year 800 candidates apply for the 20 financial analyst positions at Money Bank. Candidates are all advised that Money Bank will be using automated profiling as part of the recruitment process.
Alice, Frank and James are unsuccessful, and all considered themselves strong candidates with the qualifications and experiences advertised for the role. Alice is female, Frank is black, and James is 61 years old. Each is perplexed at their rejection and concerned that their rejection was unlawfully discriminatory. All three are suspicious of automated decision-making and have had read or heard about concerns about these systems.
Discrimination claims in the employment tribunal
Alice, Frank and James each contact Money Bank challenging their rejection. Money Bank asks one of its HR professionals, Nadine, to look at each of the applications. There is little obvious to differentiate these applications from the shortlisted candidates – and Nadine cannot see that they are obviously stronger – so confirms the results of the shortlisting process.
The Bank responds to Alice, Frank and James saying that it has reviewed the rejections, and that it uses a reputable AI system which they are reassured does not discriminate unlawfully but they do not have any more information as the criteria used are developed by the algorithm and are not visible to Money Bank. The data processing agreement between Money Bank and CaliforniaAI requires CaliforniaAI (as processor) to assist Money Bank to fulfil its obligation (as controller) to respond to rights requests, but does not specifically require CaliforniaAI to provide detailed information on the logic behind the profiling nor its application to individual candidates.
Alice, Frank and James all start employment tribunal proceedings in the UK claiming, respectively sex, race and age discrimination in breach of the UK’s Equality Act. They:
- claim direct and indirect discrimination against Money Bank; and
- sue CaliforniaAI for inducing and/or causing Money Bank to discriminate against them.
Despite CaliforniaAI having no business presence in the UK and despite the process being more complicated, the claimants can bring proceedings against an overseas party in the UK employment tribunal.
Unless the claimants are aware of each other’s cases, in reality, these cases are likely to proceed independently. However, for the purposes of this case study, all three approach the same lawyer who successfully applies for the cases to be joined and heard together.
Alice, Frank and James recognise that, despite their suspicions, they will need more evidence to back up their claims. They, therefore, contact Money Bank and CaliforniaAI asking for disclosure of documents with the data and information relevant to their rejections.
They also write to Money Bank and California AI with data subject access requests (DSARs) making similar requests for data. These requests are made by reason of their rights under UK data protection law over which the employment tribunal has no jurisdiction so is independent of their employment tribunal claims.
Disparate impact data
In order to seek to establish discrimination, each candidate requests data:
- Alice asks Money Bank for documents showing the data on both the total proportion of candidates, and the proportion of successful candidates, who were women. This is needed to establish her claim of indirect sex discrimination.
- Frank asks for the same in respect of the Black, Black British, Caribbean or African ethnic group.
- James asks for the data for both over 60-year-olds and over 50-year-olds.
They also ask CaliforniaAI for the same data from all exercises in which GetBestTalent has been used globally.
Would a tribunal order a disclosure request of this nature? In considering applications for the provision of information or the disclosure of documents or data, an employment tribunal must consider the proportionality of the request. It is more likely to grant applications which require extensive disclosure or significant time or cost to provide the requested information where the sums claimed are significant.
In this case, Money Bank has the information sought about the sex, ethnicity and age of both all candidates and of those who were successful which it records as part of its equality monitoring procedures. Providing it, therefore, would not be burdensome. In other cases, the employer may not have this data. CaliforniaAI has the means to extract the data sought, at least from many of the uses of GetBestTalent. However, it would be a time-consuming and costly exercise to do this.
Both respondents refuse to provide any of the data sought. Money Bank argues that this is merely a fishing exercise as none of the claimants have any evidence to support a discrimination claim. They also argue that the system has been audited for discrimination and, therefore, the clams are vexatious. CaliforniaAI also regards the information sought as a trade secret (of both itself and its clients) and also relies on the time and cost involved in gathering it.
In response the claimants apply to the employment tribunal for an order requiring the respondents to provide the data and information requested.
The tribunal orders Money Bank to provide the claimants with the requested documents. It declines, however, to make the order requested against CaliforniaAI.
In theory, the tribunal has the power to make the requested order against CaliforniaAI. Although, it cannot make the order against an overseas person which is not a party to the litigation, in this case California AI is a party. However, the tribunal regards the request as manifestly disproportionate and gives this request short shrift.
The disparate impact data does not amount to the individuals’ personal data so is not relevant to their DSARs.
Equality data
The claimants also request from Money Bank documents showing the details of: (a) the gender, ethnic and age breakdown of the Bank’s workforce in the UK (as the case may be); (b) the equality training of the managers connected with decision to use the GetBestTalent solution; and (c) any discrimination complaints made against Money Bank in the last five years and the outcome.
Money Bank refuses all requests as it argues that the claim relates to the discriminatory impact of CaliforniaAI’s recruitment solution so that all these other issues are irrelevant. It could provide the information relatively easily but is mindful that the Bank has faced many discrimination claims in recent years and has settled or lost a number so does not want to highlight this.
The tribunal refuses to grant the requests for the equality data as it considers it unnecessary for the claimants to prove their case. The claimants will, however, still be able to point to Money Bank’s failure to provide this information in seeking to draw inferences. The tribunal also refuses the request for details of past complaints (though details of tribunal claims which proceeded to a hearing are available from a public register).
The tribunal does ask Money Bank to provide details of the equality training provided to the relevant managers as it was persuaded that this is relevant to the issues to be decided.
This information does not amount to the individuals’ personal data so is not relevant to their DSARs.
Disclosing the algorithm and audit
The claimants also asked CaliforniaAI to provide it with:
- a copy of the algorithm used in the shortlisting programme;
- the logic and factors used by the algorithm in achieving is output (i.e. explainability information relating to their individual decisions); and
- the results of the discrimination audit.
In this case, CaliforniaAI has the information to explain the decisions, but this is not auto-generated (as it can be with some systems) or provided to Money Bank. Money Bank’s contract with CaliforniaAI does not explicitly require it to provide this information.
CaliforniaAI refuses to provide any of the requested information on the basis that these amount to trade secrets and also that the code would be meaningless to the claimants. The claimants counter that expert witnesses should be able to consider the code as medical experts would where complex medical evidence is relevant to tribunal proceedings.
The tribunal judge is not persuaded by the trade secret argument. If disclosed the code would be in the bundle of documents to which observers from the general public would have access (though couldn’t copy or remove). The tribunal has wide powers to regulate its own procedure and, in theory, could take steps in exceptional cases to limit public access to trade secrets.
However, the tribunal decides not to order disclosure of the code on the grounds of proportionality. It spends more time deliberating over the ‘explainability’ information and the details of the auditing of the system.
Ultimately, it decides not to require disclosure of either. It considers that, in so far as the direct discrimination claims are concerned, it requires more than the assertion by the claimants that they have been directly discriminated against to make the requested order proportionate. If the sums likely to be awarded had been greater, it may well have reached a different decision here. In so far as Alice’s indirect claim is concerned, the explainability information and audit are more likely to be relevant to Money Bank’s defence than Alice’s claim so leaves it Money Bank to decide whether or not to disclose it.
Arguably, UK GDPR requires Money Bank to provide the explainability information in response to the data subject access request, and for Money Bank’s data processing agreement with CaliforniaAI to oblige the American company to provide this. However, both respond to the DSAR refusing to provide this information (this case study does not consider the extent to which they might be justified in doing so under UK GDPR).
What did the data show?
The data provided by Money Bank shows that of the 800 job applicants: 320 were women (40%) and 480 were men (60%); 80 described their ethnicity as Black, Black British, Caribbean or African (10%); and James was the only applicant over the age of 50.
Of the 320 women, only four were successful (20% of total shortlisted) whereas 16 men were shortlisted (80% of shortlisted). Of the 80 applicants from Frank’s ethnic group, three were appointed (15% of successful applicants). Therefore, the data shows that the system had a disparate impact against women but not against Black, Black British, Caribbean or African candidates. There was no data to help James with an indirect discrimination claim.
Number of candidates (total 800) | % of candidates | Number of successful candidates (total 20) | % of successful candidates | |
---|---|---|---|---|
Gender - female candidates | 320 | 40% | 4 | 20% |
Ethnicity – Black, Black British, Caribbean or African candidates | 80 | 10% | 3 | 15% |
Age – candidates over 50 years old | 1 | <1% | 0 | 0% |
After consideration of the data, Frank and James abandon their indirect discrimination claims.
Establishing indirect discrimination
Alice needs to establish:
- a provision, criterion or practice (PCP);
- that the PCP has a disparate impact on women;
- that she is disadvantaged by the application of the PCP; and
- that the PCP is not objectively justifiable.
Alice relies on the AI application used by Money Bank as her PCP.
If the decision to reject her had been ‘explainable’ then, as is the case with most human-decisions, the PCP could also be the actual factor which disadvantaged her.
Putting this into practice, let’s say it could have been established from the explainability information that the algorithm had identified career breaks as a negative factor. Alice has had two such breaks and might, in such circumstances, allege that this was unlawfully indirectly discriminatory. A tribunal may well accept that such a factor disadvantages women without needing data to substantiate this. Money Bank would then need to show either that this had not disadvantaged Alice or that such a factor was objectively justifiable.
Neither defence would be easy in this case. It is possible that the respondents could run on a counterfactual to show that Alice had not been disadvantaged by her career breaks. This would mean applying the application to an alternative set of facts – so here, running it against Alice’s application but without career breaks to show she would not have been shortlisted in any event.
In our case, however, Money Bank does not have an explanation for Alice’s failure to be shortlisted.
2. Disparate impact
Alice relies on the data to show a disparate impact.
The respondents could seek to argue that there is no disparate impact based on the auditing undertaken by CaliforniaAI using larger numbers of aggregate audits and argue that the Money Bank’s data reflects a random outcome. A tribunal is certain not to accept this argument at face value. Further, the legal tests in the UK and the US are not the same so any auditing in the US will be of reduced value.
In such a case, the respondents could seek to introduce data from its verification testing or the use of the platform by other employers. This may then require expert evidence on the conclusions to be drawn from the audit data.
In our case, neither the audit nor evidence of the impact of GetBestTalent on larger numbers are before the tribunal. Indeed, here, California AI refused to disclose it.
3. Disadvantages protected group and claimant
Alice does not have to prove why a PCP disadvantages a particular group. The Supreme Court in Essop v Home Office (2017) considered a case where black candidates had a lower pass rate than other candidates under a skills assessment test. The claimants were unable to explain why the test disadvantaged that group, but this was not a bar to establishing indirect discrimination.
The PCP (the GetBestTalent solution) clearly disadvantages Alice personally as her score was the reason she was not shortlisted.
4. Justification
Alice satisfies the first three steps in proving her case.
The burden will then pass to Money Bank to show that the use of this particular application was justified – that it was a proportionate means of achieving a legitimate aim.
What aims could Money Bank rely on? Money Bank argues that its legitimate aim is decision-making which is quicker, cheaper, results in better candidates and discriminates less than with human-made decisions.
Saving money is tricky: it cannot be a justification to discriminate to save money, but this can be relevant alongside other aims. Nonetheless, Money Bank is likely to establish a legitimate aim for the introduction of automation in its recruitment process based on the need to make better and quicker decisions and avoid sub-conscious bias. The greater challenge will be showing that the use of this particular solution was a proportionate means of achieving those aims.
In terms of the objective of recruiting better candidates, Money Bank would have to do more than merely assert that the use of GetBestTalent meant higher quality short-listed candidates. It might, for example, point to historic problems with the quality of successful candidates. This would help justify automation, but Money Bank would still have to justify the use of this particular system.
Money Bank seeks to justify its use of GetBestTalent and satisfy its proportionality by relying on its due diligence. However, it did no more than ask the question of CaliforniaAI who reassured Money Bank that it had been audited.
It also points to the human oversight where a HR professional reviews all candidates which the system proposes to shortlist to verify this decision. The tribunal is unimpressed with the human oversight as this did not extend to oversight of all the unsuccessful applications.
Pulling this together, would a tribunal accept the use of this platform satisfied the objective justification test? This is unlikely. In all likelihood, Alice would succeed, and the matter would proceed to a remedies hearing to determine her compensation.
Establishing direct discrimination
Alice is also pursuing a direct sex discrimination claim and Frank and James, not deterred by the failure to get their indirect discrimination claims off the ground, have also continued their direct race and age discrimination claims respectively. The advantage for Alice in pursuing a direct discrimination claim is that this discrimination (unlike indirect discrimination) cannot be justified, and the fact of direct discrimination is enough to win her case.
Each applicant has to show that they were treated less favourably (i.e. not shortlisted) because of their protected characteristic (sex, race, age respectively). To do this, the reason for the decision not to shortlist must be established.
They have no evidence of the reason, but this does not necessarily defeat their claims. Under UK equality law, the burden of proof can, in some circumstances, transfer so that it is for the employer to prove that it did not discriminate. To prove this, the employer would then have to establish the reason and show that it was not the protected characteristic of the claimant in question. In this case, this would be very difficult for Money Bank as it does not know why the candidates were not shortlisted.
What is required for the burden of proof to transfer? The burden of proof will transfer if there are facts from which the court could decide that discrimination occurred. This is generally paraphrased as the drawing of inferences of discrimination from the facts. If inferences can be drawn, the employer will need to show that there was not discrimination.
Prospects of success
Looking at each claimant in turn:
- Frank will struggle to draw inferences as there is no disparate impact from any less favourable treatment may be inferred. The absence of any disparate impact does not mean that Frank could not have been directly discriminated against but without more his claim in unlikely to get anywhere. He does not have an explanation for the basis of the decision or the ethnic breakdown of its current workforce. He has limited information about Money Bank’s approach to equality. He can’t prove facts which in the absence of an explanation show prima facie discrimination so his claim fails.
- James ‘s claim is unlikely to be rejected as quickly as Frank’s as the data doesn’t help prove or disprove his claim. James could try to rely on the absence of older workers in the workforce, any lack of training or monitoring and past claims if he had this information as well as the absence of an explanation for his rejection but, in reality, this claim looks pretty hopeless.
- Alice may be on stronger ground. She can point to the disparate impact data as a ground for inferences but this will not normally be enough on its own to shift the burden of proof. Alice can also point to the opaque decision-making. Money Bank could rebut this if the decision was sufficiently ‘explainable’ so the reason for Alice’s rejection could be identified. However, it cannot do so here. The dangers of inexplicable decisions are obvious.
Would the disparate impact and opaqueness be enough to draw inferences? Probably not – particularly if Alice does not have any of the equality data or information about past discrimination claims referred to above and the equality training information does not show a total disregard for equality. She could try and get information about these on cross-examination of witnesses and could point to MoneyBank’s failure to provide the equality data as grounds for drawing inferences and reversing the burden of proof. However, after carefully balancing the arguments, the tribunal decides in our case that Alice can’t prove facts which, in the absence of an explanation, show prima facie discrimination. This means that her direct discrimination claim fails.
If inferences had been drawn and Money Bank had been required to demonstrate that the protected characteristic in question had not been the reason for its decision, Money Bank would have argued that it anonymises the candidate data and ensures age, sex and ethnicity of candidates is omitted and that, therefore, the protected characteristic could not have informed the decision. However, as studies have shown how difficult it is to suppress this, the tribunal would give this argument short shrift. If inferences had been drawn, Alice would have, in all likelihood, succeeded with her direct discrimination claim as well as her indirect discrimination claim.
Causing or inducing discrimination
If Money Bank is liable then CaliforniaAI is also likely to be liable for causing/inducing this unlawful discrimination by supplying the system on which Money Bank based its decision. CaliforniaAI cannot be liable if Money Bank is not liable.
The case of Alice, Frank and James highlights the real challenges for claimants winning discrimination claims where AI solutions have been used in employment decision-making. The case also illustrates the risks and pitfalls for employers using such solutions. It illustrates how both existing data protection and equality laws are unsuited for regulating automated employment decisions.
Looking forward, as the UK and other countries are debating over the appropriate level of regulation over AI in areas such as employment, it is to be hoped that these regulations recognise and embrace the inevitably of increased automation but, at the same time, ensure that individuals’ rights are protected effectively.
Is employment law ready for AI?
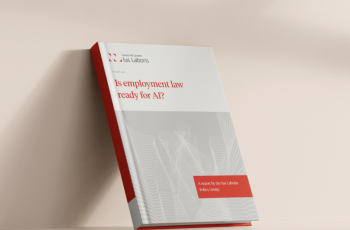
Related Insights
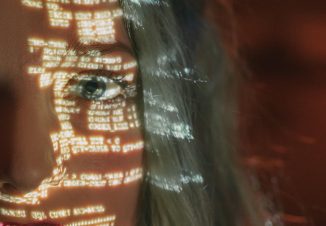
Judge halts further student loan forgiveness under part of Biden's new repayment plan
A federal judge on Monday temporarily barred the Education Department from allowing additional loan forgiveness under a key component of President Joe Biden 's student debt relief plan .
The judge's order halted the cancellation of federal student loans under the income-driven repayment plan known as SAVE after several states sued over the program.
The Biden administration is "preliminarily enjoined from any further loan forgiveness for borrowers under the Final Rule’s SAVE plan until such time as this Court can decide the case on the merits," U.S. District Judge John A. Ross wrote.
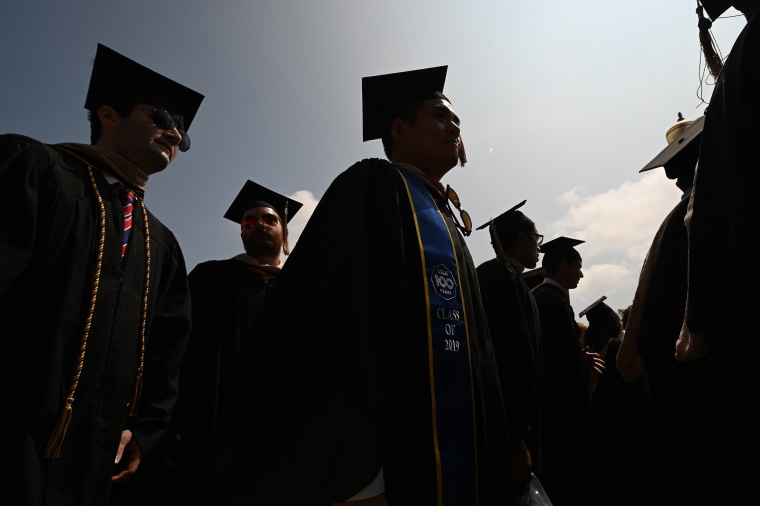
The SAVE plan came about after the Supreme Court last year rejected the Biden administration’s broader effort to address debt under its pandemic-era relief plan , which aimed to erase up to $20,000 in federal student debt for about 43 million borrowers.
Ross also rejected an effort by the Biden administration to dismiss the case, saying the states that brought the lawsuit had standing and "are likely to succeed on the merits of their argument that the early loan forgiveness provisions ... were promulgated in a manner exceeding the Secretary’s statutory authority," referring to the secretary of education.
Missouri Attorney General Andrew Bailey, representing one of the seven states that brought the lawsuit, lauded the ruling.
“By attempting to saddle working Missourians with Ivy League debt, Joe Biden is undermining our constitutional structure,” Bailey said in a statement. “Only Congress has the power of the purse, not the President. Today’s ruling was a huge win for the rule of law, and for every American who Joe Biden was about to force to pay off someone else’s debt.”
Arkansas Attorney General Tim Griffin said: "With Independence Day fast approaching, another court has reminded President Biden that he is not a king. He can’t go around Congress and unilaterally cancel student loans. He should have learned that from Schoolhouse Rock!"
Kansas Attorney General Kris Kobach echoed his counterparts, saying in statement that the loan forgiveness plan is "not only unconstitutional, it’s unfair. Blue collar Kansas workers who didn’t go to college shouldn’t have to pay off the student loans of New Yorkers with gender studies degrees.”
The other states involved in the lawsuit are Florida, Georgia, North Dakota, Ohio and Oklahoma.
In their lawsuit, the states had asked the judge to declare that the program violated the separation of powers under the Constitution.
Ross said other aspects of the program, such as lowering monthly payments and limiting interest accrual, are benefiting borrowers and can continue, adding that the states that sued "have not shown that these provisions harm them."
In another blow to the administration's student debt relief plans, a federal judge blocked the Biden administration Monday from implementing parts of the program that are set to go into effect July 1.
U.S. District Judge Daniel D. Crabtree found that while Kansas and 10 other states that sued over the SAVE plan had not demonstrated irreparable harm from provisions already in effect, they had shown irreparable harm from forthcoming provisions. He granted their request for a preliminary injunction of aspects that have not yet been implemented. His order is set to go into effect Sunday night, allowing time for any appeals.
The 11 states had argued in their March lawsuit that the program violated the Constitution and the Administrative Procedures Act and that many of them suffered losses of state tax revenue because of the plan's provisions about student loan forgiveness.
White House press secretary Karine Jean-Pierre blasted the court rulings on Monday.
"We strongly disagree with today’s rulings on our SAVE Plan and the Department of Justice will continue to vigorously defend the SAVE Plan," she said in a statement. "It’s unfortunate that Republican elected officials and their allies have fought tooth and nail to prevent their constituents from accessing lower payments and a faster path to debt forgiveness — and that courts are now rejecting authority that the Department has applied repeatedly for decades to improve income-driven repayment plans."
In a separate statement, Education Secretary Miguel Cardona added that his department "has relied on the authority under the Higher Education Act three times over the last 30 years to implement income-driven repayment plans."
The Justice Department declined to comment on the rulings.
The Biden administration has forgiv e n significant amounts under the SAVE plan. Last month officials announced the approval of an additional $613 million in debt to be canceled for more than 54,000 borrowers under the plan.
The administration said last month that overall, 4.75 million people have benefited from debt cancellation actions and that it had approved $167 billion in loan forgiveness. The figures pertained to all administrative actions, not just the SAVE plan.
Zoë Richards is the evening politics reporter for NBC News.
Social Acceptance and Educational Aspects of Third Genders: A Case Study of Abbottabad City, Pakistan
Description.
Recognizing and accepting the third gender in educational settings is paramount for fostering inclusivity and social justice. It ensures that all individuals, regardless of their gender identity, have equal access to education and are protected from discrimination and stigma. By integrating the third gender into curricula and educational resources, schools can educate students and staff about gender diversity, promoting empathy and understanding. This acceptance also contributes to broader societal attitudes and legal frameworks that protect the rights of individuals of the third gender in areas beyond education. Ultimately, embracing the third gender in education not only enriches the learning environment by embracing diversity but also nurtures personal growth and empowerment among all students and educators involved.
Institutions
Breaking News
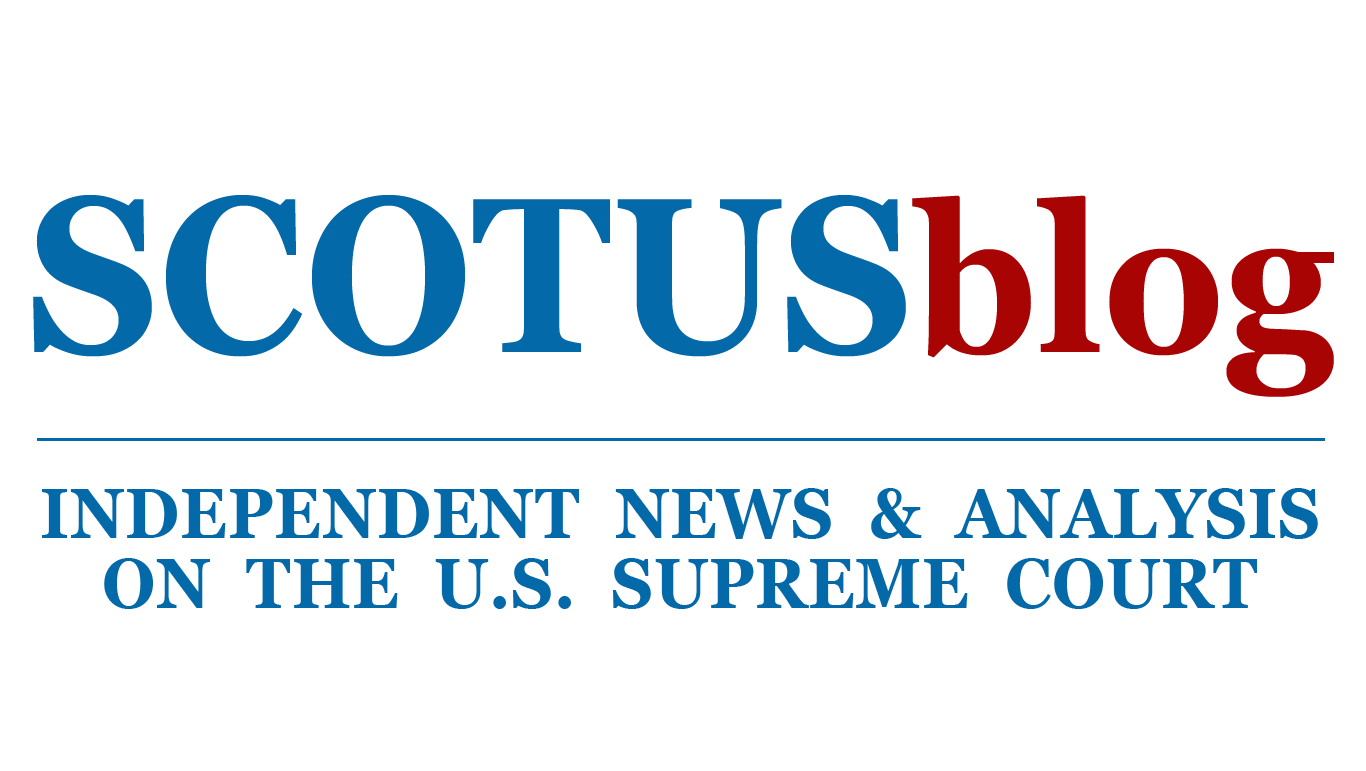
SCOTUS NEWS
Court adds seven cases to next term’s docket.
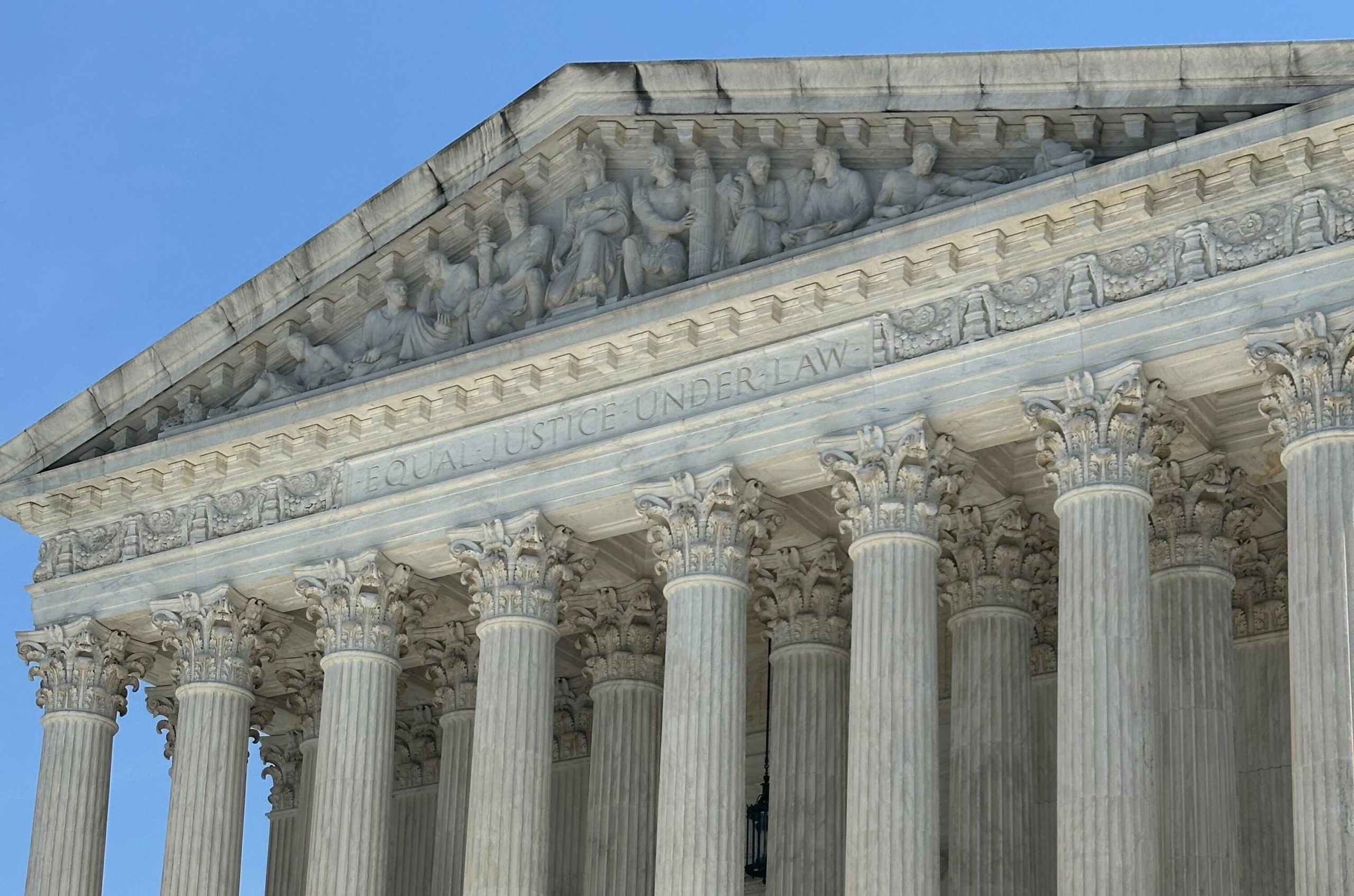
The Supreme Court on Monday added seven new cases to its merits docket for the 2024-25 term, including a challenge to Tennessee’s ban on gender-affirming care for minors. (That case is covered in a separate story .) In a list of orders from the justices’ private conference on June 20, the court agreed to take up a wide variety of issues, ranging from the interpretation of the Foreign Sovereign Immunities Act to compensation for civilians called up for active-duty military service.
Just a few weeks after the 80th anniversary of the D-Day landings, the Supreme Court agreed to take up a case by survivors of the Hungarian Holocaust seeking compensation for the seizure of their property by the Hungarian government.
The issue at the center of Republic of Hungary v. Simon is the interpretation of the Foreign Sovereign Immunities Act, which generally bars lawsuits against foreign governments in U.S. courts but carves out an exception – known as the “expropriation exception” – when (among other things) the lawsuit involves property taken in violation of international law and there is a commercial connection between that property and the United States.
The plaintiffs allege that the Hungarian government took their property, sold it, put the proceeds from the sales in the general state treasury, and then later used funds from the treasury in connection with the country’s commercial activities in the United States.
A federal appeals court in Washington, D.C., agreed that these allegations were enough unless the Hungarian government can show otherwise, while a federal appeals court in New York ruled that the plaintiffs needed to show a connection between the funds from the expropriated property and the commercial activity in the United States. On Monday, the justices agreed to weigh in.
In United States v. Miller , the justices agreed to decide a complicated bankruptcy question that arose after All Resort Group, a Utah transportation firm, filed for bankruptcy in 2017. The bankruptcy trustee attempted to reverse a 2014 payment that the company had made of approximately $145,000 to the Internal Revenue Service to cover the personal tax debts of two company officials. It relied on state law that allows four years to recover fraudulent transfers.
Federal bankruptcy law allows bankruptcy trustees to reverse transfers that a bankruptcy debtor made before filing for bankruptcy if they would be invalid “under applicable law” outside bankruptcy by one of the debtor’s actual creditors. In ARG’s case, the trustee pointed to a debt owed to a former employee, who had sued the company for discrimination and won. But the federal government contended that sovereign immunity would have barred the former employee from suing it to recover the tax payments that ALG had made to the IRS. The question that the justices agreed to take up on Monday is whether a bankruptcy trustee can reverse a debtor’s tax payment to the United States when no actual creditor could have obtained relief.
In Feliciano v. Department of Transportation , the justices agreed to weigh in on whether a federal civilian employee who is called to active military duty during a national emergency is entitled to receive differential pay – compensation for the difference between his civilian pay and his military pay – even if his duty is not directly connected to that national emergency. The question comes to the court in the case of Nick Feliciano, an air traffic controller who was called to active duty in the Coast Guard, where he manned a vessel in and around the Charleston, S.C., harbor.
In Stanley v. City of Sanford, Fla. , the justices will consider a question arising under the Americans with Disabilities Act: Does a former employee lose her right to sue over discrimination in the provision of benefits that she earned while she was employed? The question comes to the court in the case of Karyn Stanley, who worked as a firefighter for the city of Sanford, Fla., for more than two decades before Parkinson’s disease forced her to retire. She filed a lawsuit under the ADA alleging that the city’s benefits policy discriminated against disabled retirees, but the U.S. Court of Appeals for the 11th Circuit ruled that she was not a “qualified individual” protected by the ADA because she was not currently employed by the city.
In Seven County Infrastructure v. Eagle County, Colo. , the court agreed to decide whether the National Environmental Policy Act, which requires federal agencies to examine the environmental effects of proposed actions by the federal government and inform the public of the environmental issues that it considered when making its decisions, requires an agency to study environmental impacts beyond the immediate effects of the action that the agency has authority to regulate. The question comes to the court in a challenge to the scope of an environmental review by the Surface Transportation Board of a new rail line in Utah.
And in Dewberry Group v. Dewberry Engineers , the justices will consider whether, when a plaintiff obtains an award of the “defendant’s profits” in a lawsuit brought under the Lanham Act for a trademark violation, that award can include an order for the defendant to turn over the profits by a separate corporate affiliate that is not part of the case.
The justices declined to hear the case of James Broadnax , a Black man who was convicted and sentenced to death for the robbery and shooting death of two men, Stephen Swan and Matthew Butler, both of whom were white. At his trial in Dallas, prosecutors used almost half of their peremptory strikes – for which no explanation is required – to remove all seven of the potential Black jurors from the jury pool. (The judge later returned one of the Black jurors to the jury.)
In 2016, the Dallas County District Attorney’s Office disclosed a spreadsheet that its prosecutors had used during jury selection to track the race of potential jurors – and in particular, to highlight all of the prospective Black jurors. Five years later, the office disclosed handwritten notes from jury selection about the one of the Black jurors – mentioning that that the prosecutors’ “[o]nly concern” was the parallel between Broadnax’s age and race and that of the juror’s son.
After the Texas state courts rejected Broadnax’s efforts to obtain post-conviction relief, Broadnax came to the Supreme Court, asking the justices to take up his case. Arguing that [r]eview is necessary here because the newly disclosed evidence establishes that a DA’s office with a long and notorious history of racially discriminatory jury selection continued to flout this Court’s direction,” he argued that the new evidence establishes “multiple” violations of the Supreme Court’s 1986 landmark decision in Batson v. Kentucky , holding that prosecutors cannot use their peremptory challenges to exclude potential jurors based on their race.
In a brief unsigned order and without any explanation, the justices turned down Broadnax’s request. Justices Sonia Sotomayor and Ketanji Brown Jackson indicated that they would have reversed the state court’s decision.
The addition of seven new cases to the court’s docket brings the total number of cases granted for next term to 23. The cases granted on Monday’s order list will be argued in the fall, with a decision to follow sometime next year.
This article was originally published at Howe on the Court .
Posted in Capital cases , Merits Cases , Cases in the Pipeline
Cases: Feliciano v. Department of Transportation , Republic of Hungary v. Simon , Dewberry Group, Inc. v. Dewberry Engineers, Inc. , Seven County Infrastructure Coalition v. Eagle County, Colorado , Stanley v. City of Sanford, Florida , Broadnax v. Texas , United States v. Skrmetti , United States v. Miller
Recommended Citation: Amy Howe, Court adds seven cases to next term’s docket , SCOTUSblog (Jun. 24, 2024, 10:29 AM), https://www.scotusblog.com/2024/06/court-adds-seven-cases-to-next-terms-docket/
Privacy Overview
Opinion: As conservatives target same-sex marriage, its power is only getting clearer
- Copy Link URL Copied!
It’s been two years since the U.S. Supreme Court ruling in the Dobbs case that overturned the federal right to an abortion, and the troubling concurring opinion by Justice Clarence Thomas in which he expressed a desire to “revisit” other landmark precedents, including the freedom to marry for same-sex couples, codified nationally by the Obergefell Supreme Court decision, nine years ago Wednesday
Since that ruling, the LGBTQ+ and allied community has done much to protect the fundamental freedom to marry — passing the Respect for Marriage Act in Congress in 2022; sharing their stories this year to mark the 20th anniversary of the first state legalization of same-sex marriages, in Massachusetts; and in California , Hawaii and Colorado launching ballot campaigns to repeal dormant but still-on-the-books anti-marriage constitutional amendments.
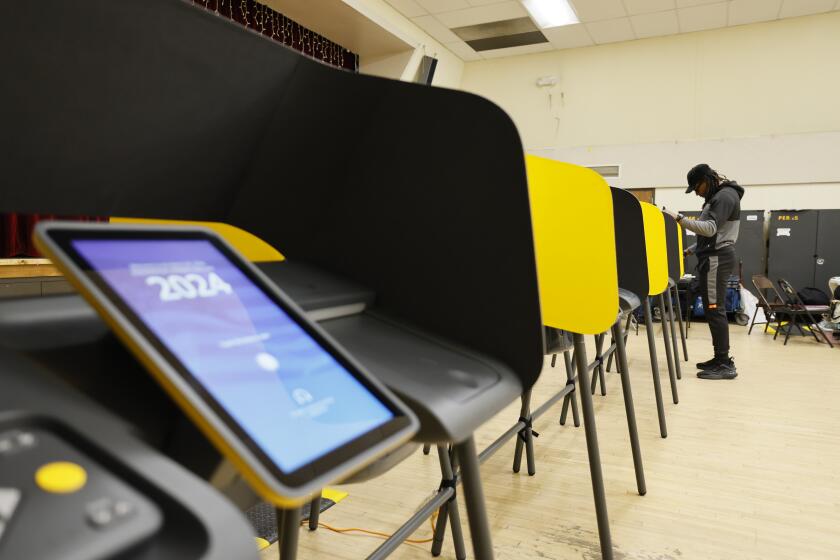
California Democratic Party endorses ballot measures on same-sex marriage, taxes, rent control
The party’s executive board voted Sunday on which measures they would endorse.
May 19, 2024
This winter, I worked with a team at the Williams Institute at UCLA School of Law to survey nearly 500 married LGBTQ+ people about their relationships. Respondents included couples from every state in the country; on average they had been together for more than 16 years and married for more than nine years. Sixty-two percent married after the court’s 2015 Obergefell marriage decision, although their relationships started before before that. More than 30% of the couples had children and another 25% wanted children in the future.
One finding that jumped out of the data: Almost 80% of married same-sex couples surveyed said they were “very” or “somewhat” concerned about the Obergefell decision being overturned. Around a quarter of them said they’d taken action to shore up their family’s legal protections — pursuing a second-parent adoption, having children earlier than originally planned or marrying on a faster-than-expected timeline — because of concerns about marriage equality being challenged. One respondent said, “We got engaged the day that the Supreme Court ruled on the Dobbs decision and got married one week after.”
World & Nation
Same-sex marriage ruling creates new constitutional liberty
The Supreme Court’s historic ruling Friday granting gays and lesbians an equal right to marry nationwide puts an exclamation point on a profound shift in law and public attitudes, and creates the most significant and controversial new constitutional liberty in more than a generation.
June 26, 2015
As we examined the survey results, it became clearer than ever why LGBTQ+ families and same-sex couples are fighting so hard to protect marriage access — and the answer is really quite simple: The freedom to marry has been transformative for them. It has not only granted them hundreds of additional rights and responsibilities, but it has also strengthened their bonds in very real ways.
Nearly every person surveyed (93%) said they married for love; three-quarters added that they married for companionship or legal protections. When asked how marriage changed their lives, 83% reported positive changes in their sense of safety and security, and 75% reported positive changes in terms of life satisfaction. “I feel secure in our relationship in a way I never thought would be possible,” one participant told us. “I love being married.”
The evolution of same-sex marriage
I’ve been studying LGBTQ+ people and families for my entire career — and even still, many of the findings of the survey touched and inspired me.
Individual respondents talked about the ways that marriage expanded their personal family networks, granting them (for better and worse!) an additional set of parents, siblings and loved ones. More than 40% relied on each other’s families of origin in times of financial or healthcare crisis, or to help out with childcare. Some told of in-laws who provided financial assistance to buy a house, or cared for them while they were undergoing chemotherapy for cancer.
Analysis:: Antonin Scalia’s dissent in same-sex marriage ruling even more scornful than usual
The legal world may have become inured to wildly rhetorical opinions by Justice Antonin Scalia, but his dissent in the Supreme Court’s same-sex marriage decision Friday reaches new heights for its expression of utter contempt for the majority of his colleagues.
And then there was the effect on children. Many respondents explained that their marriage has provided security for their children, and dignity and respect for the family unit. Marriage enabled parents to share child-rearing responsibilities — to take turns being the primary earner (and carrying the health insurance), and spending more time at home with the kids.
The big takeaway from this study is that same-sex couples have a lot on the line when it comes to the freedom to marry — and they’re going to do everything possible to ensure that future political shifts don’t interfere with their lives. As couples across the country continue to speak out, share their stories — and in California, head to the ballot box in November to protect their hard-earned freedoms — it’s clear to me that it’s because they believe wholeheartedly, and with good reason, that their lives depend on it.
Abbie E. Goldberg is an affiliated scholar at the Williams Institute at UCLA School of Law and a psychology professor at Clark University, where she directs the women’s and gender studies.
More to Read
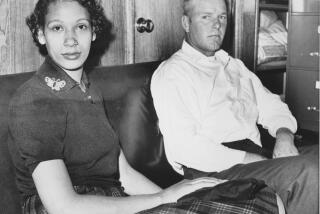
Opinion: Interracial marriage went from criminal to commonplace. Could it go back?
June 9, 2024
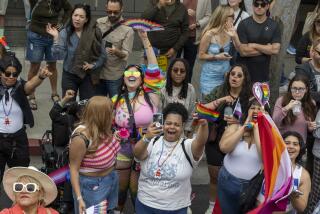
Newsom urges California voters to protect same-sex marriage amid Supreme Court distrust
June 7, 2024
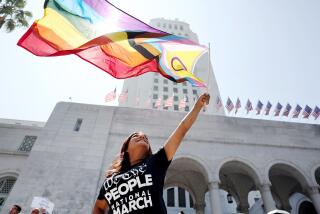
The U.S. has caught up to California on views of LGBTQ+ rights, poll shows
June 6, 2024
A cure for the common opinion
Get thought-provoking perspectives with our weekly newsletter.
You may occasionally receive promotional content from the Los Angeles Times.
More From the Los Angeles Times
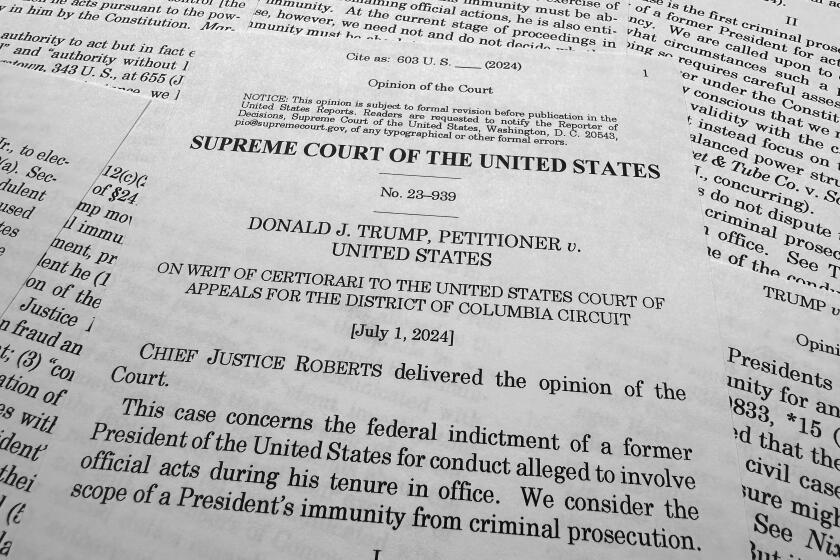
Opinion: We should all dissent from the Supreme Court’s immunity decision, and not respectfully
July 1, 2024
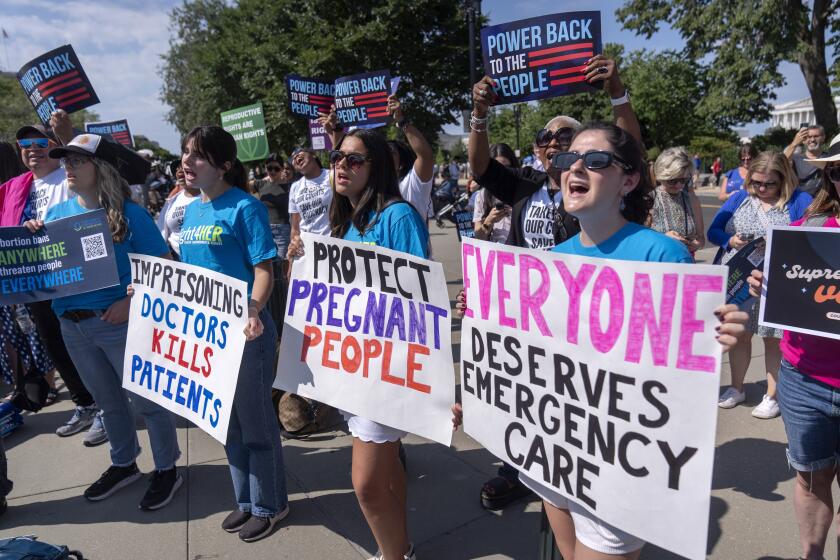
Opinion: If you were relieved by the Supreme Court’s abortion rulings this term, think again
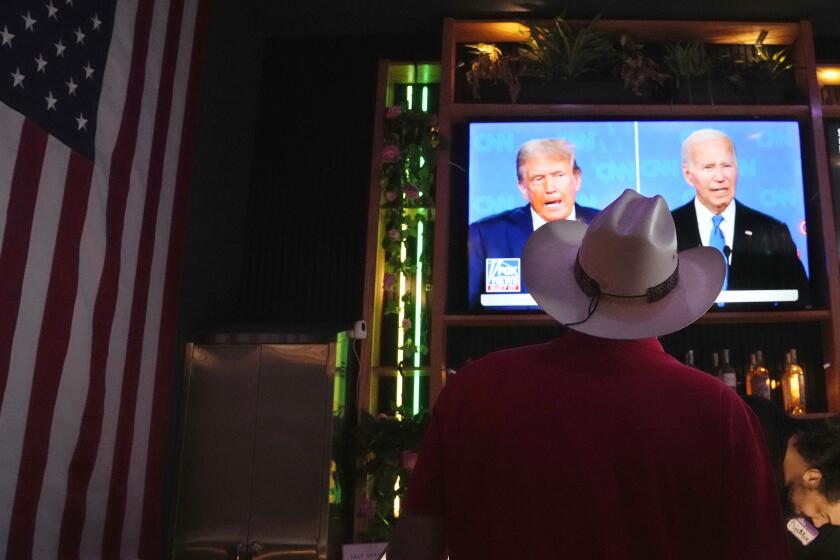
Calmes: The Biden debate and the Supreme Court — the full catastrophe
June 30, 2024
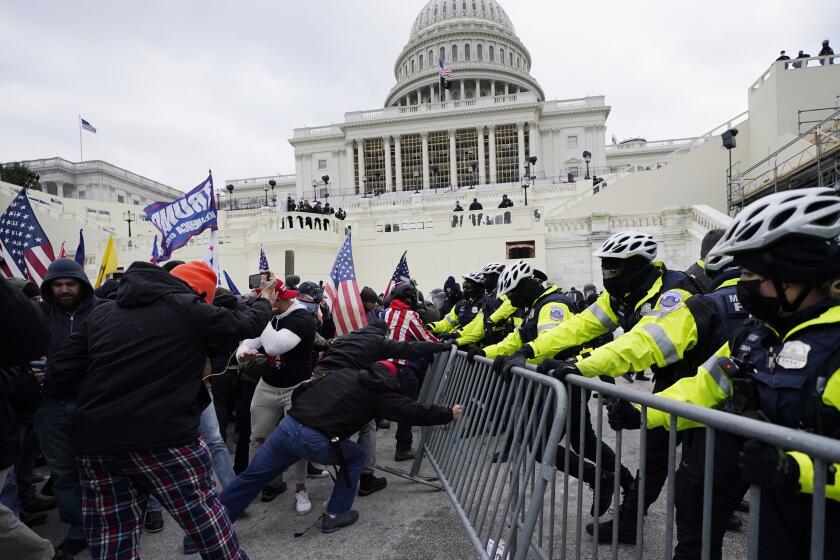
Litman: A Supreme Court ruling may help Jan. 6 rioters. Here’s why it’s less likely to help Trump
June 28, 2024

IMAGES
VIDEO
COMMENTS
Introduction. Women have traditionally been disadvantaged in the labour market, and much scholarship has documented patterns of and trends in gender inequalities (e.g. Weichselbaumer and Winter-Ebmer, 2005; Carlsson, 2011).However, women's and men's working lives have changed considerably since the mid-20th century (Goldin, 2014).In nearly all OECD countries, women now have higher ...
Summary. Progress toward gender equity in certain industries and in leadership roles has been gradual. Next time you make an informal shortlist, particularly for a leadership position or a job in ...
The audit study is designed to measure gender and racial discrimination in hiring screening decisions across three common applicant-job transitions in software engineering: early-career applicants applying laterally to early-career positions (I); the same early-career applicants seeking upward mobility to mid-level positions (II); and mid ...
Inspired by the results of the famous orchestra study — where symphony orchestras began hiring more women by having people audition from behind a screen that concealed their gender — some ...
The overall callback rate was 9.3 per cent 6 (see lowest right-hand corner of Table 1), which is within the standard of previous audit studies (Bertrand and Mullainathan, 2004; Quadlin, 2018) despite the high unemployment rate of the country.As expected, candidates with higher qualifications on their résumés were called in larger numbers than candidates with lower qualifications (11.6 per ...
Foley, M; Cooper, R; and Mosseri S (2019). Gender equitable recruitment and promotion: Leading practice guide, WGEA Commissioned Research Paper, The Australian Women's Working Futures (AWWF) Project, University of Sydney, Sydney, Australia. Gender bias is pervasive at work and in organisations, creating inequalities at every stage of the ...
1 INTRODUCTION. The current study aims to explore gender diversity in recruitment processes, with a particular focus on gender non-normativity. Discrimination against gender and sexual minorities in recruitment processes is a global problem, as measured by both self-reported experiences (FRA, 2014; Grant et al., 2011; Winter et al., 2018) and correspondence studies (Ahmed et al., 2013 ...
This proposed research on algorithmic gender bias in Amazon's recruitment process is important for. promoting diversity and inclusivity in the workplace. Howe ver, the study may encounter messy ...
The aforementioned case of gender bias ... To study the research problem of the negative impacts of algorithmic gender bias in Amazon's recruitment process for prior female IT applicants who may ...
The current research addresses gender trouble (acts that question the naturalness of a binary gender system) in two parts of the recruitment situation: applicant attraction and evaluation.
The case study examines recent aviation safety concerns at Boeing, focusing on manufacturing issues, leadership decisions and regulatory oversight. It traces Boeing's trajectory since the McDonnell Douglas merger in 1997, highlighting the changes in the engineering culture and outsourcing strategy that affected the production quality of the ...
SAN FRANCISCO (Reuters) - Amazon.com Inc's machine-learning specialists uncovered a big problem: their new recruiting engine did not like women. The team had been building computer programs since ...
The present article investigates gender discrimination in recruitment for two male-dominated occupations (mechanics and IT professionals). We empirically test two different explanatory approaches to gender discrimination in hiring; namely, statistical discrimination and taste-based discrimination. Previous studies suggest that, besides job applicants' characteristics, organisational features ...
In the case of the Amazon project, there were a few ways this happened. For example, the tool disadvantaged candidates who went to certain women's colleges presumably not attended by many existing Amazon engineers. It similarly downgraded resumes that included the word "women's" — as in "women's rugby team."
06 September 2022. BHP's first recruitment drive designed to engage with transgender and gender diverse talent has delivered results for the Maintenance and Engineering Centre of Excellence (MECoE) based in Santiago, Chile. With the MECoE having achieved and sustained gender balance for employees since May 2021, the team wanted to widen their ...
This paper explores the extent to which gender bias is introduced in the deployment of automation for hiring practices. We use an interdisciplinary methodology to test our hypotheses: observing a human-led recruitment panel and building an explainable algorithmic prototype from the ground up, to quantify gender bias. The key findings of this study are threefold: identifying potential sources ...
We use an interdisciplinary methodology to test our hypotheses: observing a human-led recruitment panel and building an explainable algorithmic prototype from the ground up, to quantify gender bias. The key findings of this study are threefold: identifying potential sources of human bias from a recruitment panel's ranking of CVs; identifying ...
DOI: 10.1109/ISTAS55053.2022.10227106 Corpus ID: 261331473; Gender Bias in AI Recruitment Systems: A Sociological-and Data Science-based Case Study @article{Njoto2022GenderBI, title={Gender Bias in AI Recruitment Systems: A Sociological-and Data Science-based Case Study}, author={Sheilla Njoto and Marc C. Y. Cheong and Reeva M. Lederman and Aidan James McLoughney and Leah Ruppanner and Anthony ...
Many companies have taken steps to address gender gap issues. In a few cases, such as with tech giant Google, the efforts were found to be lacking. Yet in other cases, changes made have led to ...
Gender Bias Case Study. Despite the progress girls and women have made in school and the workplace in the past few decades, a gender gap still persists, and our research suggests that biases could be at the root of this gap. Gender bias and discrimination is surprisingly common in many schools and sometimes happens beneath school staff's radar.
The hydropower sector is expected to employ 3.7 million people by 2050, emphasizing the potential for more gender-inclusive job opportunities 2 SHP projects around the world have already been instrumental in challenging gender roles and creating opportunities for women in the energy sector, particularly in remote and rural areas.
8. This research identifies the dynamics of gender with four aspects of. employment in civil service, namely: 1) hiring, 2) training, 3) promotion, and 4) termination of service. This study aims ...
Gender equality is not just about fairness and equity; it is also about economic empowerment and economic growth. Estonia has made great strides towards gender equality. Girls today outperform boys in educational attainment, but they are less likely than boys to study mathematics or information and communication technology.
This case study focuses on discrimination claims under the Equality Act 2010. The case study. Acquiring shortlisting tool. Money Bank gets many hundreds of applicants every year for its annual recruitment of 20 financial analysts to be based in its offices in the City of London. Shortlisting takes time and costly HR resources.
By working through a case study about the use of AI in recruitment, we examine the risks of unlawful discrimination and how that might be challenged in a UK employment tribunal. ... The claimants also request from Money Bank documents showing the details of: (a) the gender, ethnic and age breakdown of the Bank's workforce in the UK (as the ...
The U.S. Supreme Court said Monday that it will hear arguments on the constitutionality of state bans on gender-affirming care for transgender minors. The issue has emerged as a big one in the ...
In another blow to the administration's student debt relief plans, a federal judge blocked the Biden administration Monday from implementing parts of the program that are set to go into effect July 1.
Recognizing and accepting the third gender in educational settings is paramount for fostering inclusivity and social justice. It ensures that all individuals, regardless of their gender identity, have equal access to education and are protected from discrimination and stigma. By integrating the third gender into curricula and educational resources, schools can educate students and staff about ...
The Supreme Court on Monday added seven new cases to its merits docket for the 2024-25 term, including a challenge to Tennessee's ban on gender-affirming care for minors. (That case is covered in a separate story.) In a list of orders from the justices' private conference on June 20, the court agree
As we examined the survey results, it became clearer than ever why LGBTQ+ families and same-sex couples are fighting so hard to protect marriage access — and the answer is really quite simple ...